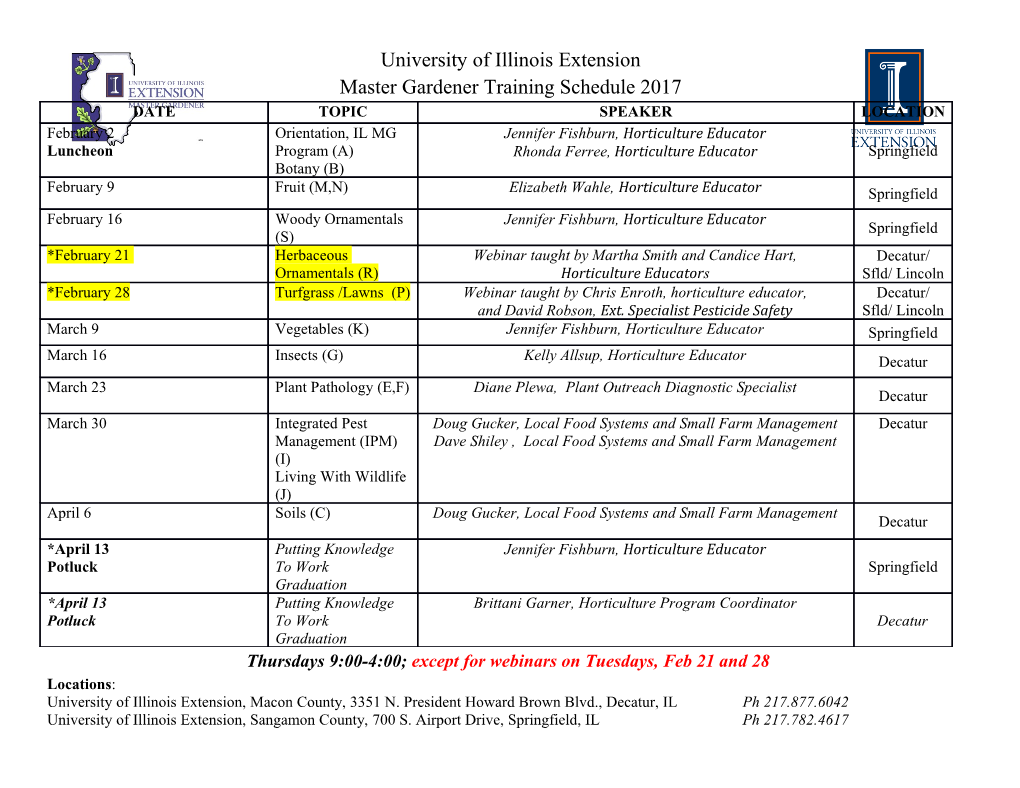
Combining Structured and Free-text Electronic Medical Record Data for Real-time Clinical Decision Support Emilia Apostolova1, Tony Wang2, Ioannis Koutroulis3, Tim Tschampel4, Tom Velez4 1 Language.ai, Chicago, IL [email protected] 2 Imedacs, Ann Arbor, MI [email protected] 3 Children’s National Health System, Washington, DC [email protected] 4 Computer Technology Associates, Ridgecrest, CA [email protected], [email protected] Abstract clinical notes (e.g. nursing notes, radiology re- ports, etc.), diagnosis and procedure codes, vital The goal of this work is to utilize Electronic signs, lab orders and results. The challenge of Medical Record (EMR) data for real-time Clinical Decision Support (CDS). We present real-time CDS systems is the variability and the a deep learning approach to combining in real availability of real-time EMR data, resulting from time available diagnosis codes (ICD codes) different charting behaviors, health care delivery and free-text notes: Patient Context Vectors. models, hospital settings, etc. Patient Context Vectors are created by averag- The goal of this work is to utilize all available ing ICD code embeddings, and by predicting EMR patient information for real-time predictive the same from free-text notes via a Convolu- modelling. While our experiments are focused on tional Neural Network. The Patient Context Vectors were then simply appended to avail- identifying ARDS cases, the described method is able structured data (vital signs and lab results) applicable to a variety of use cases needing infor- to build prediction models for a specific condi- mation dispersed across the EMR patient record. tion. Experiments on predicting ARDS, a rare The primary contribution of this work is the use and complex condition, demonstrate the utility of low-dimensional representation of the patient’s of Patient Context Vectors as a means of sum- history, current symptoms and conditions, which marizing the patient history and overall condi- we refer to as Patient Context Vector. At pre- tion, and improve significantly the prediction diction time, Patient Context Vectors are gener- model results. ated from the combination of available up-to-date 1 Introduction ICD codes (if any) and available nursing notes. A key goal in critical care medicine is the early Patient Context Vectors (vectors of real numbers) identification and timely treatment of rapidly pro- are then simply added to the list of existing struc- gressive, life-threatening conditions, such as Sep- tured data variables (vital signs and lab results) sis, Septic Shock, and Acute Respiratory Distress and used to identify patients at risk of developing Syndrome (ARDS). Such life-threatening condi- life-threatening conditions that require rapid inter- tions, are both rare, and at the same time, com- vention. plex and heterogeneous, involving the interaction 2 Method of multiple risk factors, comorbidities, and current In this work, we combine ICD codes, clinical symptoms. Hospital alert systems typically rely notes, vital signs, lab results, and demographic in- on screening of structured data such as vital signs formation to build a real-time ARDS prediction and lab results, and, in the case of such rare condi- model. Low-dimensional representation of ICD tions, are often associated with “alert fatigue” and codes (ICD embeddings) is generated from a large require manually entered clinical judgement. corpus of patient ICD records. Patient visit EMR The information needed for a reliable risk eval- data is used to look up recorded up-to-date ICD uation of such rare and complex conditions is typi- codes, clinical notes, vital signs, and lab results. cally dispersed across the patient EMR, and avail- The visit ICD codes are converted to embeddings able at different times throughout the patient stay. and averaged to produce Patient Context Vectors. The patient demographics, past medical and visit Pertinent patient information might not be nec- history, chronic conditions, risk factors, current essarily ”ICD-coded” during prediction time, but signs and symptoms can be found in the form of 66 Proceedings of the BioNLP 2019 workshop, pages 66–70 Florence, Italy, August 1, 2019. c 2019 Association for Computational Linguistics can be available in the form of nursing notes. A ICD10 codes), they tend to be interdependent, deep learning model was trained to predict the pa- and to co-occur. For example, Pneumonia ICD tient’s Patient Context Vector from nursing notes. codes are often accompanied with ICD codes The Patient Context Vectors obtained from avail- describing Cough, Fever, Pleural effusion, etc. able in the system ICD codes, and from free-text Inspired by word embeddings (Mikolov et al., notes are then used in conjunction with vital signs, 2013), it has been suggested that this medical code and lab results to predict the patient’s outcome. co-occurence can be exploited to generate low- Details for each step of the approach are provided dimensional representations of ICD codes: ICD in subsequent sections. Embeddings (Choi et al., 2016b,a; Kartchner et al., 2.1 Dataset 2017). We utilized the freely available database com- All available MIMIC3 patient data was used to prising deidentified health-related data associated generate the ICD embeddings following the ap- with over 40,000 patients who stayed in critical proach of (Choi et al., 2016b). In our approach, we care units of the Beth Israel Deaconess Medical attempted to generate a low-dimensional represen- Center between 2001 and 2012: the MIMIC3 In- tation of the patient history, symptoms, risk fac- tensive Care Unit (ICU) database (Johnson et al., tors, diagnosis, etc, by averaging the patient ICD 2016). The dataset contains over 2 million free- code embeddings (creating Patient Context Vec- text clinical notes and over 650,000 diagnosis tors). The optimum size of the vectors was deter- codes for over 58,000 visits. Included ICUs are mined to be 50. medical, surgical, trauma-surgical, coronary, and 2.3 Predicting Patient Context Vectors from cardiac surgery recovery units. EMR data includes Clinical Texts vital signs, laboratory results, diagnosis codes, While averaged ICD embeddings appear to be a free text nursing notes, radiology reports, medi- useful summary of the overall patient history, con- cations, discharge summaries, treatments, etc. dition, symptoms, and risk factors, ICD code data 2.2 ICD Embeddings and Patient Context is not necessarily available for real-time CDS sys- Vectors tems. Some ICD codes associated with patients’ Clinicians viewing properly coded patient di- history and symptoms might be entered early on agnosis codes (ICD9 and ICD10 codes1) are typ- in the EMR system. However, diagnosis ICD ically capable of deducing the overall condition, codes are typically obtained after tests and lab re- history, and risk factors associated with a patient. sults and might not be available during prediction Intuitively, the totality of patient’s diagnosis codes time. Similarly, not all relevant patient history and represent a meaningful medical summary of the symptoms are necessarily ICD-coded. patient. Diagnosis codes are used to describe At the same time, nursing notes typically con- both current diagnoses (e.g. Community-acquired tain all currently available information, even if not Pneumonia ), but also a variety of additional facts. present in the form of ICD codes. Nursing notes For example, ICD codes can describe patient’s his- include information such as past medical history, tory and chronic conditions (e.g. Chronic kid- reason for visit, current symptoms, summary of ney disease; Personal history of traumatic frac- test outcomes, etc. ture; etc.); information regarding past and current In order to capture information present in free- treatments and procedures (e.g. Infection due to text notes, we also built a word-level CNN model other bariatric procedure). In some cases, ICD that predicts the patient Patient Context Vector codes contain information such as the patient age from the note text. The model was trained group (e.g. Sepsis of newborn; Elderly multi- on available nursing and discharge notes and gravida); expected outcome (Encounter for pal- achieved a mean squared error of 0.179 on the val- liative care); patient’s social history (e.g. Adult idation set. The network was trained on 1,081,176 emotional/psychological abuse); the reason for the free-text notes, with pre-trained word-embeddings visit, (e.g. Railway accidents; Motor Vehicle acci- of size 100. The texts were truncated/padded to dents, etc). the 90th percentile length (785 tokens). The net- While there are a large number of ICD codes work consists of a Convolutional, Max Pooling (around 15,000 ICD9 codes and around 68,000 layers, followed by 2 hidden layers of size 500. 1 The International Classification of Diseases, c The World Health Orga- The last layer uses linear activation with loss func- nization. 67 tion of mean squared error to predict the Patient GBM 2 Features AUC P R F1 Context Vector . Baseline 90.42 41.76 67.80 51.68 2.4 Patient Context Vectors in Prediction Baseline + ICD 93.30 53.02 68.44 59.75 Models Patient Context Vector Baseline + Notes 91.88 48.25 64.25 55.11 In order to test the utility of the Patient Con- Patient Context Vector text Vectors for predicting patient outcomes, we Baseline + first 93.59 56.35 66.52 61.01 focused on building a real-time ARDS prediction half of notes/ICD model. ARDS is a rare and life-threatening condi- DRF Features AUC P R F1 tion that require an early intervention (Fan et al., Baseline 89.14 38.58 66.43 48.81 2017). Baseline + ICD 92.08 51.87 63.75 57.20 ARDS patients were limited to adult patients Patient Context Vector Baseline + Notes 91.18 47.89 62.11 54.08 only (age 18 or older). The patients inclusion cri- Patient Context Vector teria consist of the presence of acute respiratory Baseline + first 92.61 57.02 61.08 58.98 failure and continuous mechanical ventilation, ex- half of notes/ICD cluding patients with acute exacerbation of asthma Table 1: 10-fold cross-validation GBM and DRF results of predicting ARDS patients.
Details
-
File Typepdf
-
Upload Time-
-
Content LanguagesEnglish
-
Upload UserAnonymous/Not logged-in
-
File Pages5 Page
-
File Size-