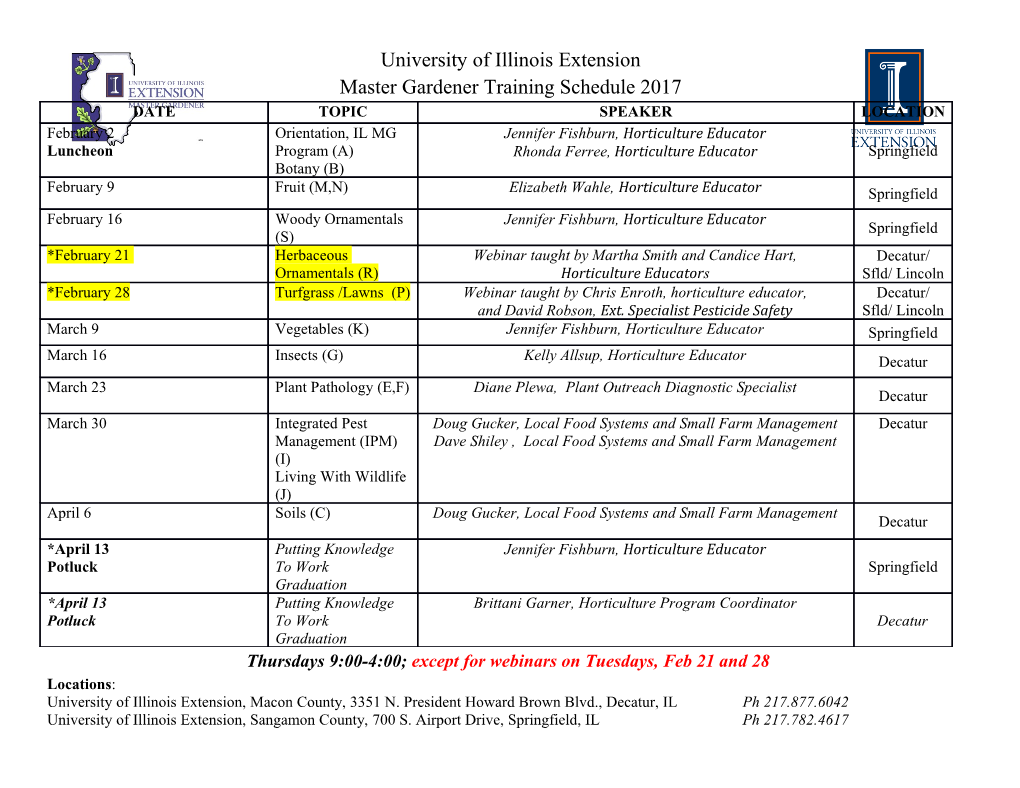
Master Thesis Electrical Engineering Micro-Expression Extraction For Lie Detection Using Eulerian Video (Motion and Color) Magnification Submitted By Gautam Krishna , Chavali Sai Kumar N V , Bhavaraju Tushal , Adusumilli Venu Gopal , Puripanda This thesis is presented as part of Degree of Master of Sciences in Electrical Engineering BLEKINGE INSTITUTE OF TECHNOLOGY AUGUST,2014 Supervisor : Muhammad Shahid Examiner : Dr. Benny L¨ovstr¨om Department of Applied Signal Processing Blekinge Institute of Technology; SE-371 79, Karlskrona, Sweden. This thesis is submitted to the Department of Applied Signal Processing at Blekinge Institute of Technology in partial fulfilment of the requirements for the degree of Master of Sciences in Electrical Engineering with emphasis on Signal Processing. Contact Information Authors: Gautam Krishna.Chavali E-mail: [email protected] Sai Kumar N V.Bhavaraju E-mail: [email protected] Tushal.Adusumilli E-mail: [email protected] Venu Gopal.Puripanda E-mail: [email protected] University Advisor: Mr. Muhammad Shahid Department of Applied Signal Processing Blekinge Institute of Technology E-mail: [email protected] Phone:+46(0)455-385746 University Examiner: Dr. Benny L¨ovstr¨om Department of Applied Signal Processing Blekinge Institute of Technology E-mail: [email protected] Phone: +46(0)455-38704 School of Electrical Engineering Blekinge Institute of Technology Internet : www.bth.se/ing SE-371 79, Karlskrona Phone : +46 455 38 50 00 Sweden. Abstract Lie-detection has been an evergreen and evolving subject. Polygraph techniques have been the most popular and successful technique till date. The main drawback of the polygraph is that good results cannot be attained without maintaining a physical con- tact, of the subject under test. In general, this physical contact would induce extra consciousness in the subject. Also, any sort of arousal in the subject triggers false posi- tives while performing the traditional polygraph based tests. With all these drawbacks in the polygraph, also, due to rapid developments in the fields of computer vision and artificial intelligence, with newer and faster algorithms, have compelled mankind to search and adapt to contemporary methods in lie-detection. Observing the facial expressions of emotions in a person without any physical con- tact and implementing these techniques using artificial intelligence is one such method. The concept of magnifying a micro expression and trying to decipher them is rather premature at this stage but would evolve in future. Magnification using Eulerian Video Magnification(EVM) technique has been proposed recently and it is rather new to ex- tract these micro expressions from magnified EVM based on Histogram of Oriented Gradients (HOG) features. HOG features is the feature extraction algorithm which ex- tracts local gradient information in an image. Till date, HOG features have been used in conjunction with SVM, and generally for person/pedestrian detection. A newer, sim- pler and modern method of applying EVM with HOG features and Back-propagation Neural Network jointly has been introduced and proposed to extract and decipher the micro-expressions on the face. Micro-expressions go unnoticed due to its involuntary nature, but EVM is used to magnify them and makes them noticeable. Emotions be- hind the micro-expressions are extracted and recognized using the HOG features & Back-Propagation Neural Network. One of the important aspects that has to be dealt with human beings is a biased mind. Since, an investigator is also a human and, he too, has to deal with his own assumptions and emotions, a Neural Network is used to give the investigator an unbiased start in identifying the true emotions behind every micro-expression. On the whole, this proposed system is not a lie-detector, but helps in detecting the emotions of the subject under test. By further investigation, a lie can be detected. Keywords: Micro Expressions, Emotions, Eulerian Video Magnification, Histogram of Oriented Gradients, Voila-Jones Algorithm, Artificial Neural Network. iii Acknowledgments We would like to express gratitude to our supervisor Mr.Muhammad Shahid for intro- ducing us to the topic and for the heartfelt support and encouragement on this pursuit of our masters. Furthermore, we would like to thank Dr. Benny L¨ovstr¨om for his useful comments and remarks through the learning process of this master thesis. The Department of Signal Processing has provided the support and equipment that are mandatory to produce and complete our thesis. In our daily work we have been blessed with a friendly and cheerful group of fellow students (PVK Chaitanya and V Revanth). We will forever be grateful for all your love and help. Finally, we thank our family members and relatives for supporting us throughout all our studies at University and helping us to move across the seas. Also, for providing a second home here, wherein we could complete our writing up. v Contents Abstract iii Acknowledgments v List of Abbreviations xii 1 Introduction 1 1.1 Objectives and Scope of work ....................... 2 1.1.1 Pre-requisitesforthemethodology................ 2 1.2 Research Questions ............................. 2 1.3TheMethod................................. 2 1.4BlockDiagram............................... 3 1.5OverviewofThesis............................. 4 2 Lies, Expressions and Emotions 5 2.1Lie...................................... 5 2.2FacialExpressions............................. 6 2.3Emotions.................................. 7 2.3.1 Anger................................ 7 2.3.2 Disgust............................... 8 2.3.3 Fear................................. 8 2.3.4 Happy................................ 9 2.3.5 Sadness............................... 9 2.3.6 Surprise............................... 9 2.3.7 Contempt.............................. 10 2.4 Micro Expressions .............................. 11 3 Eulerian Video Magnification 13 3.1 Conceptual learning about Pyramids ................... 13 3.1.1 GaussianPyramid......................... 14 3.1.2 Laplacian pyramids ......................... 15 3.2VideospecificationsandprerequisitesofEVM.............. 15 3.2.1 Standardone-to-oneinterviewsetupformat........... 15 3.3 Eulerian Video Magnification ....................... 16 3.3.1 Motion Magnification ........................ 16 3.3.2 Color Magnification ......................... 17 4 Face Recognition and Feature Extraction 20 4.1 Conceptual learning about Voila-Jones algorithm ............ 20 4.1.1 Haar-Likefeatures......................... 20 4.1.2 IntegralImage............................ 21 4.1.3 AdaBoost.............................. 22 4.1.4 CascadeofClassifiers........................ 22 4.2RecognitionofFaceusingVoila-JonesAlgorithm............. 22 vii 4.3 Conceptual learning about HOG features ................. 22 4.3.1 Gradient computation ....................... 23 4.3.2 OrientationBiANNing....................... 23 4.3.3 Descriptor Blocks .......................... 23 4.3.4 BlockNormalization........................ 23 4.4FeatureExtractionusingHOGfeatures.................. 24 5 Database of Images 26 5.1Cohn-Kanadeimagedatabase....................... 26 5.2ExtendedCohn-Kanadeimagedatabase................. 26 5.3 Discussion about emotion labels in extended Cohn-Kanade database . 27 5.3.1 AdvantagesofusingANNovermulti-classSVM......... 28 5.4WorkingwithimagesofextendedCohn-Kanadedatabase....... 28 6 Neural Network and Pulse extraction 30 6.1 Artificial Neural Network using back propagation algorithm ...... 31 6.1.1 Training phase ........................... 31 6.1.2 Testingphase............................ 33 6.2 Pulse Extraction .............................. 34 6.3Outputsrenderedasmovinggraphs.................... 34 7 Graphical User Interface 36 7.1WorkingonGUI.............................. 36 7.2OperationalflowofGUI.......................... 37 8 Results 40 8.1Performance................................. 40 8.1.1 SystemSpecifications........................ 40 8.1.2 InputSpecification......................... 40 8.1.3 TimeDuration........................... 41 8.2 Validating the Neural Network results with the results of the database . 41 8.3 Reasons for not designing and performing an experiment ........ 42 8.4 Verifying the motion magnification of the proposed design ....... 43 8.4.1 Anger................................ 43 8.4.2 Disgust-DF1di........................... 43 8.4.3 Fear-DM2fe............................ 44 8.4.4 Happy-DF1ha........................... 45 8.4.5 Sad-DF1sa............................. 46 8.4.6 Surprise-DF1su.......................... 47 8.5 Verifying both motion and color magnification of the proposed design . 49 8.5.1 Results for angry emotion using motion magnification of subject-1 49 8.5.2 Results for angry emotion using color magnification of subject-1 50 8.5.3 Results for various emotions using motion magnification of subject- 2................................... 50 8.5.4 Results for various emotions without motion magnification of subject 2 ............................... 52 8.5.5 Results for various emotions using color magnification of subject-2 52 9 Conclusion and Future Works 54 9.1Conclusion.................................. 54 9.2Futureworks................................ 54 Bibliography 56 List of Figures 1.1 Block diagram representing methodology ................. 3 2.1 Macro facial expression of a subject . ................. 6 2.2Angry-facialemotion............................ 7 2.3Disgust-facialemotion.........................
Details
-
File Typepdf
-
Upload Time-
-
Content LanguagesEnglish
-
Upload UserAnonymous/Not logged-in
-
File Pages72 Page
-
File Size-