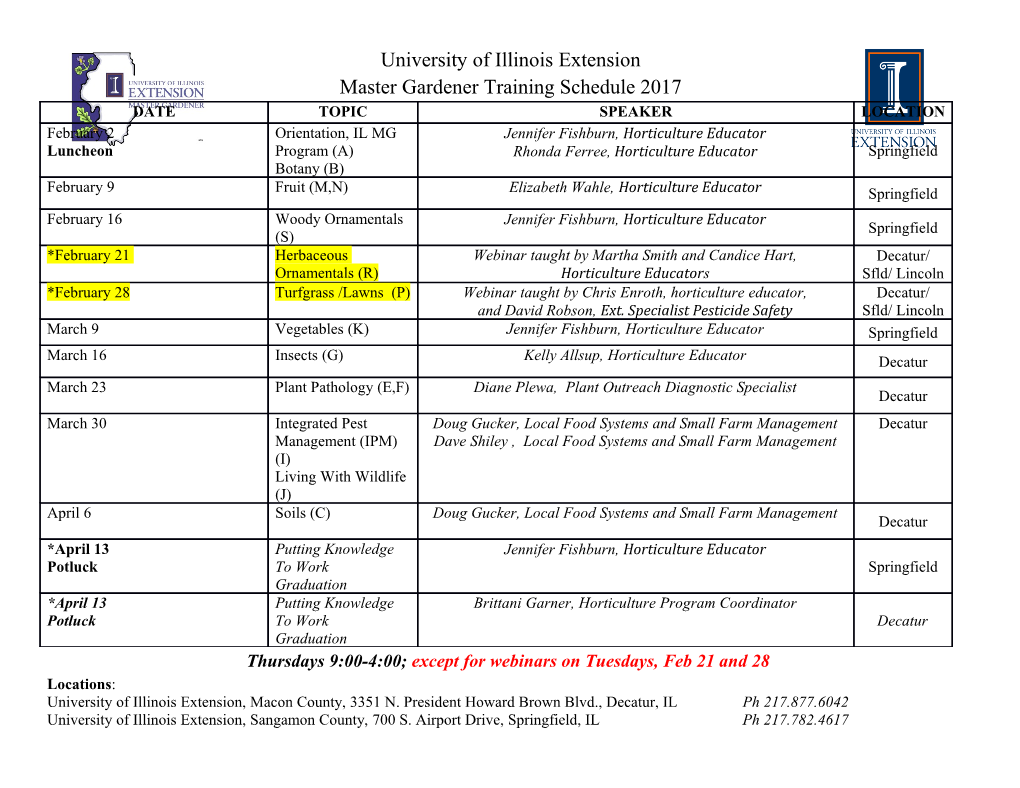
DEGREE PROJECT IN ELECTRICAL ENGINEERING, SECOND CYCLE, 30 CREDITS STOCKHOLM, SWEDEN 2019 Side-Channel Analysis of AES Based on Deep Learning Huanyu Wang KTH ROYAL INSTITUTE OF TECHNOLOGY ELECTRICAL ENGINEERING AND COMPUTER SCIENCE Abstract Side-channel attacks avoid complex analysis of cryptographic algorithms, instead they use side-channel signals captured from a software or a hardware implementation of the algorithm to recover its secret key. Recently, deep learning models, especially Convolutional Neural Networks (CNN), have been shown successful in assisting side-channel analysis. The attacker first trains a CNN model on a large set of power traces captured from a device with a known key. The trained model is then used to recover the unknown key from a few power traces captured from a victim device. However, previous work had three important limitations: (1) little attention is paid to the effects of training and testing on traces captured from different devices; (2) the effect of different power models on the attack’s efficiency has not been thoroughly evaluated; (3) it is believed that, in order to recover all bytes of a key, the CNN model must be trained as many times as the number of bytes in the key. This thesis aims to address these limitations. First, we show that it is easy to overestimate the attack’s efficiency if the CNN model is trained and tested on the same device. Second, we evaluate the effect of two common power models, identity and Hamming weight, on CNN-based side-channel attack’s efficiency. The results show that the identity power model is more effective under the same training conditions. Finally, we show that it is possible to recover all key bytes using the CNN model trained only once. Keywords Side-Channel Attack, Deep Learning, Convolutional Neural Network i Abstract Sidokanalattacker undviker komplex analys av kryptografiska algoritmer, utan använder sig av sidokanalssignaler som tagits från en mjukvara eller en hårdvaruimplementering av algoritmen för att återställa sin hemliga nyckel. Nyligen har djupa inlärningsmodeller, särskilt konvolutionella neurala nätverk (CNN), visats framgångsrika för att bistå sidokanalanalys. Anfallaren tränar först en CNN-modell på en stor uppsättning strömspår som tagits från en enhet med en känd nyckel. Den utbildade modellen används sedan för att återställa den okända nyckeln från några kraftspår som fångats från en offeranordning. Tidigare arbete hade dock tre viktiga begränsningar: (1) Liten uppmärksamhet ägnas åt effekterna av träning och testning på spår som fångats från olika enheter; (2) Effekten av olika kraftmodeller på attackerens effektivitet har inte utvärderats noggrant. (3) man tror att CNN-modellen måste utbildas så många gånger som antalet byte i nyckeln för att återställa alla bitgrupper av en nyckel. Denna avhandling syftar till att hantera dessa begränsningar. Först visar vi att det är lätt att överskatta attackens effektivitet om CNN-modellen är utbildad och testad på samma enhet. För det andra utvärderar vi effekten av två gemensamma kraftmodeller, identitet och Hamming-vikt, på CNN-baserad sidokanalangrepps effektivitet. Resultaten visar att identitetsmaktmodellen är effektivare under samma träningsförhållanden. Slutligen visar vi att det är möjligt att återställa alla nyckelbyte med hjälp av CNN-modellen som utbildats en gång. Nyckelord Side-Channel Attack, Deep Learning, Convolutional Neural Network ii Acknowledgements My study of postgraduate will soon come to an end and at the completion of my graduation thesis, I wish to express my sincere appreciation to all those who have offered me invaluable help during the two years of my postgraduate study here at Royal Institute of Technology. Firstly, I am honored to express my deeply gratitude to my dedicated examiner, Prof. Elena Dubrova and my supervisor, Prof. Mark T Smith, who have offered me valuable suggestions in the academic studies. In the preparation of this thesis, they have spent much time reading through each draft and provided me with inspiring advice. Without their patient instruction, insightful criticism and expert guidance, the completion of this thesis would not have been possible. Secondly, I also owe a special debt of gratitude to my friends Martin Brisfors, Sebastian Forsmark and my opponent Xuwei Gong, who gave me their help and time in listening to me and helping me work out my problems during the difficult course of the thesis. Lastly, I should finally like to express my gratitude to my beloved parents who have always been helping me out of difficulties and supporting without a word of complaint. iii Authors Huanyu Wang <[email protected]> Electrical Engineering and Computer Science KTH Royal Institute of Technology Place for Project Stockholm, Sweden Electrum 229, 164 40 Kista Examiner Prof. Elena Dubrova KTH Royal Institute of Technology Supervisor Prof. Mark T Smith KTH Royal Institute of Technology iv Contents 1 Introduction 1 2 Background 4 2.1 Cryptography Basics and Advanced Encryption Standard . 4 2.2 Side-Channel Attacks .......................... 11 2.3 Deep Learning and Convolutional Neural Networks . 12 3 CNN based Side Channel Analysis 17 3.1 Setup ................................... 17 3.2 Assumptions ............................... 18 3.3 Attack Point and AES Implementation . 18 3.4 Training parameters ........................... 19 3.5 Evaluation ................................ 22 4 Experimental Results 24 4.1 Comparison Between Different Target Boards . 24 4.2 Comparison Between Different Power Models . 26 4.3 Full Key Recovery ............................ 30 5 Conclusion 34 5.1 Future Work ............................... 34 References 35 v 1 Introduction Cryptography is an important part of information security and communication confidentiality. At the present stage, the algorithms, the protocols and the corresponding standards have strictly guaranteed the theoretical security of cryptography. However, for cryptographic systems, one problem that cannot be ignored is that the security in theory is not equivalent to the security in implementation. Because a cryptography algorithm relies on hardware or software implementation, there is a security risk of information leakage when it’s running in a device or a chip. The attacker can observe the side-channel leakage and combine the details of specific cryptographic algorithm for cryptanalysis. The available side-channel information includes execution time [15], power consumption [16], electromagnetic radiation [28], acoustic information [32], cache information [14][24], etc. This type of attacks is called Side-Channel Attacks (SCA). Many well-known cryptography algorithms, including Advanced Encryption Standard (AES) [7], have been broken by the SCA. One powerful tool for that side-channel attacks is Deep Learning (DL). DL helps exploring the correlation between the leakage information and the key. Unlike the traditional side-channel attacks, DL based side-channel attack enables the attacker to use little leakage information (e.g. power traces in power analysis) at the attack stage with a trained DL model. This makes side-channel attack significantly more efficient. Recent works have explored the SCA based on different deep learning techniques, including Multilayer Perception Network (MLP) [21][22][23] and Convolutional Neural Networks (CNN) [3][21]. These works demonstrate that the SCA with properly used deep learning algorithms can perform better than the template attacks [4]. Figure 1.1 shows an overview of how the DL-based SCA works. After training a DL model on a device with a known key, the attacker can apply the trained model to break the target implementation of a cryptographic algorithm with unknown key. 1 Figure 1.1: An overview of how the DL-based SCA works. Specifically, CNNs can be applied against jitter-based countermeasures [3] and masked AES implementation [25]. The further details about deep learning and convolutional neural network can be found in 2.3. The previous CNN-based SCAs have some limitations and based on [2][3][20][26][31], this thesis explores the CNN-based SCA with the following contributions: 1. This thesis explores how the board diversity can affect the performance of the CNN-based side-channel attacks. The results show that it is easy to overestimate the accuracy of the side-channel attack if the CNN models are trained and tested on traces captured from same board. 2. Rare works pay attention to how different the power models affect the CNN- based side-channel attacks. This thesis compares the 9-classifier (Hamming weight power model) and the 256-classifier (identity power model). 3. The previous work [25] claims that, to recover an entire key, the number of times a neural network must be trained is equivalent to the number of bytes in the key. This thesis demonstrates that for CNN-based SCA, it is enough to train a model with one byte of the key to recover an entire key. In our study, the target encryption algorithm is Advanced Encryption Standard 2 (AES), which plays an important role. It is one of the most popular symmetric encryption algorithms at present. See the details of AES in 2.2. In a SCA, different side-channel information requires different type of analysis. In this thesis, analysis is based on power consumption, since it has become a serious security issue for cryptographic devices such as smart cards. 3 2 Background The ability of deep learning to explore relationships in raw data makes it a good candidate for side-channel analysis. In recent years, many studies on side- channel attacks based on deep learning have emerged in order to make the SCA more efficient. Based on the previous works, this thesis aims to explore a more efficient side-channel attack based on CNN. This section introduces the theoretical background of cryptography, side-channel attacks, and machine learning. The review of each respective field will generally include the overview as well as the theoretical descriptions, traditional analytical methods, evaluation criteria and examples. 2.1 Cryptography Basics and Advanced Encryption Standard Side-channel attacks aim at breaking an implementation of the cryptographic algorithms, it is necessary to learn the cryptography basics.
Details
-
File Typepdf
-
Upload Time-
-
Content LanguagesEnglish
-
Upload UserAnonymous/Not logged-in
-
File Pages47 Page
-
File Size-