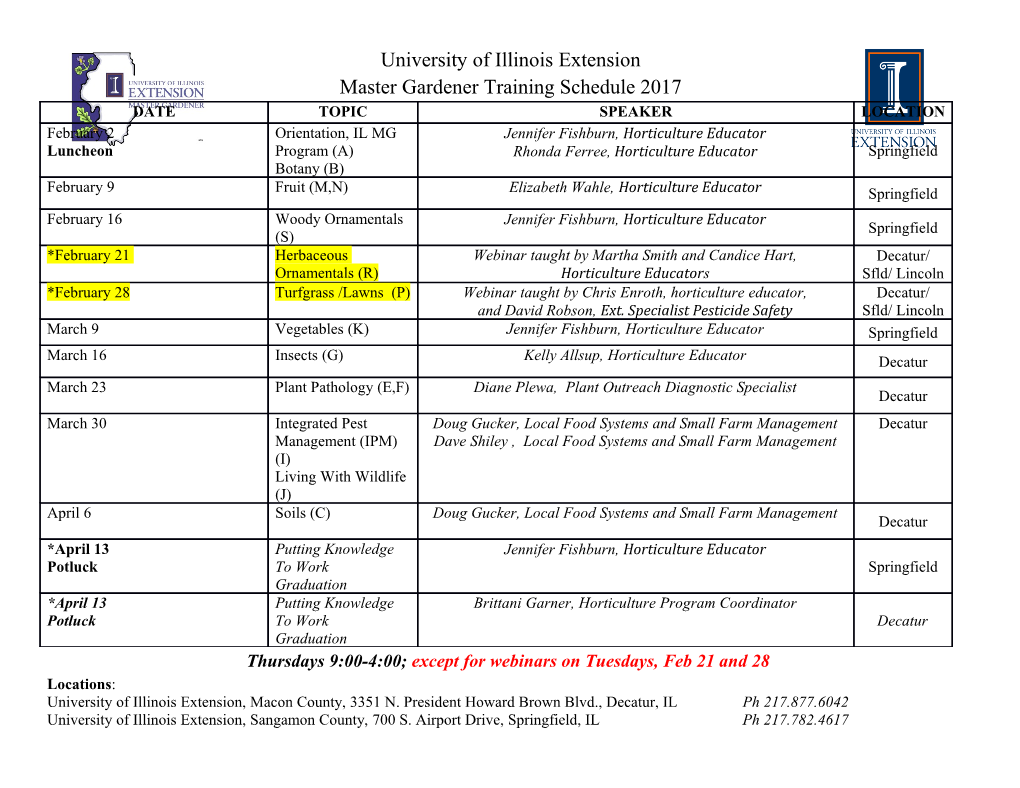
Introduction to Computational Chemical Biology Dr. Raimo Franke [email protected] Lecture Series Chemical Biology http://www.raimofranke.de/ Leibniz-Universität Hannover Where can you find me? • Dr. Raimo Franke Department of Chemical Biology (C-Building) Helmholtz Centre for Infection Research Inhoffenstr. 7, 38124 Braunschweig Tel.: 0531-6181-3415 Email: [email protected] Download of lecture slides: www.raimofranke.de • Research Topics - Metabolomics - Biostatistical Data Analysis of omics experiments (NGS (genome, RNAseq), Arrays, Metabolomics, Profiling) - Phenotypic Profiling of bioactive compounds with impedance measurements (xCelligence) (Peptide Synthesis, ABPP) I am looking for BSc, MSc and PhD students, feel free to contact me! Slide 2 | My journey… Slide 3 | Outline Primer on Statistical Methods Multi-parameter phenotypic profiling: using cellular effects to characterize bioactive compounds Network Pharmacology, Modeling of Signal Transduction Networks Paper Presentations: Proc Natl Acad Sci U S A. 2013 Feb 5;110(6):2336-41. doi: 10.1073/pnas.1218524110. Epub 2013 Jan 22. Antimicrobial drug resistance affects broad changes in metabolomic phenotype in addition to secondary metabolism. Derewacz DK, Goodwin CR, McNees CR, McLean JA, Bachmann BO. Slide 4 | Chemical Biology Arsenal of Methods In silico Target interactions Correlation signals Chemical Genetics & predictions Chemoproteomics Pharmacophore Biochemical assays Expression profiling Protein microarrays –based target SPR, ITC Proteomics Yeast-3-Hybrid prediction X-Ray, NMR Metabonomics Phage display Image analysis ABPP Impedance Affinity pulldown NCI60 panel Chemical probes Computational Methods in Chemical Biology • In silico –based target prediction: use molecular descriptors for in silico screening, molecular docking etc. (typical applications of Cheminformatics) • Biochemical Assays: SPR: curve fitting to determine kass und kdiss, Xray: model fitting, NMR: chemical shift prediction etc. • Phenotypic Profiling / HCS: mining of omics data (Proteomics, Metabolomics , automated microscopy etc.): multivariate statistics • Chemical Systems Biology: Network Pharmacology (graph theory, ODEs) All approaches have in common: application of computational/statistical methods is a key step for data analysis -> Importance of statistics and statistical data mining Slide 6 | Short Primer on Statistical Methods • Intro to statistics slides partly © Professor David Wishart, University of Alberta, Canada • Free to download, share and remix: http://bioinformatics.ca/ Slide 7 | Statistics • “There are three kinds of lies: lies, damned lies, and statistics” Benjamin Disraeli (British Prime Minister in 1870ies) • Statistics is a formalized way of describing impressions and provides a framework for decision making • I.e. formalizes the subjective impression that population A is on average taller than population B by calculating a quantitative parameter: e.g. mean or median • In Chemical Biology Research statistics becomes more and more important: from simple t-tests to evaluate results of Western Blots to complex multivariate statistics to analyze results from omics experiments • -> It is vital to have a solid understanding of statistics Review of some aspects of Statistics • Descriptive vs. inferential statistics • Normal Distribution • T-Test and p-value • Correlation • Clustering • Multivariate Statistics: PCA Slide 9 | Statistical methods used in data analysis • Descriptive Statistics : summarizes data from a sample • Inferential Statistics : draws conclusions from data that are subject to random variation (e.g. sampling variation). It tries to make inferences about a population using information from samples (drawn form the population). • Very important distinction: sample <-> population • If the population is too big to directly characterize it with descriptive statistics: draw samples -> from the samples inferences can be made to characterize the underlying population • Usually: sample mean ≠ population mean Slide 10 | Distributions • Underlies all of statistics • Distribution of a single variable over a population: e.g. height of population: Univariate Statistics Slide 11 | Univariate Statistics • Univariate means a single variable • If you measure a population using some single measure such as height, weight, test score, IQ, you are measuring a single variable • If you plot that single variable over the whole population, measuring the frequency that a given value is reached you will get the following: A Bell Curve Carl Friedrich Gauß (*1777 in Braunschweig) # of of of each each # # Height Also called a Gaussian or Normal Distribution Features of a Normal Distribution Two key parameters characterize any kind of distribution: central tendency and spread µ = mean • Symmetric Distribution • Has an average or mean value ( µ) at the centre • Has a characteristic width called the standard deviation ( σ) • Most common type of distribution known Normal Distribution • Almost any set of biological or physical measurements will display some variation and these will almost always follow a Normal distribution • The larger the set of measurements, the more “normal” the curve • Minimum set of measurements to get a normal distribution is 30- 40 • -> For any statistical test relying on the normal distribution (e.g. T- test) that means that we would at least need 30 biological replicates (often not feasible) Gaussian Distribution P(x) - probability density distribution − µ 2 − ( x ) 1 2 P ( x) = e 2σ 2πσ −x2 ~ e µ-3 σ µ-2 σ µ-σ µ µ+σ µ+2 σ µ+3 σ Some Equations µ Σ Mean = xi N σ2 Σ µ 2 Variance = (x i - ) N σ Σ µ 2 Standard Deviation = (x i - ) N uncorrected sample standard deviation (sample considered as the entire population) corrected standard deviation, σ = Σ(x - µ)2 when used as estimator for population i standard deviation N-1 The uncorrected sample sd underestimates the population variance, to correct for this bias: 1/N-1 Mean, Median & Mode • Gaussian Normal Distribution: symmetrical, many other distributions are not • Other distributions: Binomial, Poisson • Skewed distribution are often the case for real life measurement Mode Median Mean Mean, Median, Mode • In a Normal Distribution the mean, mode and median are all equal • In skewed distributions they are unequal • Mean - average value, affected by extreme values in the distribution • Median - the “middlemost ” value, usually half way between the mode and the mean • Mode - most common value Probability Distributions with Standard Deviations (z-values, z-scores) P not within AUC boarders µ ± 1.0 S.D. 0.683 > µ + 1.0 S.D. 0.158 P=32% µ ± 2.0 S.D. 0.954 > µ + 2.0 S.D. 0.023 P=5% µ ± 3.0 S.D. 0.9972 > µ + 3.0 S.D. 0.0014 P=0.3% µ ± 4.0 S.D. 0.99994 > µ + 4.0 S.D. 0.00003 µ ± 5.0 S.D. 0.999998 > µ + 5.0 S.D. 0.000001 − µ 2 P − ( x ) 1 2 P ( x) = e 2σ 2πσ µ-3 σ µ-2 σ µ-σ µ µ+σ µ+2 σ µ+3 σ z=-3 z=-2 z=-1 z=0 z=1 z=2 z=3 Significance • Based on the Normal Distribution, the probability that something is >1 SD away (larger or smaller) from the mean is 32% • Based on the Normal Distribution, the probability that something is >2 SD away (larger or smaller) from the mean is 5% • Based on the Normal Distribution, the probability that something is >3 SD away (larger or smaller) from the mean is 0.3% The P-value • The p-value is the probability of obtaining a test statistic (a score, a set of events, a height) at least as extreme as the one that was actually observed (probability of an observed event purely arising by chance) • One "rejects the null hypothesis" when the p-value is less than the significance level α which is often 0.05 or 0.01 • When the null hypothesis is rejected, the result is said to be statistically significant Box-plot Descriptive statistics can be 1.5 x IQR whisker intuitively summarized in a 75% quantile Box-plot. IQR Median 25% quantile 1.5 x IQR whisker Everything above and below 1.5 x IQR is considered an "outlier". IQR = Inter Quantile Range = 75% quantile – 25% quantile Boxplots and Normal Distribution particularly useful for comparing distributions between several groups or sets of data Slide 24 | Application Example: Edge Effect and outlier detection Label-freeSlide 25 | phenotypic profiling Application: Regulation of a metabolite in Pseudomonas aeruginosa (∆pqsE vs. wt) featureidx fold log2fold tstat pvalue 52 90,49660842 -6,49979182 -26,68745376 0,00138943 KO wt Slide 26 | Fixing a Skewed Distribution • A skewed distribution or exponentially decaying distribution can be transformed into a “normal” or Gaussian distribution by applying a log transformation • This brings the outliers a little closer to the mean because it rescales the x-variable, it also makes the distribution much more Gaussian Log Transformation Skewed distribution Normal distribution linear scale log 0.5 transformed 0.4 0.3 0.2 0.1 0.0 0 8000 16000 24000 32000 40000 48000 56000 640 8.0 8.8 9.6 10.4 11.2 12.0 12.8 13.6 14.4 15.2 16.0 V5 V4 Distinguishing 2 Populations Population 1 Population 2 The Result # of of of each each # # Height Are they different? What about these 2 Populations? Population 1 Population 2 The Result # of of of each each # # Height Are they different? Student’s t-Test • Also called the t-Test • Used to determine if 2 populations are different • Formally allows you to calculate the probability that 2 sample means are the same ≠ • Hypothesis testing: H0: µ 1 = µ 2; H1: µ 1 µ 2 • If the t-Test statistic gives you a p=0.4, and the α is 0.05, H0 is found to be true -> the 2 populations are the same
Details
-
File Typepdf
-
Upload Time-
-
Content LanguagesEnglish
-
Upload UserAnonymous/Not logged-in
-
File Pages117 Page
-
File Size-