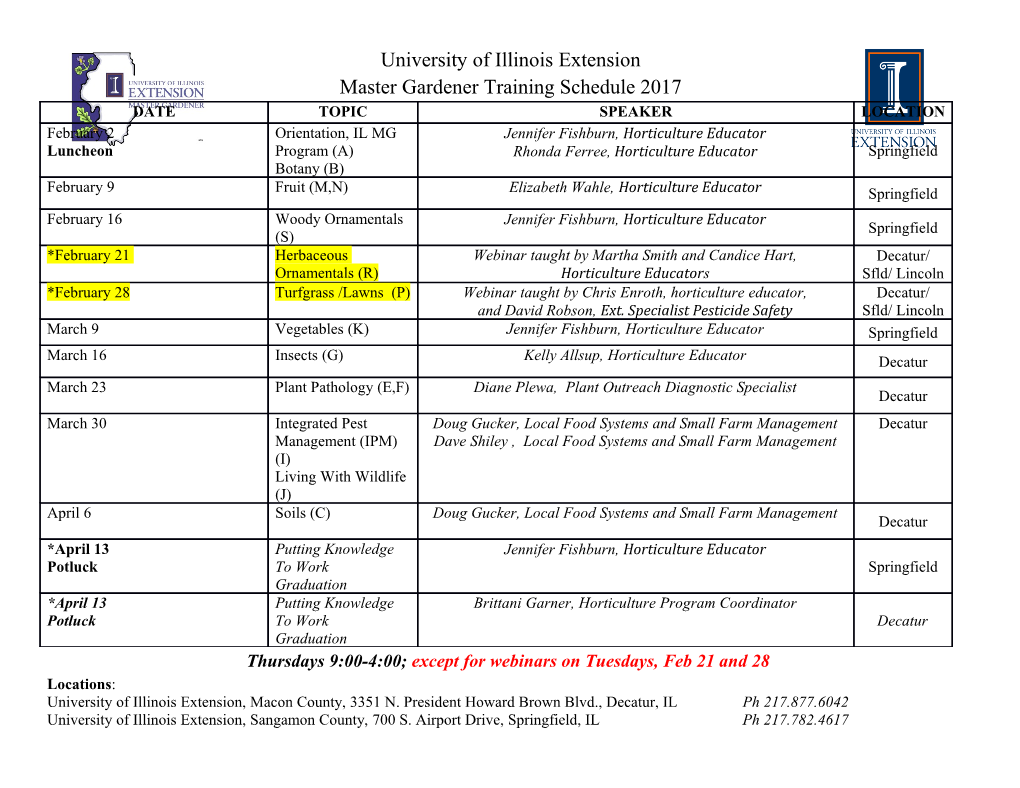
remote sensing Article Estimating Rainfall with Multi-Resource Data over East Asia Based on Machine Learning Yushan Zhang 1 , Kun Wu 1,2*, Jinglin Zhang 3, Feng Zhang 2,4 , Haixia Xiao 5,6, Fuchang Wang 4,7 , Jianyin Zhou 8, Yi Song 9 and Liang Peng 9 1 Department of Atmospheric Sciences, Nanjing University of Information Science & Technology, Nanjing 210044, China; [email protected] 2 Shanghai Qi Zhi Institute, Shanghai 200232, China; [email protected] 3 Department of Computer and Software, Nanjing University of Information Science & Technology, Nanjing 210044, China; [email protected] 4 Department of Atmospheric and Oceanic Sciences, Institute of Atmospheric Sciences, Fudan University, Shanghai 200438, China; [email protected] 5 Nanjing Joint Institute for Atmospheric Sciences, Nanjing 210008, China; [email protected] 6 Key Laboratory of Transportation Meteorology, China Meteorological Administration, Nanjing 210008, China 7 Shanghai Typhoon Institute of China Meteorological Administration, Shanghai 200030, China 8 College of Meteorology and Oceanography, National University of Defense Technology, Changsha 410073, China; [email protected] 9 Meteorological Remote Sensing Application Center, Beijing Piesat Information Technology Co., Ltd., Beijing 100195, China; [email protected] (Y.S.); [email protected] (L.P.) * Correspondence: [email protected]; Tel.: +86-151-9590-0108 Abstract: The lack of accurate estimation of intense precipitation is a universal limitation in pre- cipitation retrieval. Therefore, a new rainfall retrieval technique based on the Random Forest (RF) algorithm is presented using the Advanced Himawari Imager-8 (Himawari-8/AHI) infrared spec- Citation: Zhang, Y.; Wu, K.; Zhang, J.; trum data and the NCEP operational Global Forecast System (GFS) forecast information. And the Zhang, F.; Xiao, H. Wang, F.; Zhou, J.; gauge-calibrated rainfall estimates from the Global Precipitation Measurement (GPM) product served Song, Y.; Peng, L. Estimating Rainfall as the ground truth to train the model. The two-step RF classification model was established for with Multi-Resource Data over East (1) rain area delineation and (2) precipitation grades’ estimation to improve the accuracy of moderate Asia Based on Machine Learning. rain and heavy rain. In view of the imbalance categories’ distribution in the datasets, the resampling Remote Sens. 2021, 13, 3332. https:// technique including the Random Under-sampling algorithm and Synthetic Minority Over-sampling doi.org/10.3390/rs13163332 Technique (SMOTE) was implemented throughout the whole training process to fully learn the characteristics among the samples. Among the features used, the contributions of meteorological Academic Editor: Kenji Nakamura variables to the trained models were generally greater than those of infrared information; in particular, Received: 19 July 2021 the contribution of precipitable water was the largest, indicating the sufficient necessity of water Accepted: 19 August 2021 vapor conditions in rainfall forecasting. The simulation results by the RF model were compared Published: 23 August 2021 with the GPM product pixel-by-pixel. To prove the universality of the model, we used independent validation sets which are not used for training and two independent testing sets with different Publisher’s Note: MDPI stays neutral periods from the training set. In addition, the algorithm was validated against independent rain with regard to jurisdictional claims in gauge data and compared with GFS model rainfall. Consequently, the RF model identified rainfall published maps and institutional affil- areas with a Probability Of Detection (POD) of around 0.77 and a False-Alarm Ratio (FAR) of around iations. 0.23 for validation, as well as a POD of 0.60–0.70 and a FAR of around 0.30 for testing. To estimate precipitation grades, the value of classification was 0.70 in validation and in testing the accuracy was 0.60 despite a certain overestimation. In summary, the performance on the validation and test data indicated the great adaptability and superiority of the RF algorithm in rainfall retrieval in East Copyright: © 2021 by the authors. Asia. To a certain extent, our study provides a meaningful range division and powerful guidance for Licensee MDPI, Basel, Switzerland. quantitative precipitation estimation. This article is an open access article distributed under the terms and Keywords: Himawari-8 satellite; numerical product; rainfall retrieval; random forest; precipita- conditions of the Creative Commons tion grades Attribution (CC BY) license (https:// creativecommons.org/licenses/by/ 4.0/). Remote Sens. 2021, 13, 3332. https://doi.org/10.3390/rs13163332 https://www.mdpi.com/journal/remotesensing Remote Sens. 2021, 13, 3332 2 of 29 1. Introduction Precipitation is one of the most important indicators that reflects global and regional cli- mate system changes. In particular, summer precipitation plays a vital role in atmospheric circulation, hydrological cycle, and thermal momentum exchange [1–3]. The interdecadal variation of precipitation is tightly linked to the circulation anomaly, water vapor budget, soil humidity, and wind speeds in East Asia [4,5]. High-resolution rainfall data have an extensive application in the fields of agriculture, forestry, transportation, and marine mon- itoring [6–9]. There are obvious weaknesses in traditional ground station measurement, due to the limited coverage of rain gauges, which is confined by the complex terrain and coastline, and the uncertainty of accuracy in the weather radar detection [10]. Contrastingly, satellites can make comprehensive observations and provide intuitive remote sensing im- age information; therefore, satellite rainfall products are superior and have good prospects to improve the ability of monitoring grid rainfall [11]. Satellite rainfall products are re- trieved by microwave remote sensing, visible/infrared remote sensing, and multisensor rainfall estimation, with microwave sensors mainly carried by polar-orbiting satellites, such as NOAA, METOP, and FY-3. Although the detecting precision of polar-orbiting satellites is higher than geostationary satellites, the time sampling is low. Fortunately, since the new generation of geostationary meteorological satellites (such as GOES, MSG, Himawari-8, and FY-4) has been launched, visible/infrared remote sensing can provide high spatiotemporal resolution rainfall products. However, the rainfall accuracy still needs to be improved as a result of the indirect relationship between the infrared signal and precipitation [12–14]. Multi-channels retrieval overcomes many shortcomings of the past and has become the mainstream of high-quality rainfall products’ retrieval, including the Tropical Rainfall Measuring Mission (TRMM), Precipitation Estimation from Remotely Sensed Information using Artificial Neural Networks (PERSIANN), and the Climate Prediction Center Morph- ing Method (CMORPH), which have been used in the accuracy assessment of precipitation at different time scales [15–17]. Global Precipitation Measurement (GPM), as the successor of TRMM, has been equipped with more advanced passive microwave radiometer GMI and dual-frequency radar DPR sensors, enhancing the skill of observing solid and trace precipitation and providing a more accurate calibration reference for multisatellite precipi- tation estimation [18,19]. The Final-Run products have been corrected by the monthly scale of the ground station data, and the accuracy is closest to the real precipitation, which can be well studied and applied to climate change, drought identification, flood forecasting, and so on [20,21]. It is gratifying that the high-resolution infrared data can match the GPM products well in both time and space, which provides a favorable condition for rainfall retrieval systems [22,23]. Currently, many studies have established forecasting algorithms using real-time geo- stationary Infrared (IR) satellite data to retrieve high-resolution rainfall products based on the infrared–precipitation relationship. At an early stage, the single-channel infrared data were considered due to technical limitations. Arkin [24] found that there was a high correla- tion between the cold cloud coverage area and the 6 h precipitation accumulation in a large enough area where the cloud top temperature was lower than 225 K to 255 K in the GARP Atlantic Tropical Experiment (GATE). From this discovery, Arkin et al. [25,26] proposed a rainfall estimation algorithm, the GOES Precipitation Index (GPI); the application of the GPI requires a large enough spatial and temporal scale; however, with the improvement of resolution, the accuracy of the satellite-based estimation of small-scale precipitation is limited [27]. Todd et al. [28] combined the instantaneous value of passive remote sensing with frequent infrared images and introduced the MIRA algorithm to optimize the relation- ship between infrared and precipitation by using the probability matching method; they further reduced the spatial and temporal scale of precipitation estimation. Adler et al. [29] proposed the “inside to outside” Convective-Stratiform Technology (CST), which took the minimum value of the local brightness temperature as the convective precipitation center, allocated the precipitation rate and precipitation area to the surrounding points, Remote Sens. 2021, 13, 3332 3 of 29 and used the brightness temperature threshold value of thunderstorm anvils to determine the stratified precipitation. Grecu et al. [30] combined
Details
-
File Typepdf
-
Upload Time-
-
Content LanguagesEnglish
-
Upload UserAnonymous/Not logged-in
-
File Pages29 Page
-
File Size-