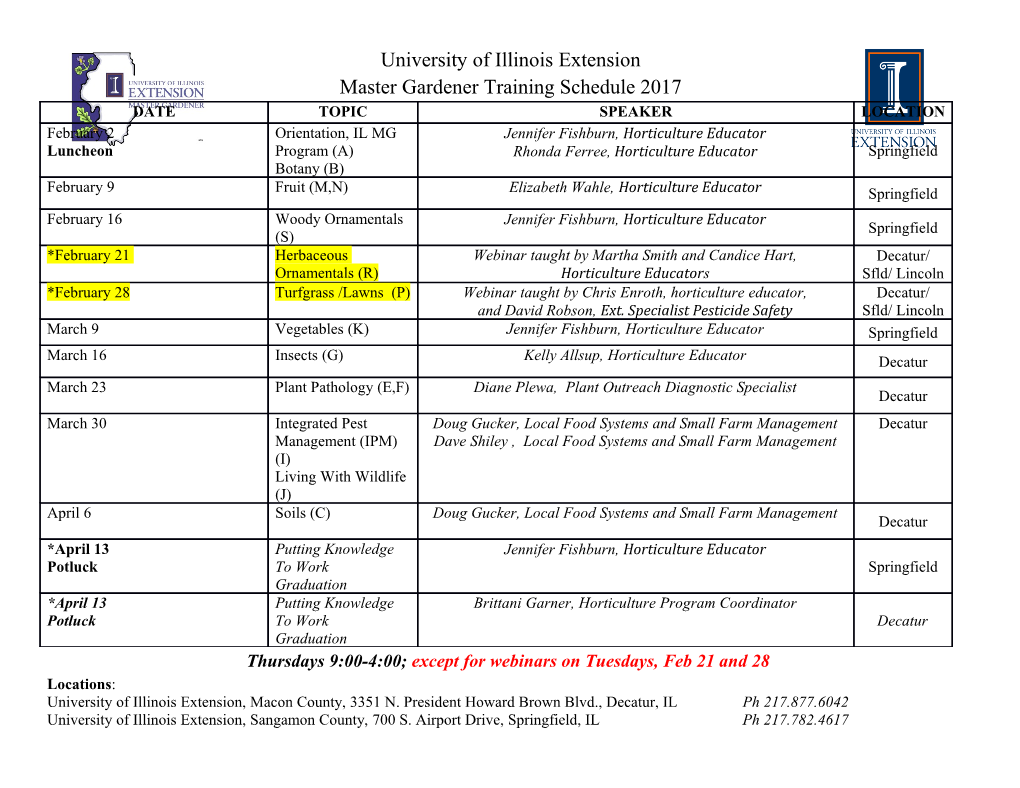
The non-central Nakagami distribution Søren Hauberg Section for Cognitive Systems Technical University of Denmark [email protected] Abstract The Nakagami distribution describe the square root of a random variable drawn from a Gamma distribution. Equivalently, the Gamma distribution can be seen as a one-dimensional Wishart distribution. In this note, we consider the distribution of the square root of a random variable drawn from a non-central one-dimensional Wishart distribution. We present the probability density function of this distribution along with closed-form expressions for its moments. This document is just a short technical note. Comments can be directed at [email protected] 1 The Nakagami distribution 2 Let zd ∼ N (0; σ ); d = 1;:::;D be iid samples from a zero-mean normal distribution. Then D X 2 + x = zd 2 R (1.1) d=1 follows a Gamma distribution. Equivalently, and more suitable for our purposes, x also follows a one-dimensional Wishart distribution [1], 2 x ∼ W1(D; σ ) (1.2) r D−2 2 x −1 x p(x) = W (x j D; σ ) = Γ(D=2) exp − : (1.3) 1 2D σ2D 2σ2 This distribution can be seen as describing the squaredp norm of a zero-mean normally distributed vector. The norm of this vector is then given by y = x, which follows a Nakagami distribution [2], 2 y ∼ Nakagami D=2; Dσ (1.4) 2 2 2 D−1 y D 2 p p(y) = Nakagami y j = ; Dσ = y exp − 2 : (1.5) Γ(D=2)( 2σ)D 2σ This expression is easily derived by the change of value theorem; see next section. The expectation and variance of a Nakagami distributed variable is D+1 p Γ 2 E [y] = D 2σ (1.6) Γ 2 2! Γ((D + 1)=2) var [y] = σ2 D − 2 : (1.7) Γ(D=2) We will derive a more general version of these results in the next section. Preprint. Work in progress. 2 The non-central Nakagami distribution 2 Now, let zd ∼ N (µd; σ ); d = 1;:::;D be independent samples from normal distributions with variance σ2. Then D X 2 + x = zd 2 R (2.1) d=1 follows a one-dimensional non-central Wishart distribution [1], 2 x ∼ W1(D; σ ; Ω) (2.2) PD µ2 Ω = d=1 d (2.3) σ2 2 p(x) = W1(x j D; σ ; Ω) r D−2 (2.4) x −1 −2 x Ω = Γ(D=2) F D=2; 1=4Ωσ x exp − exp − : 2D σ2D 0 1 2σ2 2 Here 0F1 is a generalized hypergeometric function; see Sec. 7.3 of Muirhead’s book [1]. This distribution can be seen as describing the squared norm ofp a normal distributed vector with isotropic variance. The norm of this vector is then given by y = x, which we say follows a non-central Nakagami distribution. This distribution can be derived by change-of-variables to be 2 2 p (y) = W1(y j D; σ ; Ω) · 2y (2.5) yD−1 D y2 y2 Ω = (D−2) 0F1 ; Ω exp − exp − : (2.6) 2 =2 σD Γ(D=2) 2 4σ2 2σ2 2 p To compute the moments of x we recall the following result from Muirhead [1, Theorem 10.3.7]. q×q Let X 2 R and X ∼ Wq(D; Σ; Ω) then D k k qk Γq( =2 + k) (det(X)) = (det(Σ)) 2 F (−k; D=2; −1=2Ω); (2.7) E D 1 1 Γq( =2) p where 1F1 is a generalized hypergeometric function. Since y = x is a positive scalar, its determinant is merely y, and we get hp 2ki Γ(D=2 + k) 2k k D 1 E x = σ 2 1F1(−k; =2; − =2Ω): (2.8) Γ(D=2) p From this we see that the mean and the variance of y = x is p Γ((D+1)=2) 1=2 1 D 1 E x = σ2 1F1(− =2; =2; − =2Ω) (2.9) Γ(D=2) Γ((D+2)=2) 2 D 1 E [x] = σ 2 1F1(−1; =2; − =2Ω) (2.10) Γ(D=2) p p 2 var x = E x − E x (2.11) σ22 D + 2 D 1 = Γ 1F1(−1; =2; − =2Ω) Γ(D=2) 2 (2.12) 2 ! Γ((D+1)=2) 1 D 1 2 − 1F1(− =2; =2; − =2Ω) : Γ(D=2) Acknowledgments. The author was supported by a research grant (15334) from VILLUM FONDEN. This project has received funding from the European Research Council (ERC) under the European Union’s Horizon 2020 research and innovation programme (grant agreement no 757360). References [1] R. J. Muirhead. Aspects of Multivariate Statistical Theory. John Wiley & Sons, 2005. [2] M. Nakagami. The m-distribution, a general formula of intensity of rapid fading, iwc hoffman, ed, 1960. 2.
Details
-
File Typepdf
-
Upload Time-
-
Content LanguagesEnglish
-
Upload UserAnonymous/Not logged-in
-
File Pages2 Page
-
File Size-