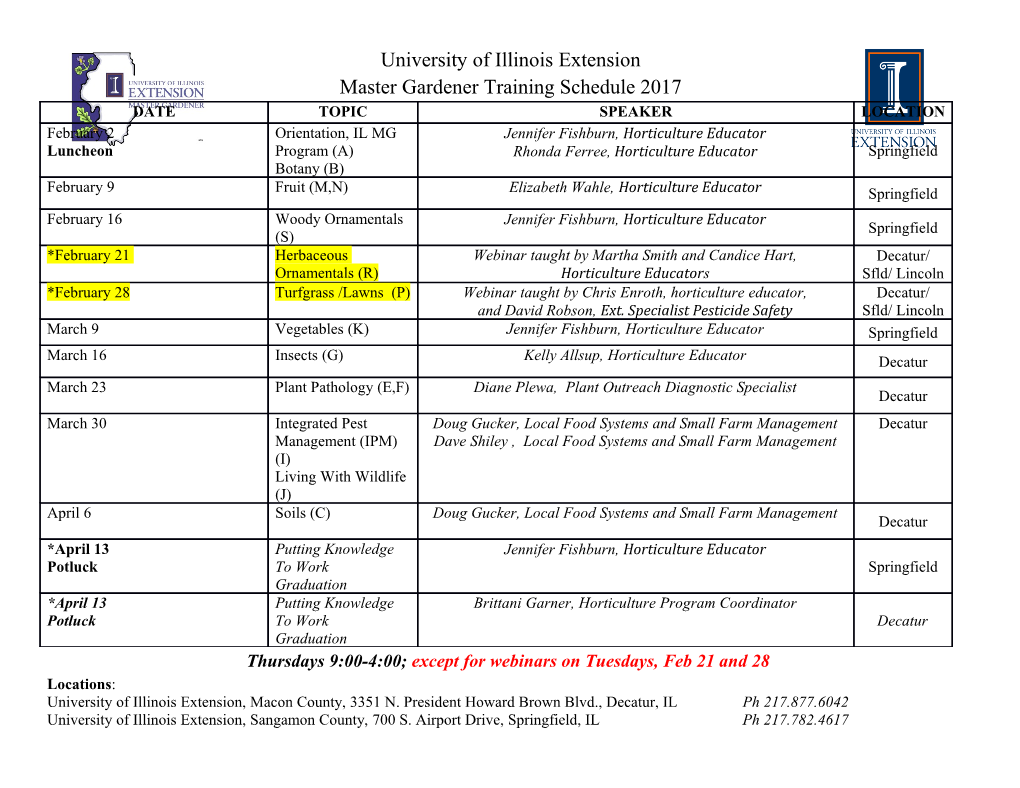
Discrete Distributions Chapter 6 Negative Binomial Distribution section 6.3 Consider k = r; r + 1; ::: independent Bernoulli trials with probability of success in one trial being p. Let the random variable X be the trial number k needed to have r-th success. Equivalently in the first k − 1 trials there are r − 1 successes (no matter when they have occurred) and the k-th trial must be a success. Since the trials are independent, the required probability is found by multiplying the two sub-probabilities: ( ) ( ) k − 1 k − 1 pr−1(1 − p)k−r × p = pr(1 − p)k−r r − 1 r − 1 where here we are assuming that k = r; r + 1; ::: . Putting n = k − r , or equivalently k = n + r , we can write the above equality in terms of n : ( ) n + r − 1 P (Y = n) = pr(1 − p)n n = 0; 1; 2; ::: where Y = X − r r − 1 ( ) ( ) n+r−1 n+r−1 But we know from \Combinatorics" that r−1 = n , therefore we have ( ) n + r − 1 P (Y = n) = pr(1 − p)n n = 0; 1; 2; ::: n 1 Finally by a change of variable p = 1+β , we can write: ( )( ) ( ) n + r − 1 1 r β n P (Y = n) = n = 0; 1; 2; ::: n 1 + β 1 + β Recall that ( ) n + r − 1 (n + r − 1)(n + r − 2) ··· (r) = n n! therefore: ( ) ( ) (n + r − 1)(n + r − 2) ··· (r) 1 r β n P (Y = n) = n = 0; 1; 2; ::: n! 1 + β 1 + β 1 In this new form , r can be taken to be any positive number (and not just a positive integer). So the negative binomial distribution has two positive parameters β > 0 and r > 0 . This distribution has an advantage over the Poisson distribution in modeling because it has one more parameter. To be able to use the table of the textbook directly , let us change n to k thoroughly: ( ) ( ) (k + r − 1)(k + r − 2) ··· (r) 1 r β k P (Y = k) = k = 0; 1; 2; ::: k! 1 + β 1 + β z k terms}| { (r)(r + 1) ··· (k + r − 1) βk P (Y = k) = k = 0; 1; 2; ::: k! (1 + β)k+r (k+r−1)(k+r−2)···(r) We need the values k! to be able to calculate these probabilities. But note that (k + r − 1)(k + r − 2) ··· (r) Γ(k + r) Γ(k+r) = (k+r−1)(k+r−2) ··· (r)Γ(r) ) = k! Γ(k + 1) Γ(r) and tables of the values the Gamma function or log-Gamma functions are provided in (k+r−1)(k+r−2)···(r) programming languages, so you can calculate the values k! through the Γ(k+r) formula Γ(k+1) Γ(r) . Here is the reason for choosing the name \Negative Binomial" For a moment let us recall the binomial series we have learned in Calculus: 1 X (α)(α − 1) ··· (α − k + 1) (1 + z)α = 1 + zk k! k=1 which is valid for all real number α and all − < z < 1. As an example: X1 − 1 − 5 ··· − 1 − 1 − 1 ( 4 )( 4 ) ( 4 k + 1) k p = (1 + z) 4 = 1 + z − 1 < z < 1 4 1 + z k! k=1 2 Now change z to −z , and substitute −r for α to get: P − −r 1 (−r)(−r−1)···(−r−k+1) − k (1 z) = 1 + k=1 k! ( z) P 1 (r)(r+1)···(r+k−1) k − k = 1 + k=1 k! z dropping ( 1) P 1 (r+k−1)(r+k−2)···(r) k = 1 + k=1 k! z rearranging P ( ) 1 k+r−1 k = k=0 r−1 z The series expansion 1 ( ) 1 X k + r − 1 (1 − z)−r = = zk (1 − z)r r − 1 k=0 is called the negative binomial expansion. Now we calculate the PGF of the negative binomial distribution. 1 1 ( )( ) ( ) X X k + r − 1 1 r β k P (z) = E(zN ) = zk P (N = k) = zk N r − 1 1 + β 1 + β k=0 k=0 ( ) 1 ( )( ) ( ) 1 ( )( ) 1 r X k + r − 1 β k 1 r X k + r − 1 zβ k = zk = 1 + β r − 1 1 + β 1 + β r − 1 1 + β k=0 k=0 ( ) ( )− ( ) ( )− 1 r zβ r 1 r 1 + β(1 − z) r = 1 − = 1 + β 1 + β 1 + β 1 + β ( ) ( ) 1 r 1 + β r 1 1 = = = 1 + β 1 + β(1 − z) (1 + β(1 − z))r (1 − β(z − 1))r Expected value and Variance p(z) = (1 + β(1 − z))−r 8 < 0 − − p (z) = rβ(1 + β(1 − z)) r 1 ) : p00(z) = r(r + 1)β2(1 + β(1 − z))−r−2 3 8 < 0 p (1) = rβ ) : p00(1) = r(r + 1)β2 8 < 0 E(N) = p (1) = rβ ) : Var(N) = p00(1) + p0(1)(1 − p0(1)) = ··· = rβ + rβ2β = rβ(1 + β) Note. As we see, in the negative binomial distribution , the variance is larger than the expected value while in the Poisson distribution they are equal, therefore in modeling the data in which the sample variance seems to be larger than the sample mean , the negative binomial distribution is preferred over the Poisson distribution. If PX (z) is the PGF of X , then 8 > 0 <> E(X) = p (1) > :> Var(X) = p00(1) + p0(1)(1 − p0(1)) Theorem (Poisson as a limit of NBinomials). Let Xn ∼ NBinomial(rn ; βn) such that d rn ! 1 and βn ! 0 and rnβn ! λ > 0. Then Xn ! Poisson(λ) Note. Before proving this theorem , we recall from Calculus that when x ! 0 , then the ln(1+x) functions ln(1 + x) and x are equivalent in the sense that limx!0 x = 1. To see this equality apply the L'Hospital rule. Once we have this , then in the quotients we can substitute x for ln(1 + x) whenever x ! 0. In fact, if g(x) is any function of x , then ( )( ) ln(1 + x) ln(1 + x) x ln(1 + x) x x lim = lim = lim lim = lim x!1 g(x) x!1 x g(x) x!1 x x!1 g(x) x!1 g(x) Proof of the Theorem. Set λn = rnβn. Then from the assumption we have λn ! λ. 4 Further: ( ) −r − n limn!1 PXn (z) = lim 1 + βn(1 z) h i = lim exp − rn ln(1 + βn(1 − z)) h n oi = exp lim − rn ln(1 + βn(1 − z)) h n oi ln(1+βn(1−z)) = exp lim − 1 rn h n − oi ln(1+ λn(1 z) ) rn = exp lim − 1 rn h n λn(1−z) oi rn = exp lim − 1 rn h n oi = exp lim − λn(1 − z) = exp(λ(z − 1)) So , we have proved that the PGF of the sequence Xn's tends to the PGF of the Poisson(λ) distribution. Whence the claim. 5 Geometric Distribution The Geometric random variable with parameter 0 < q < 1 is a variable with support f1 ; 2 ; ::::g such that X = k is the event that in a series of Bernoulli trials the first success occurs at time k. Since the first k − 1 trials should result in a failure, then P (X = k) = q (1 − q)k−1 k = 1; 2; ::: Note that n } P (X ≥ m) = q(1 − q)m−1 + q(1 − q)m + q(1 − q)m+1 + ··· n o 1 = q(1 − q)m−1 1 + (1 − q) + (1 − q)2 + ··· = q(1 − q)m−1 = (1 − q)m−1 1 − (1 − q) Then for k = 1; 2; ::: we have : P (X ≥ n + k ; X ≥ n) P (X ≥ n + k) P (X ≥ n + k j X ≥ n) = = P (X ≥ n) P (X ≥ n) (1 − q)n+k−1 = = (1 − q)k (1 − q)n−1 This property is called the memoryless property. Given that there are at least n claims, the probability distribution of the number of claims in excess of n does not depend on n : P (X = n + k j X ≥ n) = P (X ≥ n + k j X ≥ n) − P (X ≥ n + k + 1 j X ≥ n) = (1 − q)k − (1 − q)k+1 does not depend on n A random variable X has memoryless distribution if for all x's the conditional distribution (X j X ≥ x) is the same for all x's. We may consider the Geometric distribution as a special case of Negative Binomial : In fact by 1 changing k to k + 1 and substituting 1+β for p we can write the probabilities in the new form: ( )( ) 1 β k βk Geometric(β) P (N = k) = = k = 0; 1; ::: 1 + β 1 + β (1 + β)k+1 So, a Geometric distribution is a Negative Binomial distribution with r = 1. Note that in this 6 new shape , we have 1 P (N = 0) = 1 + β i.e. the value of the probability function at k = 0 equals the probability of success. 7 (a,b,0) class section 6.5 Definition. Let pk = P (X = k), k = 0; 1; 2; ::: be the probabilities of a discrete random variable. If there are two numbers a and b satisfying p b equivalently p k = a + () k k = ak + b k = 1; 2; ::: pk−1 k pk−1 then we say that X belongs to the class (a; b; 0). Note that the following four distributions are in class (a; b; 0) : Distribution a b p0 − q − − m Binomial(m; q) 1−q (m + 1)a (1 q) β − −r Negative Binomial(r; β) 1+β (r 1)a (1 + β) β −1 Geometric(q) 1+β 0 (1 + β) Poisson(λ) 0 λ e−λ As it has been shown in the literature , these four distributions are the only non-trivial distributions of the class (a; b; 0) Example ∗.
Details
-
File Typepdf
-
Upload Time-
-
Content LanguagesEnglish
-
Upload UserAnonymous/Not logged-in
-
File Pages19 Page
-
File Size-