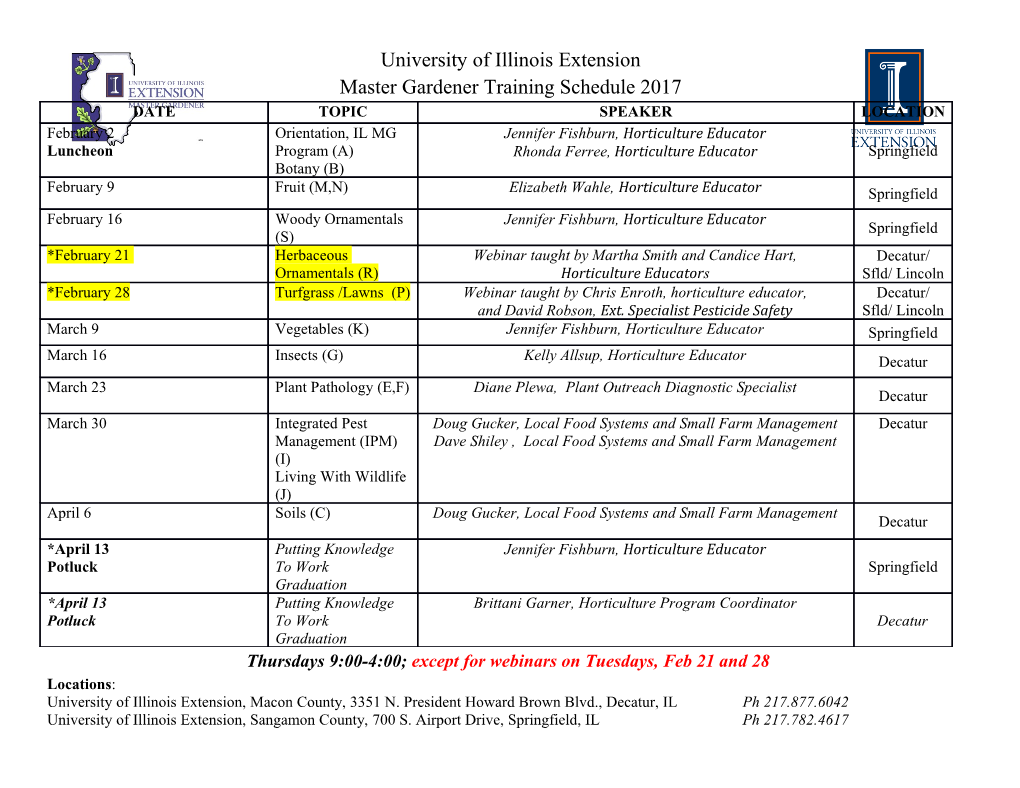
OPERATIONS RESEARCH AND DECISIONS No. 1 2013 DOI: 10.5277/ord130104 Katarzyna OSTASIEWICZ * Edyta MAZUREK* COMPARISON OF THE GINI AND ZENGA INDEXES USING SOME THEORETICAL INCOME DISTRIBUTIONS ABSTRACT The most common measure of inequality used in scientific research is the Gini index. In 2007, Zenga proposed a new index of inequality that has all the appropriate properties of an measure of equality. In this paper, we compared the Gini and Zenga indexes, calculating these quantities for the few distributions frequently used for approximating distributions of income, that is, the lognormal, gamma, inverse Gauss, Weibull and Burr distributions. Within this limited examination, we have ob- served three main differences. First, the Zenga index increases more rapidly for low values of the var- iation and decreases more slowly when the variation approaches intermediate values from above. Se- cond, the Zenga index seems to be better predicted by the variation. Third, although the Zenga index is always higher than the Gini one, the ordering of some pairs of cases may be inverted. Keywords: inequality, Gini index, Zenga index 1. Introduction During the last few decades, inequalities have played an important role in many branches of social science, mainly sociology and economics, being one of the key issues in the discourse regarding the well-being of societies and individuals (see, e.g. [6, 11, 13]). Thus, there appears a question of how to measure these inequalities ap- propriately. There exist many inequality indexes in the literature, e.g. the most popular ones, namely, the Gini index, Theil index and Atkinson measure [11]. Out of these, the Gini index [5] is most frequently used and also best known to non-scientists. Re- cently, a new inequality index was proposed by Zenga [15]. It has all the properties _________________________ *Department of Statistics, Wrocław University of Economics, Komandorska118/120, 53-345 Wro- cław, e-mail addresses: [email protected], [email protected] 38 K. OSTASIEWICZ, E. MAZUREK that are usually required for inequality measures (e.g. the Pigou–Dalton transfer prin- ciple or income scale independence [2, 12]). In order to decide which measure of ine- quality is most suitable for a given subject, it would be helpful to investigate and com- pare the properties of different indexes. The aim of this paper was to compare the values of the Gini and Zenga indexes by applying them to some theoretical distributions. As our main concern will be econom- ic inequalities, we will concentrate on distributions that are commonly used as approx- imations of real income distributions [4, 10], that is, the lognormal, gamma, inverse Gauss, Weibull and Burr distributions. We will present how these inequality indexes depend on skewness and variation. In four cases (lognormal, gamma, inverse Gauss, Weibull distributions) skewness is a unique, monotonic function of the variation, thus the relation between any index and either skewness or variation may be easily recov- ered from the relation between the index and the other. In the case of the Burr distribu- tion, a fixed value of skewness may correspond to various values of the variation and vice versa. Therefore, we should examine how these inequality indexes depend on the variation and skewness separately for this case. In what follows, we will also briefly analyze some examples of the generalized Burr distribution of the second kind and one instance of a bimodal distribution, to draw more conclusions regarding how ine- quality measures depend on the variation and skewness. The paper is organized as follows. In the next section, we briefly introduce the concept of the Zenga index. Section 3 is devoted to the methodology of the research, that is, the quantities and relations used in the subsequent sections have been defined. In sections 4–8 we examine the Zenga index and its relation to the Gini index for five different classes of distributions. Section 9 presents an in depth overview of the com- bined results from sections 4–8, while the final section contains some conclusions and a summary of the paper. 2. The Zenga index of inequality In a series of papers, Zenga (e.g. [7, 9, 14, 15]) proposed a measure of inequality based on the inequality curve I(p), which is defined in terms of the lower and upper arithmetic means of a distribution. This idea of measuring income inequality consists of comparing the arithmetic means of the incomes of two groups, called the lower and upper groups. The division of the ordered data into two groups is made by choosing a point of division. At one extreme, the lower group consists of only the lowest observation. At the other ex- treme, the upper group consists of only the greatest income. Let Comparison of the Gini and Zenga indexes 39 ⎧⎫s ⎨⎬()x jj,:1,,;0nj=≤<<<=…… s xx12 x s ; ∑ nN j ⎩⎭j =1 denote the frequency distribution of a nonnegative random variable X. Next, let us divide this distribution into two parts, the lower and upper group, respectively: ()()x11,,,,,,nxn 2 2… xnjj, xnjj,, x j++11 , n j ,,,… () xn ss . For each point of { ( )} {( )( ) } + division, it is possible to define the lower mean M ()p j and upper mean M ()p j of the divided distribution as follows: 1 j M ()pxnjsjii==∑ ,1,,… (1) N j i=1 + 1 s M ()pxnjsjii==∑ ,1,,… (2) NN− j−1 ij= j N j where: Nnj = ∑ i and p j = . i=1 N + + Comparing M ( p j ) and M ()p j using the index Up()jjj= Mp()/, Mp ()we get a point measure of the uniformity of the distribution. Up( j ) × 100 gives the lower mean as a percentage of the upper mean. The point inequality index is defined in terms of Up( j ) as: Ip( j ) =−1 Up( j ) (3) The synthetic inequality measure proposed by Zenga [15] is the following weighted arithmetic mean of the point measures I( pj): s n j ZIp= ∑ ()j (4) j =1 N Both U( pj) and I( pj) take values between 0 and 1 inclusively. In particular: x1 M x1 M Up()1 = , Up()s = , Ip()1 =−1, Ip()s =−1 M xs M xs 40 K. OSTASIEWICZ, E. MAZUREK where M is the mean of all the observations. The Zenga index takes the value 0 in the case of no inequality. The shape of the Ip( j ) curve as a function of pj is not constrained by fixed points (0, 0) and (1, 1), as in the case of the Lorenz curve [3, 8] (also, see [15] for many illustrative examples). It has been proven that the Zenga index is characterized by all the main properties that any inequality measure should satisfy (see e.g. [2]). 3. Comparison of the Zenga and Gini indexes In what follows, we compare the values of the Gini and Zenga indexes, calculated for some (theoretical) income distributions. These distributions are standard distribu- tions used to simulate distributions of real incomes, that is, the lognormal, Gamma, inverse Gauss, Weibull and Burr distributions. Our aim here is to investigate the dependence of both the Gini and Zenga indexes on the variation and skewness for each family of distributions. For distributions char- acterized by a single shape parameter (i.e. the lognormal, gamma, inverse Gauss and Weibull distributions), the skewness can be obtained from the variation and vice versa and thus there should be a unique function describing each of the inequality indexes on the variation (and skewness), while in the case of distributions with two shape pa- rameters (the Burr distribution here) we can expect, in general, different relations be- tween these inequality indexes and the variation (for constant and different values of skewness) and vice versa. We will then examine the (eventual) differences in how these inequality indexes depend on the variation. We will also examine for each distri- bution the inter-dependence between the Zenga index and the Gini index. We will adopt the following quantities as measures of variation and skewness. To measure variation, we will use the classical coefficient of variation: Var()X V = EX() where Var( X ) denotes the variance and E( X ) is the expected value of the random variable X. We will measure the skewness in terms of the third standardized moment, that is: 3 EX(()− EX()) As = Var()X 3/2 where E(·) again denotes the expected value. Comparison of the Gini and Zenga indexes 41 It is well known (see e.g. [1]) that one of the most essential properties of an income distribution is the existence of only a small number of finite moments (e.g. the Burr distribution). Hence, the parametric space in which our investigations of the inequality indexes are possible is reduced (as the existence of the first moment is necessary to de- fine both the Gini and Zenga indexes). Measuring variation and skewness in terms of the classical measures defined above reduces the parametric space even more. However, as we do not claim to investigate the whole range of the parameter space thoroughly, we will still use these classical quantities to make the results easily interpretable (the orders of magnitude of these parameters for typical income distributions are known). As for calculating the Gini and Zenga indexes, and, correspondingly, the Lorenz curve and I(p) curve, we performed numerical calculations according to the general formulas. For any continuous distribution D with probability density function f ()x , x cumulative probability density function F ()xfydy= ∫ () , and mean value −∞ ∞ myfydy= ∫ () , the Gini index may be calculated using: −∞ 1 ∞ GFxFxdx=−∫ ()()1 () (5) m −∞ As for the Zenga index, the continuous counterpart of (4) reads as follows: ∞ Z = ∫ Ixfxdx()() (6) −∞ where x ∫ yf() y dy −∞ Ix()=−1 ∞ ∫ yf() y dy x 1− F ()x The Lorenz curve is expressed as a function of the cumulative distribution: Fx−1 () 1 LFx()()= ∫ xfxdx() (7) m −∞ 42 K.
Details
-
File Typepdf
-
Upload Time-
-
Content LanguagesEnglish
-
Upload UserAnonymous/Not logged-in
-
File Pages26 Page
-
File Size-