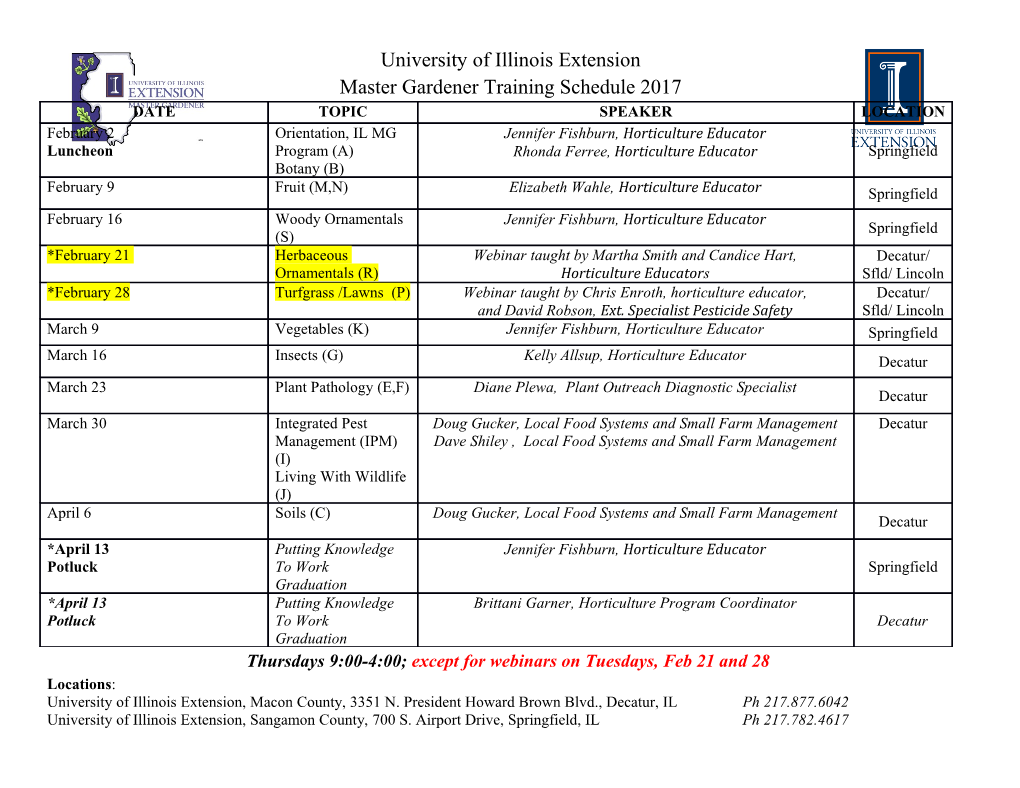
Mathematical Biosciences and Engineering, 16(4): 2942–2958. DOI: 10.3934/mbe.2019145 Received: 03 January 2019 Accepted: 26 February 2019 Published: 10 April 2019 http://www.aimspress.com/journal/MBE Research article The identification of a common different gene expression signature in patients with colorectal cancer Zhongwei Zhao1#, Xiaoxi Fan1#, Lili Yang2#, Jingjing Song1, Shiji Fang1, Jianfei Tu1, Minjiang Chen1, Liyun Zheng1, Fazong Wu1, Dengke Zhang1, Xihui Ying1 and Jiansong Ji1,* 1 Department of Interventional Radiology, Zhejiang University Lishui Hospital, The Fifth Affiliated Hospital of Wenzhou Medical University, Lishui Central Hospital, Lishui 323000, China 2 Department of Anesthesiology, Zhejiang University Lishui Hospital, The Fifth Affiliated Hospital of Wenzhou Medical University, Lishui Central Hospital, Lishui 323000, China #These authors contributed equally to this study. *Correspondence: Email: [email protected]; Tel: +86-13857088508. Abstract: Colorectal cancer (CRC) is one of the most common malignancies, giving rise to serious financial burden globally. This study was designed to explore the potential mechanisms implicated with CRC and identify some key biomarkers. CRC-associated gene expression dataset (GSE32323) was downloaded from GEO database. The differentially expressed genes (DEGs) were selected out based on the GEO2R tool. Gene ontology (GO) and Kyoto Encyclopedia of Genes and Genomes (KEGG) analyses were employed to search the enriched pathways of these DEGs. Additionally, a protein-protein interaction (PPI) network was also constructed to visualize interactions between these DEGs. Quantitative Real-time PCR (qPCR) was further performed to valid the top5 up-regulated and top5 down-regulated genes in patients with CRC. Finally, the survival analysis of the top5 up-regulated and top5 down-regulated genes was conducted using GEPIA, aiming to clarify their potential effects on CRC. In this study, a total of 451 DEGs were captured (306 down-regulated genes and 145 up-regulated genes). Among these DEGs, the top5 up-regulated genes were DPEP1, KRT23, CLDN1, LGR5 and FOXQ1 while the top5 down-regulated genes were CLCA4, ZG16, SLC4A4, ADH1B and GCG. GO analysis revealed that these DEGs were mainly enriched in cell adhesion, cell proliferation, RNA polymerase II promoter and chemokine activity. KEGG analysis disclosed that the enriched pathway included mineral absorption, chemokine signaling pathway, transcriptional misregulation in cancer, pathways in cancer and PPAR signaling pathway. Survival analysis showed that the expression level of ZG16 may correlate with the prognosis of CRC patients. 2943 Furthermore, according to the connectivity degree of these DEGs, we selected out the top15 hub genes, namely MYC, CXCR1, TOP2A, CXCL12, SST, TIMP1, SPP1, PPBP, CDK1, THBS1, CXCL1, PYY, LPAR1, BMP2 and MMP3, which were expected to be promising therapeutic target in CRC. Collectively, our analysis unveiled potential biomarkers and candidate targets in CRC, which could be helpful to the diagnosis and treatment of CRC. Keywords: colorectal cancer; biomarkers; bioinformatics analysis; differentially expressed genes (DEGs); Quantitative Real-time PCR (qPCR) 1. Introduction Colorectal cancer (CRC) is one of the leading causes of death associated with cancer worldwide, which has been estimated to cause 50,000 deaths per year in America [1,2]. The pathogenesis of CRC is a complicated process involved in mutations of various oncogenes and tumor suppressor genes that pinpoint multiple cellular events, such as endoplasmic reticulum stress [3], oxidative stress [4], epithelial-mesenchymal transition (EMT) [5], abnormal cell proliferation and apoptosis [6]. Although surgery combined with adjuvant radiotherapy or chemotherapy has effectively improved the prognosis of CRC, metastasis or neoplasm recurrence still occur in more than 50% of the CRC patients who have received a definite diagnosis or underwent intended curative treatment, contributing to a relatively poor 5-year survival rate [7]. Additionally, it is difficult for doctors to achieve early diagnosis of CRC for the reason that specificity of auxiliary examination indices is lacking in clinic, so that many patients may eventually miss the optional chances for surgery, thereby increasing the risk of death [8]. Hence, the detection of early-stage biomarkers and identification of core therapeutic target appear significant to decrease CRC-related deaths. Recent years, the high-throughput gene microarray has been widely used to screen the differentially expressed genes (DEGs) between normal samples and tumor samples in human beings and animal models, which makes it accessible for us to further explore the entire molecular alterations of tumors at multiple levels involving DNA, RNA, proteins, epigenetic alterations, and metabolism [9,10]. However, it still has a long way to go to put these microarrays in application in clinic because the DEGs identified by gene profiling were countless and the statistical analyses were also too complicated [11]. Therefore, it is urgent to verify a proper number of genes and develop a suitable approach which can be operated by routine assay in clinic. In this study, we selected the GSE32323 from Gene Expression Omnibus (GEO) and applied bioinformatics analysis to screen the DEGs in CRC. Subsequently, we used STRING to construct the protein-protein interaction (PPI) network to identify the hub genes with top15 degree of connectivity in CRC. Moreover, we made the analysis of these DEGs, including biological process (BP), molecular function (MF), cellular component (CC) and KEGG pathways. We chose top5 up-regulated and top5 down-regulated DEGs to make the overall survival (OS) analysis, aiming to observe the associations between the DEGs and the prognosis of CRC. Finally, we confirmed the mRNA expression level of the top5 up-regulated and top5 down-regulated DEGs by Q-PCR in samples obtained from patients with CRC in our hospital. These genes will assist us to screen and identify significant biomarkers and therapeutic targets of CRC in the near future. Mathematical Biosciences and Engineering Volume 16, Issue 4, 2942–2958. 2944 2. Materials and method 2.1. Data source The heading levels should not be more than 4 levels. The fond of heading and subheadings should be 12 point normal Times New Roman. The first letter of headings and subheadings should be capitalized. The gene expression profile of GSE32323 was downloaded from the GEO database, which was a free and publicly available database. 17 pairs of cancer and non-cancerous tissues from patients with CRC in this dataset were detected by Affymetrix HG-U133 Plus 2.0 arrays by Ahmed K [12]. We also downloaded the raw Series Matrix File of GSE32323 from the GEO database. 2.2. DEGs analysis In our study, the online software GEO2R was employed to analyze the tissue samples in GSE32323 dataset. GEO2R is an online software by which users can divide the samples into two and more groups and select out the DEGs. We used the Benjamini and Hochberg methods by default to discover false rate and used the adjust P value to reduce the errors of false positive. The choice criterion contains the adjust P value < 0.05 and |logFC| ≥ 2. 2.3. Gene ontology and KEGG pathway analysis of DEGs Gene ontology analysis (GO), serving as a useful approach to annotate genes and gene products, could also identify characteristic biological attributing to high-throughput genome or transcriptome data. Kyoto Encyclopedia of Genes and Genomes (KEGG) is a collection of databases, aiming to handle biological pathways, genomes, diseases, chemical substances and drugs. We divided these DEGs into up-regulated group and down-regulated group. The Database for Annotation, Visualization and Integrated Discovery (DAVID, https://david.ncifcrf.gov/), an online web based on the bioinformatics, is routinely applied for annotating genes and protein function [13]. We input these DEGs separately into DAVID to acquire the GO and KEGG analysis, P < 0.05 as choice criterion. Finally, we used the ImageGP (http://www.ehbio.com/ImageGP/index.php/Home/Index/index.html) to visualize the major biological processes (BP), molecular function (MF) and cell composition (CC) of these DEGs. 2.4. Comparison of the top5 upregulated and top5 downregulated DEGs GEPIA (http://gepia.cancer-pku.cn/index.html), designed by Chenwei Li, Zefang Tang, and Boxi Kang of Zhang Lab, Peking University, is a newly developed interactive web server aiming at analyzing the RNA sequencing expression data of 9736 tumors and 8587 normal samples from the GTEx and TCGA projects in a standard processing pipeline [14]. In this study, we employed the boxplot to visualize the mRNA expression of top5 up-regulated and top5 down-regulated DEGs in CRC tissues and and normal colorectum tissues. Transcripts per million (TPM) is the number of transcripts from this particular gene normalized first by gene length, and then by sequencing depth (in millions) in the sample. GEPIA also provides the transcripts per million (TPM) of these DEGs to display their relative expression levels. Mathematical Biosciences and Engineering Volume 16, Issue 4, 2942–2958. 2945 2.5. Comparison of the top5 upregulated and top5 downregulated DEGs The overall survival (OS) of the top5 upregulated and top5 downregulated DEGs Similarly, we used the GEPIA database to get the overall survival information of these DEGs. The logrank P value and hazard ratio (HR) with 95% confidence intervals were showed on the plot. P <
Details
-
File Typepdf
-
Upload Time-
-
Content LanguagesEnglish
-
Upload UserAnonymous/Not logged-in
-
File Pages17 Page
-
File Size-