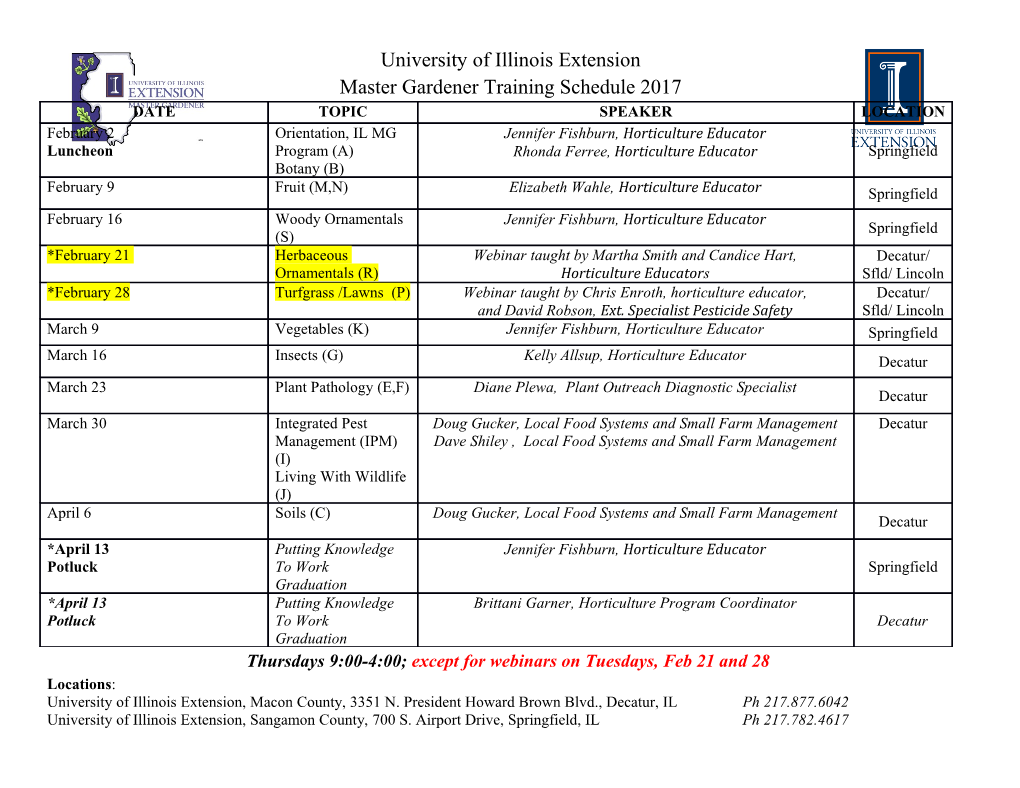
Optimal design of experiments Session 4: Some theory Peter Goos 1 / 40 Optimal design theory Ï continuous or approximate optimal designs Ï implicitly assume an infinitely large number of observations are available Ï is mathematically convenient Ï exact or discrete designs Ï finite number of observations Ï fewer theoretical results 2 / 40 Continuous versus exact designs continuous Ï ½ ¾ x1 x2 ... xh Ï » Æ w1 w2 ... wh Ï x1,x2,...,xh: design points or support points w ,w ,...,w : weights (w 0, P w 1) Ï 1 2 h i ¸ i i Æ Ï h: number of different points exact Ï ½ ¾ x1 x2 ... xh Ï » Æ n1 n2 ... nh Ï n1,n2,...,nh: (integer) numbers of observations at x1,...,xn P n n Ï i i Æ Ï h: number of different points 3 / 40 Information matrix Ï all criteria to select a design are based on information matrix Ï model matrix 2 3 2 T 3 1 1 1 1 1 1 f (x1) ¡ ¡ Å Å Å T 61 1 1 1 1 17 6f (x2)7 6 Å ¡ ¡ Å Å 7 6 T 7 X 61 1 1 1 1 17 6f (x3)7 6 ¡ Å ¡ Å Å 7 6 7 Æ 6 . 7 Æ 6 . 7 4 . 5 4 . 5 T 100000 f (xn) " " " " "2 "2 I x1 x2 x1x2 x1 x2 4 / 40 Information matrix Ï (total) information matrix 1 1 Xn M XT X f(x )fT (x ) 2 2 i i Æ σ Æ σ i 1 Æ Ï per observation information matrix 1 T f(xi)f (xi) σ2 5 / 40 Information matrix industrial example 2 3 11 0 0 0 6 6 6 0 6 0 0 0 07 6 7 1 6 0 0 6 0 0 07 ¡XT X¢ 6 7 2 6 7 σ Æ 6 0 0 0 4 0 07 6 7 4 6 0 0 0 6 45 6 0 0 0 4 6 6 / 40 Information matrix Ï exact designs h X T M ni f(xi)f (xi) Æ i 1 Æ where h = number of different points ni = number of replications of point i Ï continuous designs h X T M wi f(xi)f (xi) Æ i 1 Æ 7 / 40 D-optimality criterion Ï seeks designs that minimize variance-covariance matrix of ¯ˆ ¯ 2 T 1¯ Ï . that minimize ¯σ (X X)¡ ¯ ¯ T 1¯ Ï . that minimize ¯(X X)¡ ¯ ¯ ¯ . that maximize ¯XT X¯ or M Ï j j Ï D-optimal designs minimize ˆ Ï generalized variance of ¯ Ï volume of confidence ellipsoid about unknown ¯ Ï “D” stands for determinant 8 / 40 Ds-optimality criterion Ï useful when the interest is only in a subset of the parameters Ï useful when intercept or block effects are of no interest Ï seeks designs that minimize determinant of variance-covariance matrix corresponding to subset of ¯ Y X¯ ² Ï Æ Å X ¯ X ¯ ² Æ 1 1 Å 2 2 Å where ¯1 is the set of parameters of interest 9 / 40 Ds-optimality criterion · T T ¸ T X1 X1 X1 X2 Ï M (X X) T T Æ Æ X2 X1 X2 X2 · ¸ T 1 A B cov(¯ˆ ) (X X)¡ Ï Æ Æ CD part of the variance-covariance matrix corresponding to ¯1 minimizing A is the same as minimizing Ï j j ¯¡ T T T 1 T ¢ 1¯ ¯ X X X X (X X )¡ X X ¡ ¯ ¯ 1 1 ¡ 1 2 2 2 2 1 ¯ and as maximizing ¯ T T T 1 T ¯ ¯X X X X (X X )¡ X X ¯ 1 1 ¡ 1 2 2 2 2 1 10 / 40 A-optimality criterion Ï seeks designs that minimize average variance of parameter estimates Ï seeks designs that minimize p X ¡ ˆ ¢ var ¯i /p i 1 Æ p = number of model parameters Ï seeks designs that minimize 2 ¡ T ¢ 1 trace σ X X ¡ or ¡ T ¢ 1 trace X X ¡ 11 / 40 V- and G-optimality criteria Ï V-optimality (also Q-, IV-, I-optimality) Ï seeks designs that minimize average prediction variance over design region Â Ï . that minimize Z 2 T T 1 σ f (x)(X X)¡ f(x)dx Â Ï G-optimality Ï seeks designs that minimize maximum prediction variance over design region Â Ï . that minimize T T 1 max var{yˆ(x)} max f (x)(X X)¡ f(x) Â Æ Â 12 / 40 Discussion Ï problem: in general, all optimality criteria lead to different designs Ï exception: D-optimal continuous designs = G-optimal continuous designs Ï general equivalence theorem Ï prediction variance is maximal in design points Ï maximum = number of model parameters Ï what optimality criterion to use? 13 / 40 D-optimality criterion Ï most popular criterion Ï D-optimal designs are usually quite good w.r.t. other criteria Ï D-optimal designs are not affected by linear transformations of levels of the experimental variables Ï computational advantages: update formulas 14 / 40 Linear transformations of factor levels X XA Z ! Æ #¯ ¯ & ¯ ¯ max¯XT X¯ max¯ZT Z¯ ¯ ¯ ¯(XA)T XA¯ Æ ¯ ¯ ¯AT XT XA¯ Æ ¯ ¯¯ ¯ ¯AT ¯¯XT X¯ A Æ j j ¯ ¯ A 2 ¯XT X¯ Æ j j 15 / 40 Quadratic regression in one variable  [ 1,1] Ï Æ ¡ Y ¯ ¯ x ¯ x2 ² Ï Æ 0 Å 1 Å 2 Å Ï D-optimal continuous design ½ 1 0 1 ¾ Design 1 ¡ Å Æ 1/3 1/3 1/3 Ï information matrix 2 1 0 2/33 M1 4 0 2/3 0 5 Æ 2/3 0 2/3 16 / 40 Quadratic regression in one variable Ï general equivalence theorem implies that D-optimal continuous design is also G-optimal Ï D-optimal design minimizes the maximum prediction variance Ï maximum is equal to p Ï check by plotting prediction variance T 1 var{yˆ(x)} f (x)M¡ f(x) Æ 1 for all x [ 1,1] 2 ¡ 17 / 40 Prediction variance T 1 var{yˆ(x)} f (x)M¡ f(x) Æ 1 2 3 0 332 1 3 £ 2¤ 3 ¡ 1 x x 4 0 0 54 x 5 Æ 2 3 0 9 x2 ¡ 2 2 1 3 £ 2 3 9 2¤ 3 3x x 3 x 4 x 5 Æ ¡ 2 ¡ Å 2 x2 3 9 3 3x2 x2 3x2 x4 Æ ¡ Å 2 ¡ Å 2 9 9 3 x2 x4 Æ ¡ 2 Å 2 18 / 40 Prediction variance var{yˆ(x)} p 3 Æ 2 1 1 0.5 0 0.5 1 ¡ ¡ 19 / 40 Quadratic regression in one variable Ï consider other design ½ 1 0 1 ¾ Design 2 ¡ Å Æ 1/4 1/2 1/4 Ï information matrix 2 1 3 1 0 2 1 M2 40 2 05 Æ 1 1 2 0 2 20 / 40 Prediction variance T 1 var{yˆ(x)} f (x)M¡ f(x) Æ 2 2 2 0 232 1 3 £ 2¤ ¡ 1 x x 4 0 2 0 54 x 5 Æ 2 0 4 x2 ¡ 2 1 3 £ 2 2¤ 2 2x 2x 2 4x 4 x 5 Æ ¡ ¡ Å x2 2 2x2 2x2 2x2 4x4 Æ ¡ Å ¡ Å 2 2x2 4x4 Æ ¡ Å 21 / 40 Prediction variance var{yˆ(x)} 4 p 3 Æ 2 1 1 0.5 0 0.5 1 ¡ ¡ + Design 2 is not D-optimal 22 / 40 Optimal exact designs Ï A-optimal continuous design ½ 1 0 1¾ » ¡1 1 1 Æ 4 2 4 what if n 4,8,12? Ï Æ Ï multiply weights of continuous design by 4, 8 or 12 Ï integer numbers of runs at each point what if n 5,6,7? Ï Æ Ï multiply weights by 5, 6, or 7 Ï non-integer numbers of runs at some of the points Ï not useful in practice 23 / 40 Polynomial regression in one variable D-optimal design points for the dth order polynomial regression in one variable d x1 x2 x3 x4 x5 x6 x7 weight 1 1 1 1/2 ¡ 2 1 0 1 1/3 ¡ 3 1 a a 1 1/4 ¡ ¡ 3 3 4 1 a 0 a 1 1/5 ¡ ¡ 4 4 5 1 a b b a 1 1/6 ¡ ¡ 5 ¡ 5 5 5 6 1 a b 0 b a 1 1/7 ¡ ¡ 6 ¡ 6 6 6 q a p1/5 b (7 2p7)/21 3 Æ 5 Æ ¡ q a p3/7 a (15 2p15)/33 4 Æ 6 Æ Å q q a (7 2p7)/21 b (15 2p15)/33 5 Æ Å 6 Æ ¡ 24 / 40 Quadratic regression in one variable Ï Design 1 has covariance matrix 2 3 0 3 3 T 1 ¡ (X X)¡ 4 0 3/2 0 5 Æ 3 0 9/2 ¡ T 1 with trace(X X)¡ 3 3/2 9/2 9 Æ Å Å Æ Ï Design 2 has covariance matrix 2 2 0 23 T 1 ¡ (X X)¡ 4 0 2 0 5 Æ 2 0 4 ¡ T 1 with trace(X X)¡ 2 2 4 8 Æ Å Å Æ Ï Design 2 is A-optimal 25 / 40 23 Factorial design Ï main-effects model Y ¯ ¯ x ¯ x ¯ x ² Æ 0 Å 1 1 Å 2 2 Å 3 3 Å Ï model matrix 21 1 1 13 ¡ ¡ ¡ 61 1 1 17 6 Å ¡ ¡ 7 61 1 1 17 6 ¡ Å ¡ 7 61 1 1 17 X 6 Å Å ¡ 7 Æ 61 1 1 17 6 ¡ Å Å 7 61 1 1 17 6 Å Å Å 7 61 1 1 17 4 ¡ ¡ Å 5 1 1 1 1 Å ¡ Å 26 / 40 23 factorial design Ï (total) information matrix 28 0 0 03 T 60 8 0 07 M X X 6 7 Æ Æ 40 0 8 05 0 0 0 8 Ï per observation information matrix 21 0 0 03 1 T 60 1 0 07 M¤ (X X) 6 7 Æ n Æ 40 0 1 05 0 0 0 1 27 / 40 Prediction variance T © ª 1 var{yˆ(x)} f (x) M¤ ¡ f(x) Ï Æ 2 1 3 £ ¤© ª 1 6x17 1 x1 x2 x3 M¤ ¡ 6 7 Æ 4x25 x3 1 x2 x2 x2 Æ Å 1 Å 2 Å 3 if  [ 1,1]3, then this is maximal when Ï Æ ¡ x 1, x 1, x 1 1 Ƨ 2 Ƨ 3 Ƨ Ï maximum = 4 = p Ï general equivalence theorem is satisfied 3 Ï 2 factorial design is D- and G-optimal 28 / 40 More Ï Plackett-Burman designs: optimal for main-effects model Ï factorial designs: optimal for main-effects-plus-interactions models Ï some remarks Ï off-diagonal elements of information matrix often zero (impossible when quadratic terms) Ï optimal designs are often symmetric Ï these properties are more difficult to achieve for exact designs 29 / 40 Quadratic regression in two variables Y ¯ ¯ x ¯ x ¯ x x ¯ x2 ¯ x2 ² Ï Æ 0 Å 1 1 Å 2 2 Å 12 1 2 Å 11 1 Å 22 2 Å Ï D-optimal continuous design 1 Å 0.096 x2 0.08025 1 0.14575 ¡ 1 x1 1 ¡ Å Ï D-optimal discrete designs n 9: one observation at each point of the Ï Æ continuous D-optimal design n 6: D-optimal exact design does not resemble Ï Æ D-optimal continuous one 30 / 40 Information matrix D-optimal continuous design 2 3 1 0 0 0 0.74 0.74 6 0 0.74 0 0 0 0 7 6 7 6 7 6 0 0 0.74 0 0 0 7 6 7 6 0 0 0 0.58 0 0 7 6 7 40.74 0 0 0 0.74 0.585 0.74 0 0 0 0.58 0.74 31 / 40 Quadratic regression in two variables n 6 n 7 Æ Æ n 8 n 9 D-optimalÆ exact designsÆ 32 / 40 Quadratic design in two variables n 6 n 7 Æ Æ n 8 n 9 D-optimalÆ exact three-levelÆ designs 33 / 40 Quadratic regression in 2 variables 1 run, 2 runs, 3 runs D-optimal exact design n 18 Æ 34 / 40 An industrial example Ï experiment to investigate effect of amount of glycerine (%), x (1 x 3) Ï 1 · 1 · Ï speed temperature reduction (°F/min), x2 (1 x 3) · 2 · Ï response: amount of surviving biological material (%), y Ï context: freeze-dried coffee retaining volatile compounds in freeze-dried coffee is important for its smell and taste Ï Excel file: quadratic3.xls 35 / 40 An industrial example Y ¯ ¯ x ¯ x Ï i Æ 0 Å 1 1i Å 2 2i ¯ x x ¯ x2 ¯ x2 ² Å 12 1i 2i Å 11 1i Å 22 2i Å Ï 9 observations / tests 2 Ï 3 factorial design is optimal Ï what if combination of high x1 and high x2 are not allowed? 36 / 40 Constrained design region 3 x2 2 x1 x2 5 constraintÅ · 1 1 2 3 x1 x1: glycerine, x2: temperature reduction 37 / 40 Constrained design region: solution I scale 32 factorial design down so that it fits in constrained design region 3 x2 2 1 1 2 3 x1 T 1 det(X X)¡ 0.0192 Æ 38 / 40 Constrained design region: solution II move forbidden point (3,3) inward 3 x2 2 1 1 2 3 x1 T 1 det(X X)¡ 0.0006 Æ 39 / 40 Constrained design region: solution III use optimal design approach 3 x2 2 (1.9,1.9) 1 1 2 3 x1 T 1 det(X X)¡ 0.0005 Æ 40 / 40.
Details
-
File Typepdf
-
Upload Time-
-
Content LanguagesEnglish
-
Upload UserAnonymous/Not logged-in
-
File Pages20 Page
-
File Size-