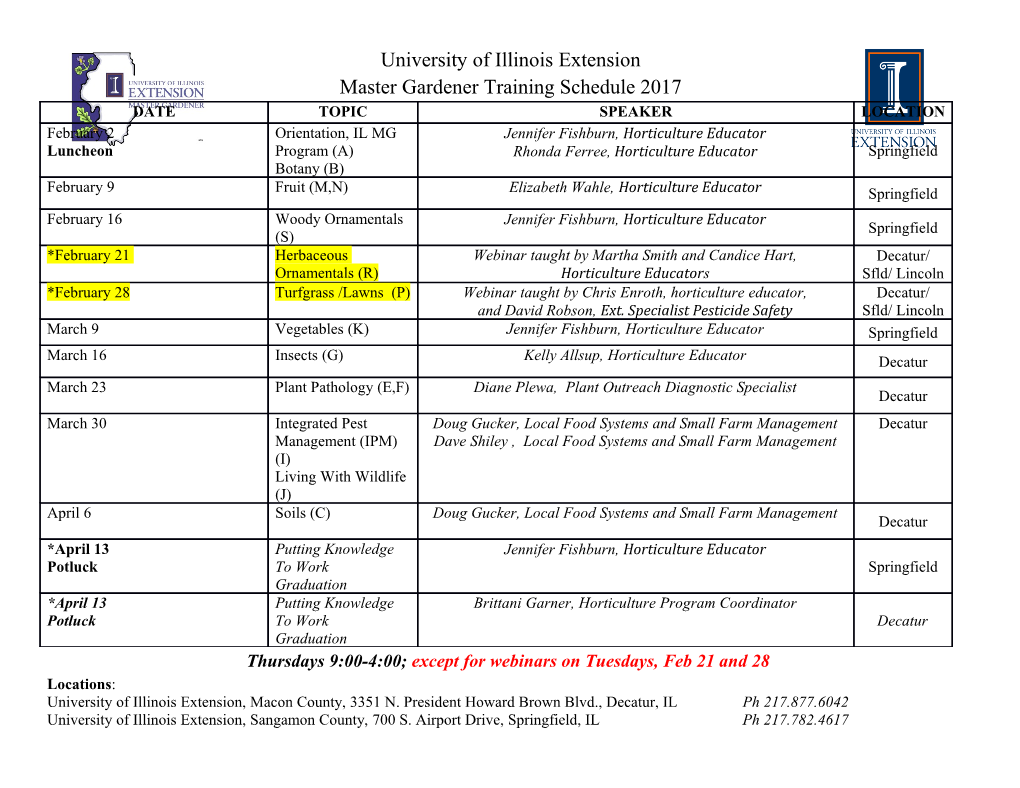
Asymptotic equivalence for inhomogeneous jump diffusion processes and white noise. Ester Mariucci To cite this version: Ester Mariucci. Asymptotic equivalence for inhomogeneous jump diffusion processes and white noise.. 2014. hal-00986607v1 HAL Id: hal-00986607 https://hal.archives-ouvertes.fr/hal-00986607v1 Preprint submitted on 2 May 2014 (v1), last revised 21 Mar 2015 (v2) HAL is a multi-disciplinary open access L’archive ouverte pluridisciplinaire HAL, est archive for the deposit and dissemination of sci- destinée au dépôt et à la diffusion de documents entific research documents, whether they are pub- scientifiques de niveau recherche, publiés ou non, lished or not. The documents may come from émanant des établissements d’enseignement et de teaching and research institutions in France or recherche français ou étrangers, des laboratoires abroad, or from public or private research centers. publics ou privés. ASYMPTOTIC EQUIVALENCE FOR INHOMOGENEOUS JUMP DIFFUSION PROCESSES AND WHITE NOISE. ESTER MARIUCCI Laboratoire Jean Kuntzmann, Grenoble. Abstract. We prove the global asymptotic equivalence between the experi- ments generated by the discrete (high frequency) or continuous observation of a path of a time inhomogeneous jump-diffusion process and a Gaussian white noise experiment. Here, the considered parameter is the drift function, and we suppose that the observation time T tends to ∞. The approximation is given in the sense of the Le Cam ∆-distance, under smoothness conditions on the unknown drift function. These asymptotic equivalences are established by constructing explicit Markov kernels that can be used to reproduce one experiment from the other. 1. Introduction Consider a one-dimensional time inhomogeneous jump-diffusion process Xt t 0 defined by { } ≥ t t Nt (1) Xt = f(s)ds + σ(s)dWs + Yi, t 0, 0 0 ≥ Z Z Xi=1 where: W = Wt t 0 is a standard Brownian motion; • { } ≥ N = Nt t 0 is an inhomogeneous Poisson process with intensity function • λ( ), independent{ } ≥ of W ; · (Yi)i 1 is a sequence of i.i.d. real random variables with distribution • G (either≥ concentrated on Z or absolutely continuous with respect to Lebesgue), independent of W and N; σ2( ) is supposed to be known and f( ) belongs to a certain non-parametric • class· F. · We suppose to observe Xt t 0 at discrete times 0 = t0 < t1 < < tn = Tn { } ≥ ··· such that ∆n = max1 i n ti ti 1 0 as n goes to infinity. We are interested ≤ ≤ | − − | ↓ n in estimating the drift function f( ) from the discrete data (Xti )i=1. At least two natural questions arise: · (1) How much information about the parameter f( ) do we lose by observing n · (Xti )i=1 instead of Xt t [0,Tn]? { } ∈ (2) Can we construct an easier (read: mathematically more tractable), but n equivalent, model from (Xti )i=1? E-mail address: [email protected]. Date: May 2, 2014. 1 2 ASYMPTOTIC EQUIVALENCE FOR ADDITIVE PROCESSES The aim of this paper is to give an answer to questions 1 and 2 by means of the Le Cam theory of statistical experiments. For the basic concepts and a detailed description of the notion of asymptotic equivalence that we shall adopt, we refer to [24, 25]. We recall the relevant definitions and properties in Section 2.2. The first asymptotic equivalence results for non-parametric experiments date to 1996 and are due to Brown and Low [3] and Nussbaum [29]. The interest of an equivalence in the Le Cam sense is that, when minimax rates of convergence in a non-parametric estimation problem are obtained in one experiment, the same rates automatically hold in any globally asymptotically equivalent experiment. Moreover, when explicit transformations from one experiment to another are available, statistical procedures can be carried over from one experiment to the other (see Remark 2.15). In the literature there is an abundance of works devoted to establishing as- ymptotic equivalence results for non-parametric experiments. In particular, as- ymptotic equivalence theory has been developed for non-parametric regression [3, 1, 21, 32, 8, 7, 30, 9, 27], non-parametric density estimation models [29, 6, 23, 2], generalized linear models [20], time series [22, 28], diffusion models [13, 18, 11, 12, 31, 17], GARCH model [5], functional linear regression [26] and spectral density estimation [19]. Negative results are somewhat harder to come by; the most notable among them are [15, 4, 35]. There is however a lack of equivalence results concerning processes with jumps. To our knowledge, this is the first one for what concerns the estimation of a drift function issued from a discretely (high frequency) observed Lévy process. We actually allow it to be inhomogeneous in time, i.e. an additive process. In this setting one should also cite the works [16, 14] as they are the only ones we know about treating (pure jumps) Lévy processes. However, they both give asymptotic results for the estimation of the Lévy measure. The interest in Lévy processes is due to them being a building block for sto- chastic continuous time models with jumps. Because of that, they are widely used in finance, queueing, telecommunications, extreme value theory, quantum theory or biology. Their stationarity property, however, makes them rather in- flexible; as a consequence, in recent years additive processes have been preferred in financial modelling (see [10], Chapter 14). It is therefore in this more general setting that we present our results. In order to mathematically reformulate questions 1 and 2, let us denote by D (f,σ2,λG) D (D, ) the Skorokhod space; define P as the law of Xt t [0,Tn] on (D, ) 2 { } ∈ (f,σ ,λG) Rn B Rn and Qn as the law of the vector (Xt1 ,...,Xtn ) on ( , ( )). Let us then consider the following statistical models, sharing the same param- eter set F: 2 2 P(f,σ ,λG) = D, D, (P (f,σ ,λG) : f F) , 2 2 ∈ Q(f,σ ,λG) = Rn, B(Rn), (Q(f,σ ,λG) : f F) . n n ∈ We state here our results under some general but not optimal conditions. For the precise statements see Section 2.4. Theorem 1.1. Suppose that F is a class of α-Hölder, uniformly bounded func- tions on R. Suppose also that σ( ) L (R) is bounded away from zero with · ∈ ∞ ASYMPTOTIC EQUIVALENCE FOR ADDITIVE PROCESSES 3 derivative σ′( ) in L (R) and λ( ) is in L (R). Then, ∞ ∞ 2 · 2 · 2 2 ∆ Q(f,σ ,λG), P(f,σ ,0) 0 and ∆ P(f,σ ,λG), Q(f,σ ,λG) 0 as n , n → n → → ∞ under either of the following two sets of conditions: (1) Y is discrete with support on Z, α 1 and T ∆ 0 as n ; in 1 ≥ 2 n n → → ∞ this case the rate of convergence is O(√Tn∆n). (2) Y admits a density with respect to the Lebesgue measure on R, α 1 1 ≥ 4 and Tn√∆n 0 as n ; in this case the rate of convergence is 1 1 2 4 → → ∞ O Tn ∆n . The paper is organized as follows. Sections 2.1 to 2.3 fix assumptions and notation. The main results, as well as examples, are given in Section 2.4. A dis- cussion of the results can be found in Section 2.5. The proofs are postponed to Section 3. They are obtained as a sequence of results proving different (asymp- totic) equivalences. Loosely speaking, we firstly reduce to having in each interval of the discretization at most one jump (Bernoulli approximation, Section 3.1). Secondly, we filter it out via an explicit Markov kernel, reducing ourselves to treating independent Gaussian variables (Section 3.2). Finally, we apply an ar- gument similar to that in [3] (Section 3.3) and collect all the pieces to conclude the proofs in Section 3.4. 2. Assumptions and main results 2.1. Additive processes. Time inhomogeneous jump-diffusion processes are a special case of additive processes. Here we briefly recall definitions and properties of this class of processes. Definition 2.1. A stochastic process Xt t 0 on R defined on a probability space (Ω, A , P) is an additive process if{ the} following≥ conditions are satisfied. (1) X0 = 0 P-a.s. (2) Independent increments: for any choice of n 1 and 0 t0 < t1 < . < t , random variables X , X X ,...,X ≥ X are≤ independent. n t0 t1 − t0 tn − tn−1 (3) There is Ω0 A with P(Ω0) = 1 such that, for every ω Ω0, Xt(ω) is right-continuous∈ in t 0 and has left limits in t > 0. ∈ (4) Stochastic continuity:≥ ε > 0, P( X X ε) 0 as h 0. ∀ | t+h − t| ≥ → → Thanks to the Lévy-Khintchine formula (see [10], Theorem 14.1), the charac- teristic function of any additive process X = Xt t [0,T ] can be expressed, for all u in R, as: { } ∈ (2) t u2 t E iuXt 2 iuy I e = exp iu f(r)dr σ (r)dr (1 e + iuy y 1)νt(dy) , 0 − 2 0 − R − | |≤ Z Z Z where f( ) and σ2( ) belongs to L (R) and ν is a positive measure on R satisfying · · 1 t 2 νt( 0 ) = 0 and (y 1)νt(dy) < , t [0,T ]. { } R ∧ ∞ ∀ ∈ Z 2 In the sequel we shall refer to (f(t), σ (t), νt)t [0,T ] as the local characteristics of ∈ the process X and a νt as above will be called a Lévy measure, for all t. This data characterizes uniquely the law of the process X. In the case where f( ) and · 4 ASYMPTOTIC EQUIVALENCE FOR ADDITIVE PROCESSES σ( ) are constant functions and νt = ν for all t, the process X satisfying (2) is stationary,· and is called a Lévy process of characteristic triplet (f, σ2, ν).
Details
-
File Typepdf
-
Upload Time-
-
Content LanguagesEnglish
-
Upload UserAnonymous/Not logged-in
-
File Pages17 Page
-
File Size-