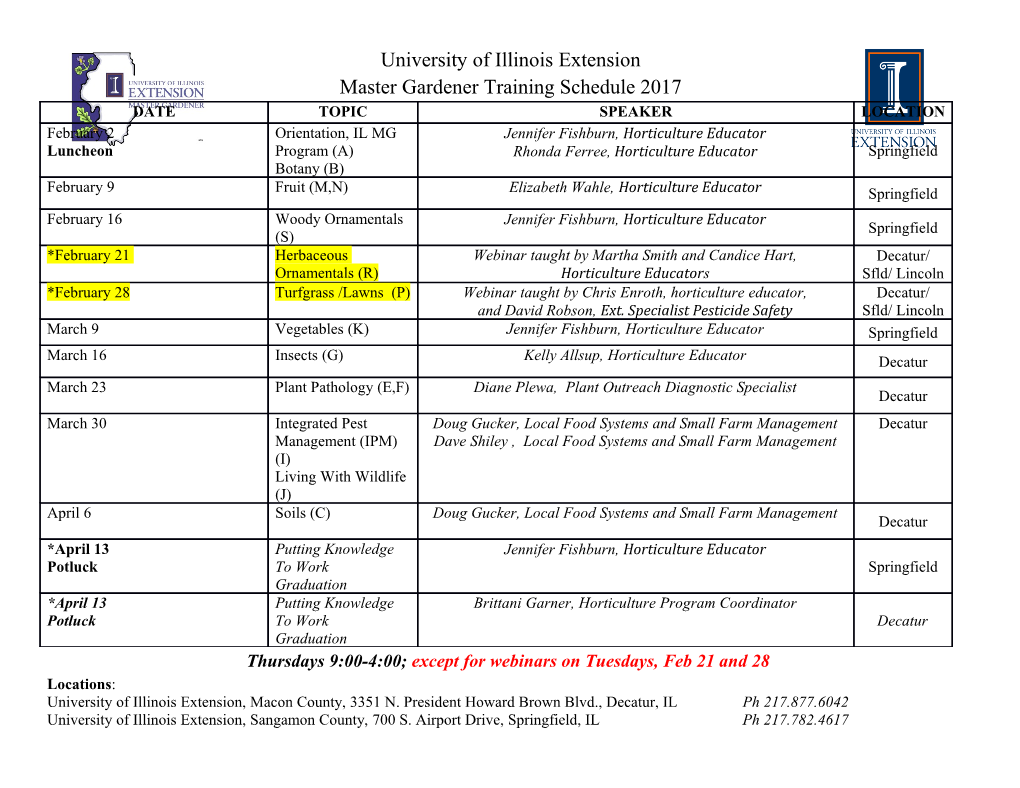
University of Kentucky UKnowledge Biostatistics Faculty Publications Biostatistics 3-19-2019 Feature Selection for Longitudinal Data by Using Sign Averages to Summarize Gene Expression Values over Time Suyan Tian The First Hospital of Jilin University, China, [email protected] Chi Wang University of Kentucky, [email protected] Right click to open a feedback form in a new tab to let us know how this document benefits oy u. Follow this and additional works at: https://uknowledge.uky.edu/biostatistics_facpub Part of the Biostatistics Commons, Computational Biology Commons, Longitudinal Data Analysis and Time Series Commons, and the Microarrays Commons Repository Citation Tian, Suyan and Wang, Chi, "Feature Selection for Longitudinal Data by Using Sign Averages to Summarize Gene Expression Values over Time" (2019). Biostatistics Faculty Publications. 43. https://uknowledge.uky.edu/biostatistics_facpub/43 This Article is brought to you for free and open access by the Biostatistics at UKnowledge. It has been accepted for inclusion in Biostatistics Faculty Publications by an authorized administrator of UKnowledge. For more information, please contact [email protected]. Feature Selection for Longitudinal Data by Using Sign Averages to Summarize Gene Expression Values over Time Notes/Citation Information Published in BioMed Research International, v. 2019, article ID 1724898, p. 1-12. © 2019 Suyan Tian and Chi Wang. This is an open access article distributed under the Creative Commons Attribution License, which permits unrestricted use, distribution, and reproduction in any medium, provided the original work is properly cited. Digital Object Identifier (DOI) https://doi.org/10.1155/2019/1724898 This article is available at UKnowledge: https://uknowledge.uky.edu/biostatistics_facpub/43 Hindawi BioMed Research International Volume 2019, Article ID 1724898, 12 pages https://doi.org/10.1155/2019/1724898 Research Article Feature Selection for Longitudinal Data by Using Sign Averages to Summarize Gene Expression Values over Time Suyan Tian 1,2 and Chi Wang 3,4 Division of Clinical Research, e First Hospital of Jilin University, Xinmin Street, Changchun, Jilin , China Center for Applied Statistical Research, School of Mathematics, Jilin University, Qianjin Street, Changchun, Jilin , China Department of Biostatistics, College of Public Health, University of Kentucky, Lexington, KY , USA Markey Cancer Center, University of Kentucky, Rose St., Lexington, KY , USA Correspondence should be addressed to Suyan Tian; [email protected] and Chi Wang; [email protected] Received 28 November 2018; Accepted 25 February 2019; Published 19 March 2019 Academic Editor: Betti Giusti Copyright © 2019 Suyan Tian and Chi Wang. Tis is an open access article distributed under the Creative Commons Attribution License, which permits unrestricted use, distribution, and reproduction in any medium, provided the original work is properly cited. With the rapid evolution of high-throughput technologies, time series/longitudinal high-throughput experiments have become possible and afordable. However, the development of statistical methods dealing with gene expression profles across time points has not kept up with the explosion of such data. Te feature selection process is of critical importance for longitudinal microarray data. In this study, we proposed aggregating a gene’s expression values across time into a single value using the sign average method, thereby degrading a longitudinal feature selection process into a classic one. Regularized logistic regression models with pseudogenes (i.e., the sign average of genes across time as predictors) were then optimized by either the coordinate descent method or the threshold gradient descent regularization method. By applying the proposed methods to simulated data and a traumatic injury dataset, we have demonstrated that the proposed methods, especially for the combination of sign average and threshold gradient descent regularization, outperform other competitive algorithms. To conclude, the proposed methods are highly recommended for studies with the objective of carrying out feature selection for longitudinal gene expression data. 1. Introduction with expression profles across time points has not kept pace. Feature selection, a mighty tool to tackle the high dimen- One commonly used strategy is to stratify time series sionality issue accompanying high-throughput experiments data into separate time points and then analyze these points where the number of measured features (e.g., genes or separately. Tis approach may lead to inefciency in statistical metabolites), is much larger than that of samples and has been power by ignoring the highly correlated structure of gene employed with increasing frequency in many research areas, expression values across time and thus result in failure to including biomedical research. Te ultimate goal of feature detect patterns of change across time [1–3]. selection is to correctly identify features associated with the An alternative strategy to conduct feature selection for phenotypes of interest while ruling out irrelevant features as longitudinal gene expression data is to use statistical methods much as possible. capable of detecting diferent expression patterns across time Because biological systems or processes are dynamic, between groups. Examples include Signifcance Analysis of it is useful for researchers to investigate gene expression Microarray [4], Extraction of Diferential Gene Expression patterns across time in order to capture biologically mean- (EDGE) [1, 5], Linear Models for Microarray Data (limma) ingful dynamic changes. With the rapid evolution of high- [6], and Microarray Signifcant profles [7]. EDGE uses a throughput technology, time series/longitudinal microarray spline approach and is one of the frst methods to specifcally experiments have become possible and even afordable. address identifcation of diferentially expressed genes across However, development of specifc statistical methods dealing time [8]. In contrast, the limma method has a more general 2 BioMed Research International purpose and is easily understood and implemented [7]; association with the phenotype of interest at diferent time therefore, it has gained extreme popularity and become points, the mean average operator that does not account for the gold standard to detect diferentially expressed genes efect directions may cancel out the diferent time efects of under diferent scenarios (e.g., two-group or multiple-group this gene [20]. In contrast, construction of pathway dereg- comparison) for microarray data. Nevertheless, because the ulation scores is more theoretically complicated and com- limma method usually does not correctly account for the putationally intensive, requiring involvement of an expert order of time points or the correlation structure introduced statistician. For the frst principal component summary, it is by multiple observations from the same subject, it tends well known that the direction of the largest variance of the to be outperformed by other relevant methods. Since these gene expression values is pinpointed instead of the genes that statistical strategies usually screen genes one by one according are most related to the phenotypes of interest. to the magnitude of a gene’s relevance to the phenotype of Te sign average [21, 22], also known as the Direction interest, they may be classifed as the flter methods [9]. Te Aware Average [20], takes into account the directions of big drawback of flter methods is that many false positive association between genes and the phenotype of interest as genes remain in the fnal model [9]. well as genes’ expression values and might be less subject Some researchers have extended two typical longitudinal to overftting since these directions are more robust than data analysis strategies, namely, the generalized estimating their estimated efects [20, 21]. As opposed to the average equation (GEE) method [10] and a mixed model [11], to operator, the sign average considers not only the expression carry out feature selection for time series gene expression values of a gene at each time point but also the direction profles. Te GEE-based screening procedure [3], penalized- of its association with the phenotype of interest at those GEE (PGEE) [2], and glmmLasso [12] methods belong to this time points; therefore, positive and negative associations do category. Among them, the GEE-based screening procedure not cancel each other out. Te sign average is an average in fts a GEE model to each gene and then flters out the non- essence, however, and not as sophisticated as the pathway signifcant genes. By fltering genes one by one, this procedure deregulation scores. Given the fact that the sign average is is very likely to mistakenly include redundant genes highly capable of mitigating these two drawbacks simultaneously, correlated with the true relevant genes in the fnal gene list. it may be a more suitable choice to summarize a genes’ Te PGEE algorithm [2] adds the SCAD penalty term [13] to expression value at the gene set level or a gene’s expression the corresponding quasilikelihood function of a GEE model values over time. to implement feature selection and model construction. In In this study, we consider a scenario that has a long contrast, the glmmLasso method [12] maximizes the corre- history—traumatic injury with subsequent infection. In sponding penalized log likelihood function of a generalized ancient times, traumatic injury with
Details
-
File Typepdf
-
Upload Time-
-
Content LanguagesEnglish
-
Upload UserAnonymous/Not logged-in
-
File Pages15 Page
-
File Size-