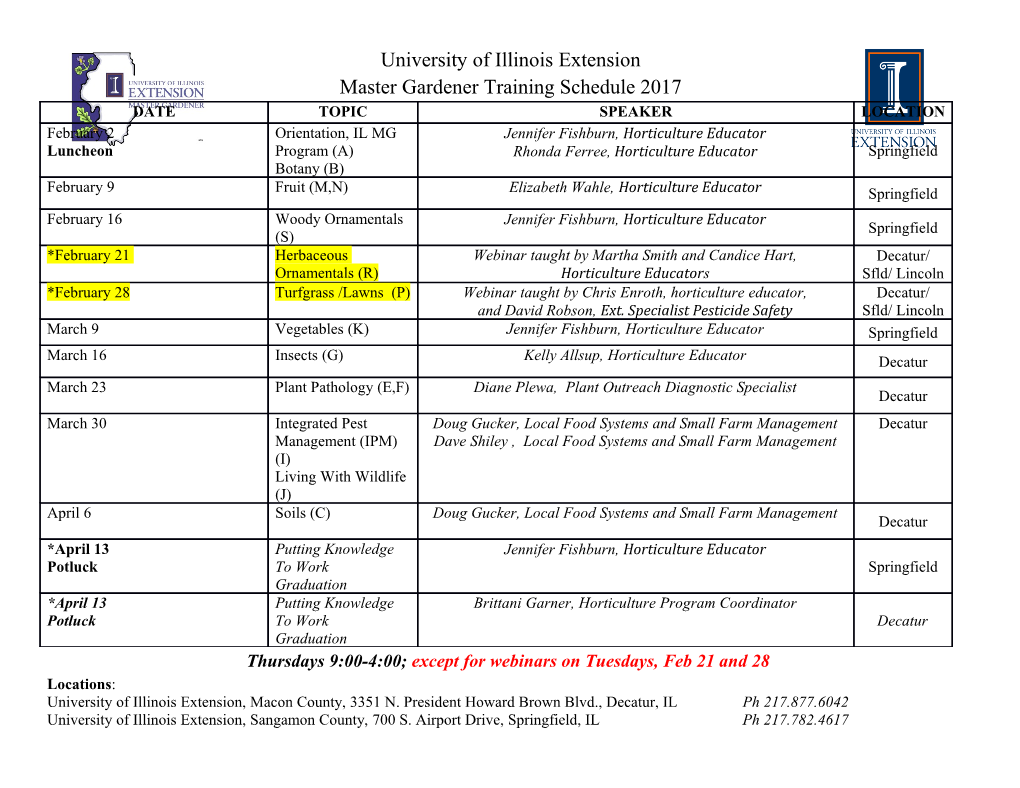
TOWARDS UNBIASED ARTIFICIAL INTELLIGENCE: LITERARY REVIEW OF DEBIASING TECHNIQUES Smulders, C.O., Ghebreab, S. Abstract Historical bias has been feeding into the algorithmic bias inherent in artificial intelligence systems. When considering negative social bias, this process becomes indirectly discriminatory and leads to faulty artificial intelligence systems. This problem has only recently been highlighted as a possible way in which bias can propagate and entrench itself. The current research attempt to work toward a debiasing solution which can be used to correct for negative bias in artificial intelligence. A literary analysis was done on technical debiasing solutions, and actors which effect their implementation. A recommendation for the creation of a debiasing open source platform is made, in which several technical proposed solutions should be implemented. This allows for a low-cost way for companies to use debiasing tools in their application of artificial intelligence systems. Furthermore, development would be sped up, taking proposed solutions out of the highly restricted academic field and into the world of external application. A final note is made on the feasibility of elimination of bias in artificial intelligence, as well as society at large 1: Introduction “Artificial intelligence presents a cultural shift as much as a technical one. This is similar to technological inflection points of the past, such as the introduction of the printing press or the railways” (Nonnecke, Prabhakar, Brown, & Crittenden, 2017). The invention of the printing press was the cause of much social progress: the spread of intellectual property, and the increase in social mobility being just two facets of this breakthrough. It allowed for a shake-up of the vestiges of absolute power derived from medieval society, and reduced age-old economic and social biases (Dittmar, 2011). Conversely, the invention of the steam engine and the industrial revolution that followed, was the cause of great social division: economic domination of the many by the few, the formation of perennial economical groups, and arguably the invention of modern 1 slave labor. While this distinction in not clear cut, it serves as an easy reminder of the possibilities and the danger that a massive paradigm shift in the form of artificial intelligence could bring about (figure 1). The introduction of artificial intelligences into the commercial market (Rockwood, 2018), political systems (Accenture, 2017), legal systems (Furman, Holdren, Munoz, Smith, & Zients, 2016; Nonnecke et al., Figure 1: model of resource and appropriation theory adopted from van Dijk (2012). 2017), warfare (DARPA, 2016), and many Information and Computer Technologies (ICTs) have technological aspects (characteristics), and relational aspects (e.g. participation which affect expanded inequality (i.e. bias). The current other fields is indicative of its future research will focus mainly on the characteristics of artificial intelligence (one possible ICT). widespread usage. Indeed, sources from both commercial (Coval, 2018; Gershgorn, 2018; Nadella, 2018) as well as political institutions (Daley, 2018; Goodman & Flaxman, 2016; STEM-C, 2018) highlight the need for fast and successful implementation of artificial intelligence systems. At a recent keynote address at the World Economic Forum of 2017, the growing AI economic sector, as well as the market efficiency achievable through implementation of AI systems in other sectors, was highlighted as the most important economic change for the coming decades (Bossmann, 2017). Similarly, the European Union (Goodman & Flaxman, 2016), United States (Furman et al., 2016), Great Britain (Daley, 2018), as well as representatives of the G20 (Accenture, 2017), have all mentioned that the use of artificial intelligence and big data in decision-making as key issues to be legislated in the coming decades. The large-scale realization that society is on the brink of an industrial revolution level paradigmatic shift is partly due to the widely discussed book: ”Weapons of Math Destruction” by Cathy O’Neil (O’Neil, 2016). In her book, O’Neil outlines the proliferation of artificial intelligence in many sectors of society, a process that has ramped up significantly after the economic crash of 2008. While Artificial Intelligence is often marketed as efficient, effective, and unbiased, O’Neil criticizes the use of artificial intelligence as markedly unfair, explaining that it propagates existing forms of bias, and drives increasing economic inequality. While she highlights specific cases, such as the racist bias in recidivism models and the economic bias of credit scoring algorithms, the books message revolves around the larger danger that artificial intelligence poses for ingraining existing inequalities. The main message propagated by O’Neil is ‘garbage in, garbage out’, later hyperbolized to: ‘racism in, racism out’. If algorithms are made without regards to the previously existing biased social structures (e.g. financial segregation of minority groups in the US), then those biases will be propagated and enforced. Examples and an explanation of how this phenomenon occurs, both mechanistically and practically, will be expanded upon in the section “Bias in AI”. In the two years following the publishing of “Weapons of Math Destruction” a host of literature, both scientific and newsprint, has been published on this subject (O’Neil, 2017). Many of these articles focus on identifying other segments of society where artificial intelligence is currently causing biased classification (Buranyi, 2017; Knight, 2017), while others focus on potential solutions to the problem (Howard & 2 Borenstein, 2017; Sherman, 2018). Unfortunately, as this field of literature is very young, no synchronicity exists amongst different proposed solutions, nor has much criticism of existing solutions been levied. This leaves a gap in our literary understanding of how different actors can works towards reducing the effects of negative bias in artificial intelligence systems. In a recent article in the New York Times, Kate Crawford, an industry specialist currently working on the issue of ethical artificial intelligence, mentions the urgency of this problem (Crawford, 2018). She compares the issue of biased artificial intelligence to the problem of creating autonomous artificial intelligence noted by tech giants like Elon Musk and Warren Buffet (i.e. the problem of emergence), and notes that these concerns are only relevant if the current problem of biased artificial intelligence can be solved. Indeed, the danger of unquestionable mathematics created under the guise of science resulting in massive disparity is a pressing concern. It is therefore important understand how we can prevent artificial intelligence from learning our worst impulses. The current study will try to fill this research gap by answering the following question: How can we reduce the effects of negative bias in our artificial intelligence systems? This thesis is structured by first elaborating on the definitions of artificial intelligence and bias, creating a clear understanding of the terminology used throughout the paper and delineating the framework of the inquiry. Secondly, an account is made of the processes and mechanisms involved in bias creation in human cognition, artificial intelligence, and at a more conceptual philosophical level. Concrete examples from a variety of disciplines will be explored to elucidate the depth and severity of the problem. Concurrently, proposed solutions will be discussed at multiple stages of the implementation of artificial intelligence: Commercial Political interests interest Artifical intelligence bias Technical Transparency laws implementation Figure 2: model of the four topics of previously proposed solutions which will be discussed in the current paper. It is important to note that all proposed solutions will be discussed as to their effect on, and viability for, any technical implementation of artificial intelligence. 3 technical solutions, market solutions, and political solutions (figure 2). The section of solutions will be concluded by a discussion on solutions of transparency existing in all previously mentioned relevant sectors. Finally, a discussion section will highlight the synchronized proposed solution that resulted from this literary analysis, and a recommendation will be made on how different levels of society (figure 2) can work towards creating more ethically conscious artificial intelligence programming. 2: What is Artificial Intelligence? There is a myriad of examples in popular culture of what Artificial Intelligence can look like, from the 1968 HAL9000 to the eastern Puppetmaster in Ghost in the Shell. Unfortunately, these portrayals often don’t do justice to the wide breadth of technological innovations which fall under the rubric of artificial intelligence. Definitionally, artificial intelligence covers all technology that artificially mimics a certain aspect of cognitive intelligence, be it sensory intelligence, visuospatial intelligence, emotional intelligence, or any other form of intelligence (Gordon-Murnane, 2018). Yet a more generalizable method of describing artificial intelligence would be: an artificially created system that, when given external data, identifies patterns through features to achieve classification of novel stimuli (figure 3, Dietterich & Kong, 1995). As an example, for sensory intelligence, a voice to text translator might be trained on a set of dictated
Details
-
File Typepdf
-
Upload Time-
-
Content LanguagesEnglish
-
Upload UserAnonymous/Not logged-in
-
File Pages28 Page
-
File Size-