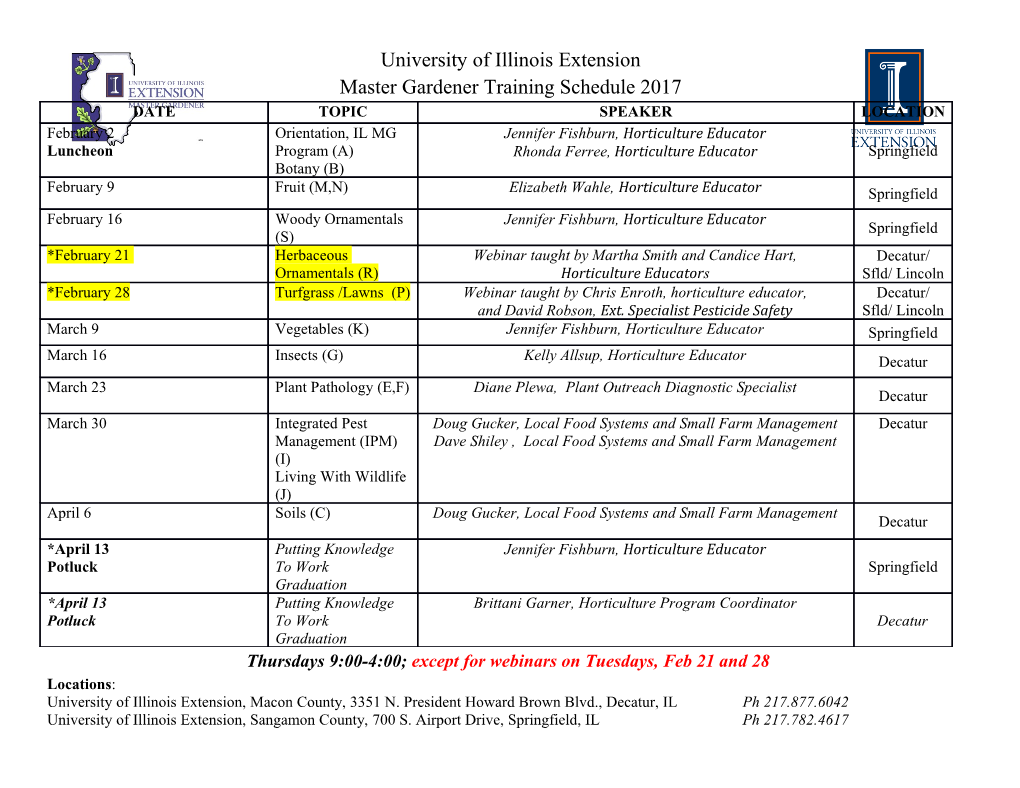
Generalized runs tests to detect randomness in hedge funds returns Rania Hentati-Kaffel, Philippe de Peretti SYRTO WORKING PAPER SERIES Working paper n. 17 | 2014 This project has received funding from the European Union’s Seventh Framework Programme for research, technological development and demonstration under grant agreement n° 320270. This documents reflects only the author's view. The European Union is not liable for any use that may be made of the information contained therein. Generalized Runs Tests to Detect Randomness in Hedge Funds Returns Rania Hentati-Kaffel Contact Author. Centre d’Economie de la Sorbonne (CES), 106-112 Boulevard de l’Hôpital, 75013 Paris, France. Tel: 0033144078763, mail: rania.kaff[email protected] Philippe de Peretti Centre d’Economie de la Sorbonne (CES), 106-112 Boulevard de l’Hôpital, 75013 Paris, France. Tel: 0033144078763, mail: [email protected] Abstract Using a large sample of hedge funds from the HFR universe over January 2000 to December 2012, we test and analyze the randomness, i.e. the lack of persistence, of absolute and relative returns using generalized runs tests. Our findings suggest that about 42% of the universe exhibit iid absolute returns, mainly found in proportions within the Macro and Equity Hedge strategies. A similar result holds for relative returns. Furthermore, funds having non-iid absolute or relative returns often exhibit ARCH effects and structural breaks. At last, using standard runs tests, a small percentage displays persistence in their relative performance (8.15% to 16.7% according to the benchmark), measured by their ability to produce clusters. Our work contributes to the hedge fund literature in terms of methodology, portfolio allocation, and performance measurement. Key words: Hedge Funds; Randomness; Persistence; Generalized Runs Tests JEL: G14; G23; C14 Preprint submitted to the Journal of Banking and Finance September 8, 2014 1. Introduction The recent financial crisis has stressed the crucial role of hedge funds returns analysis in the selection process of managers. Indeed, due to the well known opacity of hedge funds, limiting access to information on implemented positions and on strategies, past performances analysis to detect positive persistence is often one of the main quantitative tools helping investors to pick the right manager. Apart from investors, analyzing performance persistence is also of interest for economists trying to study the market efficiency as in Fama and MacBeth (1973). Performance persistence has been studied by many authors using para- metric and non-parametric methods. Following Boyson (2008), this literature beginning with the early studies of Sharpe (1966) and Jensen (1968), has re- turned contradictory results, and drawing clear-cut conclusions is uneasy. Thus whether or not hedge funds are able to produce persistence remains an open question (see Eling, 2009 for a survey of this vast literature). Indeed, whereas early studies support short-term but not long-term persistence (see e.g. Agarwal and Naik, 2000a, 2000b, Baquero, ter Horst and Verbeek, 2005, Brown, Goetzman and Park, 2001 or Gyger, Gibson and Bares (2003), late studies suggest that long-term persistence is also likely (see e.g. Fung et al., 2008, Jagannathan et al., 2007 or Kosowski et al., 2007). This literature has been extended in at least two ways. The first tries to link hedge fund performance persistence to fund characteristics (Amenc et al., 2003; Getmansky, 2012), whereas the other uses more advanced econo- metric tools, as the CPR (Agarwal and Naik, 2000a), the Chi-square test (Park and Staum, 1998, Carpenter and Lynch, 1999), the RIC (Herzberg and Mozes, 2003), the Kolmogorov-Smirnov goodness-of-fittest(Agarwal and Naik, 2000a) or the Hurst exponent as in De Souza and Gokcan (2004). 2 At last, recently, Kosowski, Naik and Teo (2007) have introduced a Bayesian approach to improve the accuracy of alpha estimates in parametric models. Our paper clearly relates to this second branch, and one of our major con- tributions is to analyze performance persistence using the newly introduced generalized runs tests of Cho and White (2011). Generalized runs tests are simple and powerful tests that allow to check for the null of randomness, i.e. lack of persistence, against a broad and undefined alternative including first and second-order dependence or structural breaks. To our knowledge, it is the first time that generalized runs tests are used on financial data. The second contribution of the paper is to implement these tests on a selected sample of hedge funds extracted from HFR database (4759 funds) over the period Janu- ary 2000 to December 2012. Both absolute and relative returns are analyzed. Concerning the latter, several benchmarks are used corresponding to either the hedge fund industry, or to an external market. Results are reported using a break down by primary strategies, i.e. Equity Hedge, Event-Driven, Macro and Relative Value. At last, since generalized runs tests are entirely based on the null, an incomplete mapping of the alternative is considered consisting in: i) AutoRegressive Conditional Heteroskedasticity (ARCH), ii) Structural breaks, and iii) Clustering, this latter being our measure of persistence. Our main findings are: i) About less than half of the sample exhibit iid returns. A similar figure holds for relative returns, regardless of the bench- mark used, ii) Under the alternative, most funds exhibit volatility clustering and/or structural breaks, whereas clustering, i.e. the ability to over-perform the market is found for between 8.15% and 16.7% of the funds, iii) In pro- portions, funds clustering are mainly found within the Relative Value and Event-Driven strategies, iv) Results are highly dependent on the benchmark and on the strategy, thus suggesting that the benchmark type (peer group 3 average versus traditional) is a crucial step in the investment process. Our work has therefore direct implications in terms of methodology, portfolio allocation, and performance measurement. This paper is structured as follows. In Section 2, we present the gener- alized runs tests. In Section 3, we implement the tests on HFR database. Section 4 goes deeper into the alternative, and Section 5 concludes and dis- cusses our results. 2. Generalized runs tests To analyze the randomness of absolute and relative returns we use Gener- alized Runs (GR) tests. Generalized runs tests have been introduced by Cho and White (2011) as a powerful mean to test the iid assumption against an unspecified broad alternative, including first and second order dependence or structural. This is a major difference with classical runs tests (Mood, 1940) in which the alternative is defined, i.e. clustering or mixing. Define rj T as a track record of absolute or relative returns of a hedge { it}t=1 j fund i having a strategy j computed as residuals of the linear model rit = oj oj h(Xt, θ),whereXt =(bt,rit ), bt is a benchmark of interest at time t, rit is the observed return, and θ is a parameter. The assumption we want to test j T j T is 0 : r is an iid sequence, against the broad alternative that r H { it}t=1 { it}t=1 is not an iid sequence. Let F (.) be the cumulative distribution function (cdf) of rj T ,andfirst assume that both θ and F (.) are perfectly known. Then { it}t=1 using the notation in Cho and White (2011), the runs are definedintwosteps. j First, for a given probability p build the set Tn(p)= t 1, 2, ..., n F (r ) < { ∈ { }| it p ,n=1, 2, ... that contains all indices such that the percentiles are less than } the probability p.LetMn(p) be the number of elements in the set. Now, sort by ascending order Tn(p),andlettn,r(p) be the element being at the rth 4 position of the sorted set, r =1, ..., Mn(p).Foragivenp,thep-runs Rn,r(p) are defined as follows: tn,r(p),r=1; R (p)= (1) n,r ⎧ tn,r(p) tn,r 1(p),r=2, ..., Mn(p). ⎨ − − To test for the null hypothesis⎩ and for a given s S compute the goodness- ∈ of-fit statistic Gn(p, s): M (p) 1 n sp G (p, s)= sRn,r(p) (2) n √n 1 s(1 p) r=1 − − − Various tests statistics are then derived by integrating Gn(p, s) over s for a given p; integrating over p for a given s; integrating over both p and s,or taking the supremum of the function for some p or s. p In this paper, among all these statistics, we focus on 1,n(S1)= Gn(p, s) ds, T S1 | | S1 =[ 0.99, 0.99] for p 0.1, 0.3, 0.5, 0.7, 0.9 , setting n = T .Testingfor − ∈ { } p the null then amounts to computing 1,n(S1) for various p andthencompar- T ing the computed values to critical values at a given threshold. Let pcv be the (5 1) vector of critical values at a given threshold for the various p, × p and let τ be the corresponding vector of 1,n(S1) statistics. Then, we fail to T reject the null if τ << pcv (critical values are reported by Cho and White, 2011). Now, if either F (.) or θ, or both are unknown, they are replaced by j 1 T their estimators F (rit)= T t=1 1(rj rj ) and θ. In this case, Empirical it≤ it Generalized Runs (EGR) tests are used in a similar fashion, replacing T (p)= n j j t 1, 2, ..., n F (r ) <p by Tn(p)= t 1, 2, ..., n F (r ) <p , tn,r(p) { ∈ { }| it } { ∈ { }| it } by t (p), M (p) by M (p). Thus, (1) and (2) are re-defined as: n,r n n tn,r(p),r=1; R (p)= (3) n,r ⎧ tn,r(p) tn,r 1(p),r=2, ..., Mn(p).
Details
-
File Typepdf
-
Upload Time-
-
Content LanguagesEnglish
-
Upload UserAnonymous/Not logged-in
-
File Pages24 Page
-
File Size-