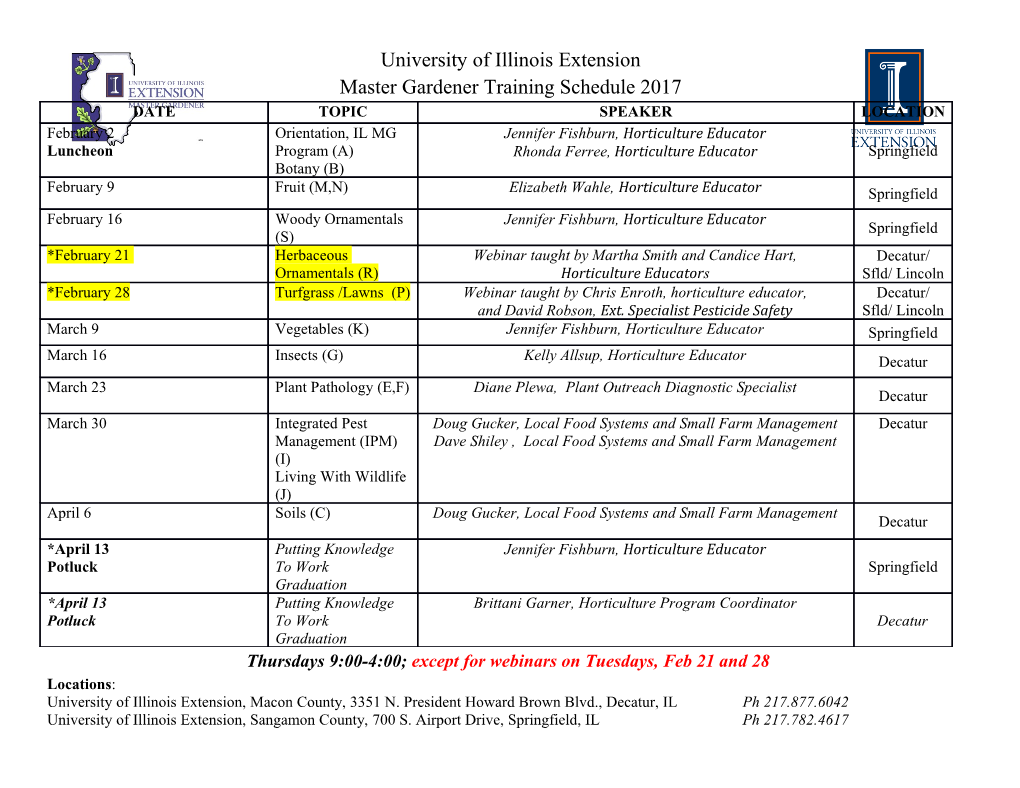
Time Series: Co-integration Series: Economic Forecasting; Time Series: General; Watson M W 1994 Vector autoregressions and co-integration. Time Series: Nonstationary Distributions and Unit In: Engle R F, McFadden D L (eds.) Handbook of Econo- Roots; Time Series: Seasonal Adjustment metrics Vol. IV. Elsevier, The Netherlands N. H. Chan Bibliography Banerjee A, Dolado J J, Galbraith J W, Hendry D F 1993 Co- Integration, Error Correction, and the Econometric Analysis of Non-stationary Data. Oxford University Press, Oxford, UK Time Series: Cycles Box G E P, Tiao G C 1977 A canonical analysis of multiple time series. Biometrika 64: 355–65 Time series data in economics and other fields of social Chan N H, Tsay R S 1996 On the use of canonical correlation science often exhibit cyclical behavior. For example, analysis in testing common trends. In: Lee J C, Johnson W O, aggregate retail sales are high in November and Zellner A (eds.) Modelling and Prediction: Honoring December and follow a seasonal cycle; voter regis- S. Geisser. Springer-Verlag, New York, pp. 364–77 trations are high before each presidential election and Chan N H, Wei C Z 1988 Limiting distributions of least squares follow an election cycle; and aggregate macro- estimates of unstable autoregressive processes. Annals of Statistics 16: 367–401 economic activity falls into recession every six to eight Engle R F, Granger C W J 1987 Cointegration and error years and follows a business cycle. In spite of this correction: Representation, estimation, and testing. Econo- cyclicality, these series are not perfectly predictable, metrica 55: 251–76 and the cycles are not strictly periodic. That is, each Engle R F, Granger C W J 1991 Long-run Economic Relations: cycle is unique. Quite generally, it is useful to think of Readings in Co-integration. Oxford University Press, Oxford, time series as realizations of stochastic processes, and UK this raises the question of how to represent cyclicality Hiraki T, Shiraishi N, Takezwa N 1996 Cointegration, common in stochastic processes. The major descriptive tool for factors, and term structure of Yen offshore interest rates. this is the ‘spectral density function’ (or ‘spectrum’), Journal of Fixed Income December: 69–75 which is the subject of this article. Johansen S 1988 Statistical analysis of co-integration vectors. Journal of Economic Dynamics and Control 12: 231–54 Johansen S 1995 Likelihood-based Inference in Cointegrated Vector Autoregressie Models. Oxford University Press, Oxford, UK 1. Cycles in a Typical Time Series Johansen S 1996 Likelihood-based inference for co-integration Figure 1 shows a time series plot of new housing of some nonstationary time series. In: Cox D R, Hinkley D V, authorizations (‘building permits’) issued by com- Barndorff-Nielsen O E (eds.) Time Series Models: In Econo- munities in the USA, monthly, from 1960 through metrics, Finance and Other Fields. Chapman and Hall, New 1999. This plot has characteristics that are typical of York Lee C M C, Myers J, Swaminathan B 1999 What is the intrinsic many economic time series. First, the plot shows a value of the Dow? Journal of Finance 54: 1693–741 clear seasonal pattern: permits are low in the late fall Lien D, Tse Y K 1999 Forecasting the Nikkei spot index with and early winter, and rise markedly in the spring and fractional co-integration. Journal of Forecasting 18: 259–73 summer. This seasonal pattern is persistent through- Lu$ tkepohl H 1993 Introduction to Multiple Time Series Analysis. out the sample period, but does not repeat itself 2nd edn. Springer-Verlag, New York exactly year-after-year. In addition to a seasonal Maddala G S, Kim I M 1998 Unit Roots, Cointegration, and pattern, there is a slow moving change in the level of Structural Change. Cambridge University Press, Cambridge, the series associated with the business cycle. For UK example, local minima of the series are evident in 1967, Park J Y 1992 Canonical co-integrating regressions. Econo- the mid-1970s, the early 1980s, and in 1990, which metrica 60: 119–43 correspond to periods of macroeconomic slowdown Phillips P C B 1991 Optimal inference in co-integrated systems. or recession in the US economy. Econometrica 59: 283–306 How can the cyclical variability in the series be Reinsel G C, Ahn S K 1992 Vector AR models with unit roots represented? One approach is to use a periodic and reduced rank structure: estimation, likelihood ratio test, and forecasting. Journal of Time Series Analysis 13: 353–75 function like a sine or cosine function. But this is Stock J H 1994 Unit roots, structural breaks and trends. In: inadequate in at least two ways. First, a deterministic Engle R F, McFadden D L (eds.) Handbook of Econometrics, periodic function doesn’t capture the randomness in Vol. IV. Elsevier, Netherlands the series. Second, since several different periodicities Stock J H, Watson M W 1988 Testing for common trends. (seasonal, business cycle) are evident in the plot, Journal of the American Statistical Association 83: 1097–107 several periodic functions will be required. The next Tanaka K 1996 Time Series Analysis: Nonstationary and section presents a representation of a stochastic Noninertibel Distribution Theory. Wiley, New York process that has these ingredients. 15714 Time Series: Cycles Figure 1 Building permits in the USA 2. Representing Cyclical Behaior in Time Series: and troughs. Finally, the process is covariance station- l λ l σ# ω The Spectrum ary with E(Yt) 0 and k cos( k). Adding together several components like Eqn. 1 produces a more interesting stochastic process: 2.1 Spectral Representation of a Coariance Stationary Process n l α ω jδ ω Consider a sequence of scalar random variables Yt [ j cos( jt) j sin( jt)] (2) oY q where t l 0, p1, p2,…, and E(Y ) l µ, j=" t l λ t Co (YtYt−k) k. That is, assume that Yt is observed at regular intervals, like a week, a month, a year, etc. with E(α ) l E(δ ) l 0, for all j; E(α δ ) l 0, for all j, k; α α lj δ δ j l j k α# l δ# l Assume that the first and second moments of the E( j k) E( j k) 0, for j k; and E( j ) E( j ) µ λ σ# process do not depend on time, that is and k are time j . For this process, Yt is the sum of n different invariant. To simplify notation, assume that µ l 0. uncorrelated components, each corresponding to a To motivate the spectral representation of Y ,itis different frequency, and each with its own variance. t α ω jδ ω σ# useful to begin with a very special and simple stoch- (The variance of j cos( jt) j sin( jt)is j .) A l λ l n σ# ω astic process: calculation shows E(Yt) 0, k j=" j cos( jk), and the variance of Y is given by ar (Y ) l n σ#. l α ω jδ ω t t j=" j Yt cos( t) sin( t) (1) So far, this has all been special. That is, two very special processes have been constructed. However, a where α and δ are random variables with E(α) l E(δ) fundamental result (Crame! r 1942, Kolmorgorov 1940) l 0; E(αδ) l 0; and E(α#) l E(δ#) l σ#. While simple, shows that a generalization of this decomposition can this process has three attractive characteristics. First, be used to represent any covariance stationary process. it is periodic: since cos(aj2π) l cos(a) and sin (aj2π) The result, known as the ‘Spectral Representation l sin(a), then Y l Y for QjQ l 1, 2,… . So Y t+j#π/ω t t Theorem,’ says that if Yt is covariance stationary, then repeats itself with a period of 2π\ω. Second, the it can be represented as random components α and δ give Y a random amplitude (value of at its peak) and a random phase π π (value at t l 0). Thus, two realizations of Y will have l ω α ω j ω δ ω Yt & cos( t)d ( ) & sin( t)d ( ) different amplitudes and different timing of their peaks ! ! 15715 Time Series: Cycles where dα(ω) and dδ(ω) are zero mean random vari- The spectrum can be determining by inverting this ables that are mutually uncorrelated, uncorrelated relation: across frequency, and have variances that depend on frequency. Like the special example shown in Eqn. 2, _ ω l π −" λ −iωj this general representation decomposes Yt into a set of S( ) (2 ) je (5) strictly periodic components—each uncorrelated with j=−_ the others, and each with its own variance. For example, processes with important seasonal com- (To verify that Eqn. 5 is the inverse of Eqn. 4, use ponents will have large values of the components corresponding to the seasonal frequency, processes for π 1 π l 5 iωk 2 2 for k 0 6 & e dω l 3 7 (6) series with strong ‘business cycle’ components will 0 for k 0 have large variances at business cycle frequencies, etc. −π 4 8 Sometimes the spectral representation is written π −"o!π _ λ −iω(j−k) ωqlλ using complex numbers as: so that (2 ) −π j=−_ je d k.) π A simpler formula for the spectrum is Y l & eiωtdγ(ω) (3) t _ π − S(ω) l (2π)−"[λ j2 λ cos( jω)]. (7) N ! j where dγ(ω) l (1\ 2)[dα(ω)kidδ(ω)] for ω & 0 and j=" dγ(ω) l dγ(kω) for ω ! 0.
Details
-
File Typepdf
-
Upload Time-
-
Content LanguagesEnglish
-
Upload UserAnonymous/Not logged-in
-
File Pages8 Page
-
File Size-