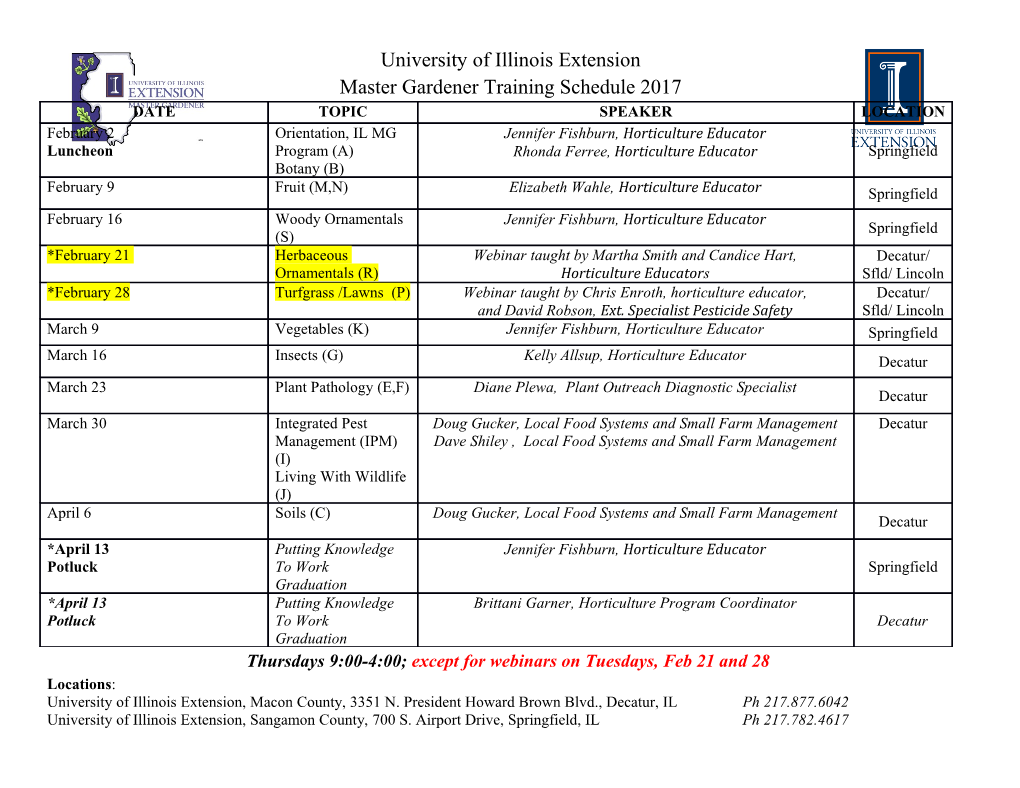
Proceedings, APSIPA Annual Summit and Conference 2018 12-15 November 2018, Hawaii Hypercomplex Principal Component Pursuit via Convex Optimization Takehiko Mizoguchi∗y and Isao Yamada∗ ∗Department of Information and Communications Engineering, Tokyo Institute of Technology, Tokyo, Japan yNEC Corporation, Kanagawa, Japan E-mail: fmizoguchi,[email protected] Abstract—Expressing multidimensional information as a value color, material, intensity, etc., and each attribute may have in hypercomplex number systems (e.g., quaternion, octonion, etc.) correlation with other attributes. Modeling of the correlations has great potential, in signal processing applications, to enjoy among attributes in multidimensional data and will be more their nontrivial algebraic benefits which are not available in standard real or complex vector systems. Exploiting sparsity of and more important by the popularization of 3D printer [11], hypercomplex matrices or vectors would play an important role virtual reality, medical imaging etc. Algebraically natural oper- in utilizing such benefits. In this paper, we propose a new spar- ations in hypercomplex number system has great potential for sity measure for evaluating sparsity of hypercomplex matrices. such modelingof various correlations (see e.g., [12]–[16] for With utilizing this measure, the hypercomplex robust principal color image processing applications). However, because of the component analysis can be relaxed into the hypercomplex robust principal component pursuit and it can be reduced to a real “singularity” of higher dimensional C-D number systems (see convex optimization problem. We then derive an algorithmic e.g., Example 1), few mathematical tools had been maintained solution to the hypercomplex principal component pursuit based [17]–[20]. To overcome this situation, in our previous works on a proximal splitting technique. Numerical experiments are [21]–[23], we have proposed several useful mathematical tools performed in the octonion domain and show that the proposed for designing advanced algorithm for optimization, learning algorithm outperforms a part-wise state-of-art real, complex and quaternion algorithm. and low rank approximation in hypercomplex domain. In [21] we proposed an algebraic real translation for clarifying the I. INTRODUCTION relation between C-D linear system and real vector valued Multidimensional information arises naturally in many areas linear systems, and successfully designed some online learning of engineering and science since almost all observations have algorithms which are available in general C-D domain. More- many attributes. Utilizing hypercomplex number system for over, we also proposed in [22], [23] useful tools C-D singular representing such multidimensional information is one of the value decomposition, R-rank (see Section II-C) and low rank most effective ways because we can express multidimensional approximation technique and proposed an algorithmic solution information not in terms of vectors but in terms of num- for low rank hypercomplex tensor completion. bers among which we can define the four basic arithmetic One of the other standard approximation methods utilizing operators. Indeed, it has been used in many areas such as simpler structure of matrices is the robust principal component computer graphics [1] and robotics [2], [3] wind forecasting analysis (RPCA) [24], which separates an input matrix into a [4]–[6] and noise reduction in acoustic systems [7]. In the low-rank and sparse ones: statistical signal processing field, effective utilization of the k k m-dimensional Cayley-Dickson number system (C-D number minimize rank(L) + λ S 0 s.t. M = L + S; (1) L;S2RM×N system) [8], [9], which is a standard class of hypercomplex ∥·∥ number systems [10], including, e.g., real R, complex C, where 0 denotes the `0-norm, which counts the number quaternion H, octonion O and sedenion S etc., have been of non-zero entries of S. The RPCA has been successfully investigated. used for various signal processing applications such as source A hypercomplex number has one real part and many imag- separation [25], face recognition [26] and so on. Unfortunately, inary parts, and it can represent multidimensional data as a the formulation (1) is NP-hard, so its convex relaxation called number for which the four arithmetic operations including the principal component pursuit [24] is often considered in multiplication and division are available. It can fulfill the stead of the RPCA. Recently, the PCP is extended to simpler four arithmetic operation for multidimensional information, hypercomplex domains C and H using the complex matrix which are not available for ordinary real multidimensional isomorphism [27]. However, it can be only applied up to four vectors. Moreover, thanks to the nontrivial algebraic struc- dimensional data and hard to be generalized to octonion and ture, the multiplication of hypercomplex numbers can enjoy higher dimensional C-D domain only with this isomorphism. algebraically interactions among real and imaginary parts. In this paper, to establish a RPCA framework in hyper- Hypercomplex vectors, matrices and tensors can also enjoy complex domain, first, we present Cayley-Dickson principal these benefits. For example, in 3D object modeling, each point component analysis (C-D PCP) as a convex relaxation of the in 3-dimensional space can have multiple attribute such as C-D extension of RPCA. This relaxation is based on the 978-988-14768-5-2 ©2018 APSIPA 579 APSIPA-ASC 2018 Proceedings, APSIPA Annual Summit and Conference 2018 12-15 November 2018, Hawaii R-rank proposed in [22] and a new sparsity measure, `1- m-dimensional hypercomplex number Am is extended to A2m norm of C-D matrices. This sparsity measure can be inter- [8], [9] as preted as evaluating a group sparsity of real matrices, so the z := x + yi 2 A ; x; y 2 A ; proximity operator can be easily calculated. Hence, the C-D m+1 2m m PCP is a convex optimization in real domain which can be where im+1 62 Am is the additional imaginary unit for dou- A 2 − solved by applying proximal splitting techniques to a certain bling the dimension of m satisfying im+1 = 1, i1im+1 = structured convex optimization problem. We finally propose im+1i1 = im+1 and ivim+1 = −im+1iv =: im+v for all A an hypercomplex PCP algorithm m based on Douglas- v = 2; : : : ; m. For example, the real number system (A1 :=) R Rachford splitting (DRS) [28]. The proposed algorithm is a is extended into complex number system C (= A2) by the C-D C-D generalization of a PCP algorithm (DR-PCP) proposed construction. Note that the value of m is restricted to the form in [29] and can be applied to general C-D domains. of 2n (n 2 N). The hypercomplex number systems constructed Numerical experiments are performed in the context of inductively from the real number by the C-D construction are recovering sparsely corrupted low rank matrices in octonion called Cayley-Dickson number system (C-D number system). domain and show that the proposed algorithm successfully uti- The imaginary units appeared in the C-D number systems 2 − lizes algebraically natural correlations of real and all imaginary have many characteristic properties [21] such as iα = 1 − 6 2 f g parts to recover much more faithfully the original matrices, and iαiβ = iβiα(α = β)Pfor all α; β 2; : : : ; m . These corrupted randomly by noise, than a part-wise real, complex ∗ m 2 ≥ 2 A properties ensures aa = `=1 a` 0 for any a m in ∗ and quaternion PCP algorithms. (2) and a 2 Am in (3) and enablep us to define the absolute j j ∗ II. PRELIMINARIES values of C-D number a as a := aa . A. Hypercomplex Number System Example 1. 1) A representative example of hypercomplex Let N and R be respectively the set of all non-negative inte- number is the quaternion H. The quaternion number gers and the set of all real numbers. Define an m-dimensional system is constructed from the complex number system hypercomplex number Am (m 2 N n f0g) expanded on the by using the C-D construction. A quaternion number is real vector space [8] a 4-dimensional hypercomplex which is defined as 2 H 2 R a := a1i1 + a2i2 + ··· + amim 2 Am; a1; : : : ; am 2 R (2) q = q1 + q2{ + q3| + q4κ ; q1; q2; q3; q4 based on imaginary units i1;:::; im, where i1 = 1 represents with the multiplication table: the vector identity element. Any hypercomplex number is {| = −|{ = κ, ȷκ = −κȷ = {; κı = −ıκ = |; (4) expressed uniquely in the form of (2). The coefficient of each {2 = |2 = κ2 = −1 imaginary unit a` (` = 1; : : : ; m) is represented as a` = =`(a). A multiplication table defines the products of any imaginary by letting m = 4, i1 = 1, i2 = {, i3 = | and i4 = κ. 2 2 − From (4), quaternions are not commutative, i.e., pq =6 qp unit with each other or with itself (e.g., i1 = 1; i2 = 1 for p; q 2 H in general. and i1i2 = i2i1 = i2 for A2(=: C)). The addition and the subtraction of two hypercomplex numbers are defined as 2) The octonion O can be constructed from the quaternion commutative binomial operations H by the C-D construction. Note that the multiplication in O is neither commutative nor associative, i.e., pq =6 qp ··· a b := (a1 b1)i1 + (a2 b2)i2 + + (am bm)im and (pq)r =6 p(qr) for p; q; r 2 O in general [10]. For the octonion multiplication table, see, e.g., [10]. for a; b 2 Am, where b := b1i1 + b2i2 + ··· + bmim, 2 R b1; : : : ; bm . From the unique expression of (2), the The C-D number system can be seen as an algebraically nat- multiplication of two hypercomplex numbers ural higher dimensional generalization of our familiar fields, R C ab = (a1i1 + a2i2 + ··· + amim)(b1i1 + b2i2 + ··· + bmim) i.e., and . AN f >j 2 A Xm Xm We also define m := [x1; : : : ; xN ] xi m (i = > := akb`iki` 2 Am 1;:::;N)g for 8N 2 N n f0g, where (·) stands for the H N k=1 `=1 transpose.
Details
-
File Typepdf
-
Upload Time-
-
Content LanguagesEnglish
-
Upload UserAnonymous/Not logged-in
-
File Pages8 Page
-
File Size-