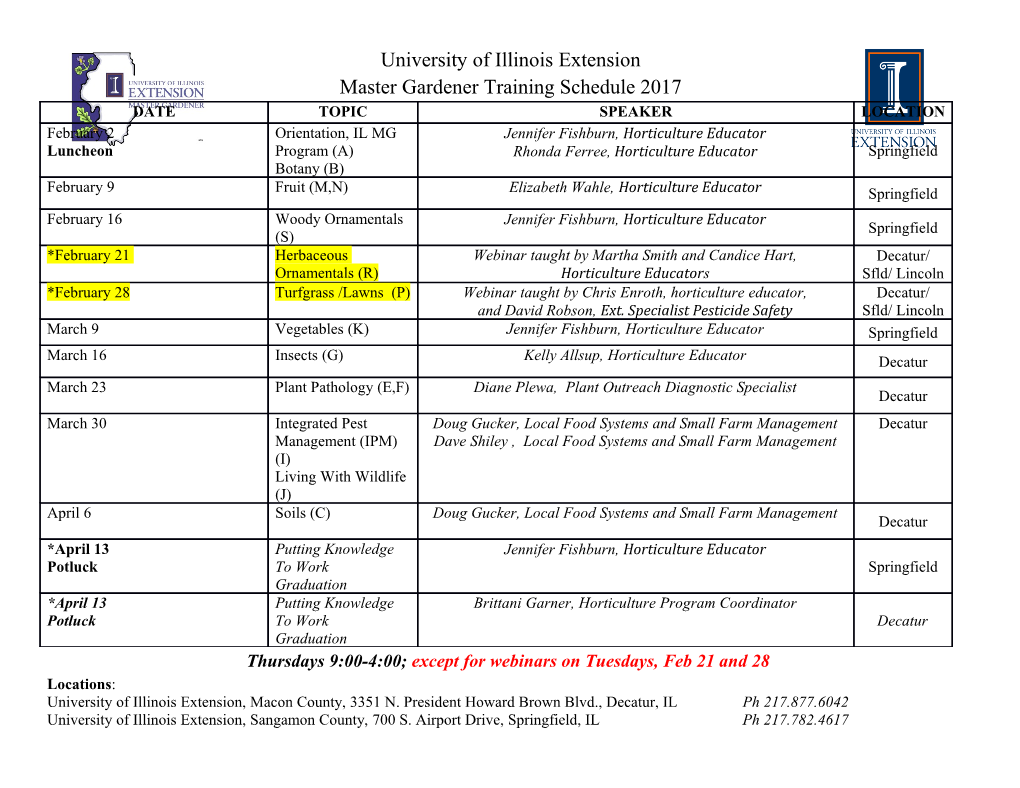
Copyright c 2008–2010 John Lafferty, Han Liu, and Larry Wasserman Do Not Distribute Chapter 36 Minimax Theory Minimax theory provides a rigorous framework for establishing the best pos- sible performance of a procedure under given assumptions. In this chapter we discuss several techniques for bounding the minimax risk of a statistical problem, including the Le Cam and Fano methods. 36.1 Introduction When solving a statistical learning problem, there are often many procedures to choose from. This leads to the following question: how can we tell if one statistical learning procedure is better than another? One answer is provided by minimax theory which is a set of techniques for finding the minimum, worst case behavior of a procedure. In this chapter we rely heavily on the following sources: Yu (2008), Tsybakov (2009) and van der Vaart (1998). 36.2 Definitions and Notation Let be a set of distributions and let X ,...,X be a sample from some distribution P 1 n P . Let ✓(P ) be some function of P . For example, ✓(P ) could be the mean of P , the 2P variance of P or the density of P . Let ✓ = ✓(X1,...,Xn) denote an estimator. Given a metric d, the minimax risk is b b Rn Rn( )=infsup EP [d(✓,✓(P ))] (36.1) ⌘ P ✓ P 2P where the infimum is over all estimators. Theb sample complexityb is n(✏, )=min n : R ( ) ✏ . (36.2) P n P n845 o 846 Chapter 36. Minimax Theory 36.3 Example. Suppose that = N(✓, 1) : ✓ R where N(✓, 1) denotes a Gaussian P { 2 } with mean ✓ and variance 1. Consider estimating ✓ with the metric d(a, b)=(a b)2. The − minimax risk is 2 Rn =infsup EP [(✓ ✓) ]. (36.4) ✓ P − 2P In this example, ✓ is a scalar. b b 2 36.5 Example. Let (X1,Y1),...,(Xn,Yn) be a sample from a distribution P . Let m(x)= EP (Y X = x)= ydP(y X = x) be the regression function. In this case, we might | | use the metric d(m ,m )= (m (x) m (x))2dx in which case the minimax risk is 1R 2 1 − 2 R 2 Rn =infsup EP (m(x) m(x)) . (36.6) m P − 2P Z In this example, ✓ is a function. b b 2 Notation. Recall that the Kullback-Leibler distance between two distributions P0 and P1 with densities p0 and p1 is defined to be dP p (x) KL(P ,P )= log 0 dP log 0 p (x)dx. 0 1 dP 0 p (x) 0 Z ✓ 1 ◆ Z ✓ 1 ◆ The appendix defines several other distances between probability distributions and explains how these distances are related. We write a b =min a, b and a b = max a, b . If ^ { } _ { } P is a distribution with density p, the product distribution for n iid observations is P n with n n n n density p (x)= i=1 p(xi). It is easy to check that KL(P0 ,P1 )=nKL(P0,P1). For positive sequences an and bn we write an = (bn) to mean that there exists C>0 such Q X that a Cb for all large n. a b if a /b is strictly bounded away from zero and n ≥ n n ⇣ n n n infinity for all large n; that is, an = O(bn) and bn = O(an). 36.3 Bounding the Minimax Risk The way we find Rn is to find an upper bound and a lower bound. To find an upper bound, let ✓ be any estimator. Then b Rn =infsup EP [d(✓,✓(P ))] sup EP [d(✓,✓(P ))] Un. (36.7) ✓ P P ⌘ 2P 2P b b So the maximum risk ofb any estimator provides an upper bound Un. Finding a lower bound Ln is harder. We will consider two methods: the Le Cam method and the Fano method. If ↵ the lower and upper bound are close, then we have succeeded. For example, if Ln = cn− ↵ and Un = Cn− for some positive constants c, C and ↵, then we have established that the ↵ minimax rate of convergence is n− . 36.4. Lower Bound Method 1: Le Cam 847 36.4 Lower Bound Method 1: Le Cam 36.8 Theorem. Let be a set of distributions. For any pair P0,P1 , P 2P ∆ n n ∆ nKL(P0,P1) inf sup EP [d(✓,✓(P ))] [p0 (x) p1 (x)]dx e− (36.9) ✓ P ≥ 4 ^ ≥ 8 2P Z where ∆=bd(✓(P0),✓(P1b)). Remark: The second inequality is useful, if KL(P0,P1)= , since it is usually difficult 1 to compute [pn(x) pn(x)]dx directly. An alternative is 0 ^ 1 R 2n n n 1 1 [p (x) p (x)]dx 1 p p . (36.10) 0 ^ 1 ≥ 2 − 2 | 0 − 1| Z ✓ Z ◆ 36.11 Corollary. Suppose there exist P0,P1 such that KL(P0,P1) log 2/n. Then 2P ∆ inf sup EP [d(✓,✓(P ))] (36.12) ✓ P ≥ 16 2P where ∆=d(✓(P0),✓(P1)). b b Proof. Let ✓0 = ✓(P0), ✓1 = ✓(P1) and ∆=d(✓0,✓1). First suppose that n =1so that we have a single observation X. An estimator ✓ defines a test statistic , namely, 1ifbd(✓,✓1) d(✓,✓0) (X)= ( 0ifd(✓,✓1) >d(✓,✓0). b b If P = P0 and =1then b b ∆=d(✓ ,✓ ) d(✓ , ✓)+d(✓ , ✓) d(✓ , ✓)+d(✓ , ✓)=2d(✓ , ✓) 0 1 0 1 0 0 0 and so d(✓ , ✓) ∆ . Hence 0 ≥ 2 b b b b b b ∆ ∆ EP0 [d(✓,✓0)] EP0 [d(✓,✓0)I( = 1)] EP0 [I( = 1)] = P0( = 1). (36.13) ≥ ≥ 2 2 Similarly, b b ∆ EP1 [d(✓,✓1)] P1( = 0). (36.14) ≥ 2 Taking the maximum of (36.13) and (36.14),b we have ∆ sup EP [d(✓,✓(P ))] max EP [d(✓,✓(P ))] max P0( = 1),P1( = 0) . P ≥ P P0,P1 ≥ 2 2P 2{ } n o b b 848 Chapter 36. Minimax Theory Taking the infimum over all estimators, we have ∆ inf sup EP [d(✓,✓(P ))] ⇡ ✓ P ≥ 2 2P where b b ⇡ =infmax Pj( = j). (36.15) j=0,1 6 Since a maximum is larger than an average, P0( = 0) + P1( = 1) ⇡ =infmax Pj( = j) inf 6 6 . j=0,1 6 ≥ 2 The sum of the errors P ( = 0) + P ( = 1) is minimized (see Lemma 36.16) by the 0 6 1 6 Neyman-Pearson test 0ifp (x) p (x) (x)= 0 ≥ 1 ⇤ 1ifp (x) <p (x). ⇢ 0 1 From Lemma 36.17, P0( = 0) + P1( = 1) 1 ⇤ 6 ⇤ 6 = [p (x) p (x)]dx. 2 2 0 ^ 1 Z Thus we have shown that ∆ inf sup EP [d(✓,✓(P ))] [p0(x) p1(x)]dx. ✓ P ≥ 4 ^ 2P Z b b n n Now suppose we have n observations. Then, replacing p0 and p1 with p0 (x)= i=1 p0(xi) n n and p (x)= p1(xi), we have 1 i=1 Q Q ∆ n n inf sup EP [d(✓,✓(P ))] [p0 (x) p1 (x)]dx. ✓ P ≥ 4 ^ 2P Z b In Lemma 36.18 below,b we show that p q 1 e KL(P,Q). Since KL(P n,Pn)= ^ ≥ 2 − 0 1 nKL(P ,P ), we have 0 1 R ∆ nKL(P0,P1) inf sup EP [d(✓,✓(P ))] e− . ✓ P ≥ 8 2P The result follows. b b 36.16 Lemma. Given P0 and P1, the sum of errors P0( = 1) + P1( = 0) is minimized over all tests by the Neyman-Pearson test 0ifp (x) p (x) (x)= 0 ≥ 1 ⇤ 1ifp (x) <p (x). ⇢ 0 1 36.4. Lower Bound Method 1: Le Cam 849 Proof. See exercise 2. 36.17 Lemma. For the Neyman-Pearson test , ⇤ P0( = 0) + P1( = 1) 1 ⇤ 6 ⇤ 6 = [p (x) p (x)]dx 2 2 0 ^ 1 Z Proof. See exercise 3. 1 KL(P,Q) 36.18 Lemma. For any P and Q, p q e− . ^ ≥ 2 R Proof. First note that max(p, q)+ min(p, q)=2. Hence R R 2 p q 2 p q p q = p q p q ^ ≥ − ^ ^ ^ _ Z Z Z Z Z 2 2 (p q)(p q) ppq =exp 2 log ppq ≥ ^ _ ≥ ✓ ◆ ✓ ◆ ✓ ◆ Z p Z Z q KL(P,Q) =exp 2 log p q/p exp 2 log p log = e− ≥ p ✓ ◆ ✓ ◆ Z p Z r where we used Jensen’s inequality in the last inequality. 36.19 Example. Consider data (X1,Y1),...,(Xn,Yn) where Xi Uniform(0, 1), Yi = ⇠ m(X )+✏ and ✏ N(0, 1). Assume that i i i ⇠ m = m : m(y) m(x) L x y , for all x, y [0, 1] . 2M ( | − | | − | 2 ) So is the set of distributions of the form p(x, y)=p(x)p(y x)=φ(y m(x)) where P | − m . 2M How well can we estimate m(x) at some point x? Without loss of generality, let’s take x =0so the parameter of interest is ✓ = m(0). Let d(✓ ,✓ )= ✓ ✓ . Let m (x)=0 0 1 | 0 − 1| 0 for all x. Let 0 ✏ 1 and define L(✏ x)0 x ✏ m (x)= 1 0 − x ✏. ⇢ ≥ 850 Chapter 36. Minimax Theory Then m ,m and ∆= m (0) m (0) = L✏. The KL distance is 0 1 2M | 1 − 0 | 1 p (x, y) KL(P ,P )= p (x, y) log 0 dydx 0 1 0 p (x, y) Z0 Z ✓ 1 ◆ 1 p (x)p (y x) = p (x)p (y x) log 0 0 | dydx 0 0 | p (x)p (y x) Z0 Z ✓ 1 1 | ◆ 1 φ(y) = φ(y) log dydx φ(y m (x)) Z0 Z ✓ − 1 ◆ ✏ φ(y) = φ(y) log dydx 0 φ(y m1(x)) Z ✏ Z ✓ − ◆ = KL(N(0, 1),N(m1(x), 1))dx.
Details
-
File Typepdf
-
Upload Time-
-
Content LanguagesEnglish
-
Upload UserAnonymous/Not logged-in
-
File Pages28 Page
-
File Size-