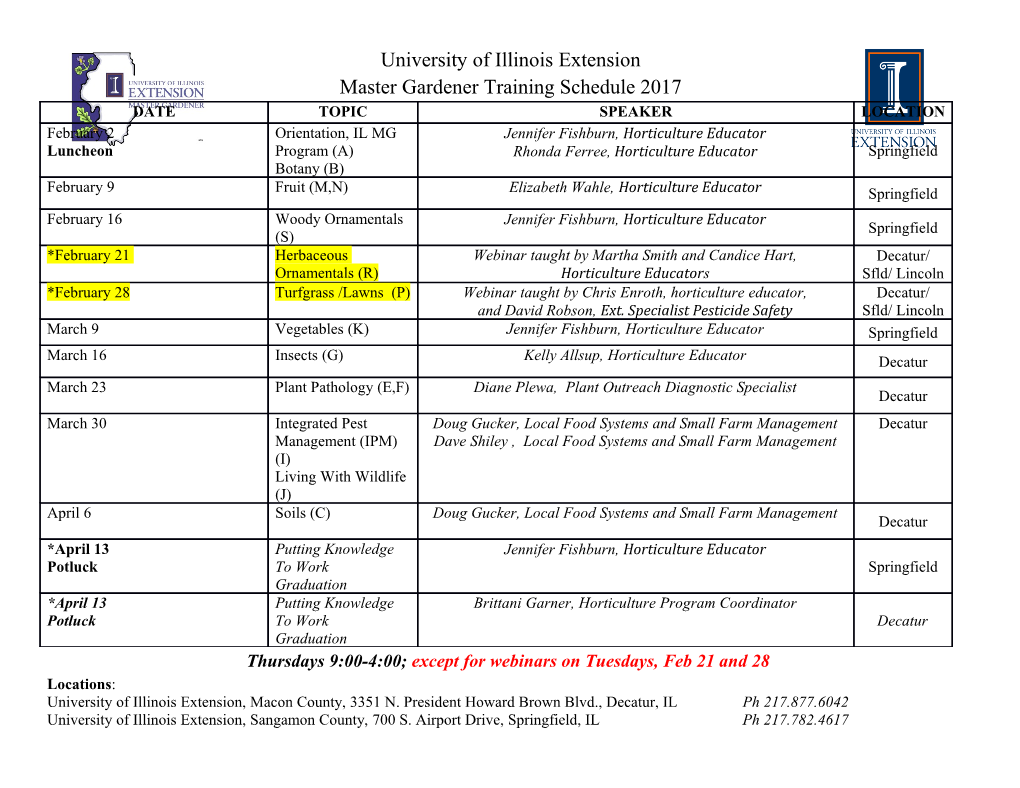
0 0 1 Matrix splitting B a2;1 0 C B C B . .. C A = diag (a1;1; a2;2; ··· ; an;n) + B . C B C @ an−1;1 an−1;2 ··· 0 A an;1 an;2 ··· an;n−1 0 0 1 0 a1;2 ··· a1;n−1 a1;n B 0 ··· a2;n−1 a2;n C B C B .. C + B . C B C @ 0 an−1;n A 0 0 1 0 1 0 1 B C B C B C def B C B C B C = D − L − U = B C − B C − B C : @ A @ A @ A x7.3 The Jacobi and Gauss-Siedel Iterative Techniques n×n I Problem: To solve Ax = b for A 2 R . I Methodology: Iteratively approximate solution x. No GEPP. 0 1 0 1 0 1 B C B C B C def B C B C B C = D − L − U = B C − B C − B C : @ A @ A @ A x7.3 The Jacobi and Gauss-Siedel Iterative Techniques n×n I Problem: To solve Ax = b for A 2 R . I Methodology: Iteratively approximate solution x. No GEPP. 0 0 1 Matrix splitting B a2;1 0 C B C B . .. C A = diag (a1;1; a2;2; ··· ; an;n) + B . C B C @ an−1;1 an−1;2 ··· 0 A an;1 an;2 ··· an;n−1 0 0 1 0 a1;2 ··· a1;n−1 a1;n B 0 ··· a2;n−1 a2;n C B C B .. C + B . C B C @ 0 an−1;n A 0 x7.3 The Jacobi and Gauss-Siedel Iterative Techniques n×n I Problem: To solve Ax = b for A 2 R . I Methodology: Iteratively approximate solution x. No GEPP. 0 0 1 Matrix splitting B a2;1 0 C B C B . .. C A = diag (a1;1; a2;2; ··· ; an;n) + B . C B C @ an−1;1 an−1;2 ··· 0 A an;1 an;2 ··· an;n−1 0 0 1 0 a1;2 ··· a1;n−1 a1;n B 0 ··· a2;n−1 a2;n C B C B .. C + B . C B C @ 0 an−1;n A 0 0 1 0 1 0 1 B C B C B C def B C B C B C = D − L − U = B C − B C − B C : @ A @ A @ A 0 10 −1 2 0 1 B −1 11 −1 3 C Ex: Matrix splitting for A = B C @ 2 −1 10 −1 A 0 3 −1 8 0 1 0 1 0 1 B C B C B C B C B C B C A = B C − B C − B C @ A @ A @ A 0 0 1 0 0 1 −2 0 1 B 1 0 C B 0 1 −3 C = diag (10; 11; 10; 8) − B C − B C @ −2 1 0 A @ 0 1 A 0 −3 1 0 0 Gauss-Siedel Method: Rewrite x = (D − L)−1 U x + (D − L)−1 b: Gauss-Siedel iteration with given x(0), x(k+1) = (D − L)−1 U x(k) + (D − L)−1 b; for k = 0; 1; 2; ··· : The Jacobi and Gauss-Siedel Methods for solving Ax = b Jacobi Method: With matrix splitting A = D − L − U, rewrite x = D−1 (L + U) x + D−1 b: Jacobi iteration with given x(0), x(k+1) = D−1 (L + U) x(k) + D−1 b; for k = 0; 1; 2; ··· : The Jacobi and Gauss-Siedel Methods for solving Ax = b Jacobi Method: With matrix splitting A = D − L − U, rewrite x = D−1 (L + U) x + D−1 b: Jacobi iteration with given x(0), x(k+1) = D−1 (L + U) x(k) + D−1 b; for k = 0; 1; 2; ··· : Gauss-Siedel Method: Rewrite x = (D − L)−1 U x + (D − L)−1 b: Gauss-Siedel iteration with given x(0), x(k+1) = (D − L)−1 U x(k) + (D − L)−1 b; for k = 0; 1; 2; ··· : Jacobi iteration with x(0) = 0, for k = 0; 1; 2; ··· (k+1) −1 (k) −1 xJ = D (L + U) xJ + D b 0 1 2 1 0 6 1 0 10 − 10 0 10 1 1 3 25 B 11 0 11 − 11 C (k) B 11 C = B 2 1 1 C xJ + B 11 C @ − 10 10 0 10 A @ − 10 A 3 1 15 0 − 8 8 0 8 Ex: Jacobi Method for Ax = b, with 0 10 −1 2 0 1 0 6 1 B −1 11 −1 3 C B 25 C A = B C ; b = B C @ 2 −1 10 −1 A @ −11 A 0 3 −1 8 15 A = D − L − U 0 0 1 0 0 1 −2 0 1 B 1 0 C B 0 1 −3 C = diag (10; 11; 10; 8) − B C − B C : @ −2 1 0 A @ 0 1 A 0 −3 1 0 0 Ex: Jacobi Method for Ax = b, with 0 10 −1 2 0 1 0 6 1 B −1 11 −1 3 C B 25 C A = B C ; b = B C @ 2 −1 10 −1 A @ −11 A 0 3 −1 8 15 A = D − L − U 0 0 1 0 0 1 −2 0 1 B 1 0 C B 0 1 −3 C = diag (10; 11; 10; 8) − B C − B C : @ −2 1 0 A @ 0 1 A 0 −3 1 0 0 Jacobi iteration with x(0) = 0, for k = 0; 1; 2; ··· (k+1) −1 (k) −1 xJ = D (L + U) xJ + D b 0 1 2 1 0 6 1 0 10 − 10 0 10 1 1 3 25 B 11 0 11 − 11 C (k) B 11 C = B 2 1 1 C xJ + B 11 C @ − 10 10 0 10 A @ − 10 A 3 1 15 0 − 8 8 0 8 Gauss-Siedel iteration with x(0) = 0, for k = 0; 1; 2; ··· (k+1) −1 −1 xGS = (D − L) U xGS + (D − L) b 0 10 1−1 00 0 1 −2 0 1 1 B −1 11 C BB 0 1 −3 C (k)C = B C BB C x C @ 2 −1 10 A @@ 0 1 A GS A 0 3 −1 8 0 0 6 1 10 25 B 11 C + B 11 C : @ − 10 A 15 8 Ex: Gauss-Siedel Method for Ax = b A = D − L − U 0 10 1 0 0 1 −2 0 1 B −1 11 C B 0 1 −3 C = B C − B C : @ 2 −1 10 A @ 0 1 A 0 3 −1 8 0 Ex: Gauss-Siedel Method for Ax = b A = D − L − U 0 10 1 0 0 1 −2 0 1 B −1 11 C B 0 1 −3 C = B C − B C : @ 2 −1 10 A @ 0 1 A 0 3 −1 8 0 Gauss-Siedel iteration with x(0) = 0, for k = 0; 1; 2; ··· (k+1) −1 −1 xGS = (D − L) U xGS + (D − L) b 0 10 1−1 00 0 1 −2 0 1 1 B −1 11 C BB 0 1 −3 C (k)C = B C BB C x C @ 2 −1 10 A @@ 0 1 A GS A 0 3 −1 8 0 0 6 1 10 25 B 11 C + B 11 C : @ − 10 A 15 8 0 1 1 B 2 C Jacobi vs. Gauss-Siedel: solution x = B C. @ −1 A 1 Convergence Comparision, Jacobi vs. G-S 10 0 Jacobi G-S 10 -2 10 -4 10 -6 10 -8 10 -10 10 -12 10 -14 10 -16 0 5 10 15 20 25 30 35 40 45 50 General Iteration Methods To solve A x = b with matrix splitting A = D − L − U, I Jacobi Method: (k+1) −1 (k) −1 xJ = D (L + U) xJ + D b: I Gauss-Siedel Method: (k+1) −1 (k) −1 xGS = (D − L) U xGS + (D − L) b: General Iteration Method: for k = 0; 1; 2; ··· x(k+1) = T x(k) + c: Next: convergence analysis on General Iteration Method Proof: Assume ρ(T ) < 1. Then (1) has unique solution x(∗). x(k+1) − x(∗) = T x(k) − x(∗) = T 2 x(k−1) − x(∗) = ··· = T k+1 x(0) − x(∗) =) 0: Conversely, if ··· (omitted) General Iteration: x(k+1) = T x(k) + c for k = 0; 1; 2; ··· Thm: The following statements are equivalent I ρ(T ) < 1. I The equation x = T x + c (1) has a unique solution and fx(k)g converges to this solution from any x(0). General Iteration: x(k+1) = T x(k) + c for k = 0; 1; 2; ··· Thm: The following statements are equivalent I ρ(T ) < 1. I The equation x = T x + c (1) has a unique solution and fx(k)g converges to this solution from any x(0). Proof: Assume ρ(T ) < 1. Then (1) has unique solution x(∗). x(k+1) − x(∗) = T x(k) − x(∗) = T 2 x(k−1) − x(∗) = ··· = T k+1 x(0) − x(∗) =) 0: Conversely, if ··· (omitted) Jacobi on random upper triangular matrix −1 I A = D − U. T = D U with ρ(T ) = 0: A is randn upper triangular with n = 50 0 5 10 15 20 25 30 35 40 45 50 0 10 20 30 40 50 nz = 1275 I Convergence plot G-S Convergence on upper triangular matrix 10 14 10 12 10 10 10 8 10 6 10 4 10 2 10 0 10 -2 10 -4 0 200 400 600 800 1000 1200 1400 1600 1800 2000 Taking difference of two equations, (D − ! L) x = ((1 − !) D + ! U) x + ! b: Successive Over-Relaxation (SOR), for k = 0; 1; 2; ··· (k+1) −1 (k) −1 xSOR = (D − ! L) ((1 − !) D + ! U) xSOR + ! (D − ! L) b def (k) = TSOR xSOR + cSOR: converges if ρ (TSOR) < 1.
Details
-
File Typepdf
-
Upload Time-
-
Content LanguagesEnglish
-
Upload UserAnonymous/Not logged-in
-
File Pages75 Page
-
File Size-