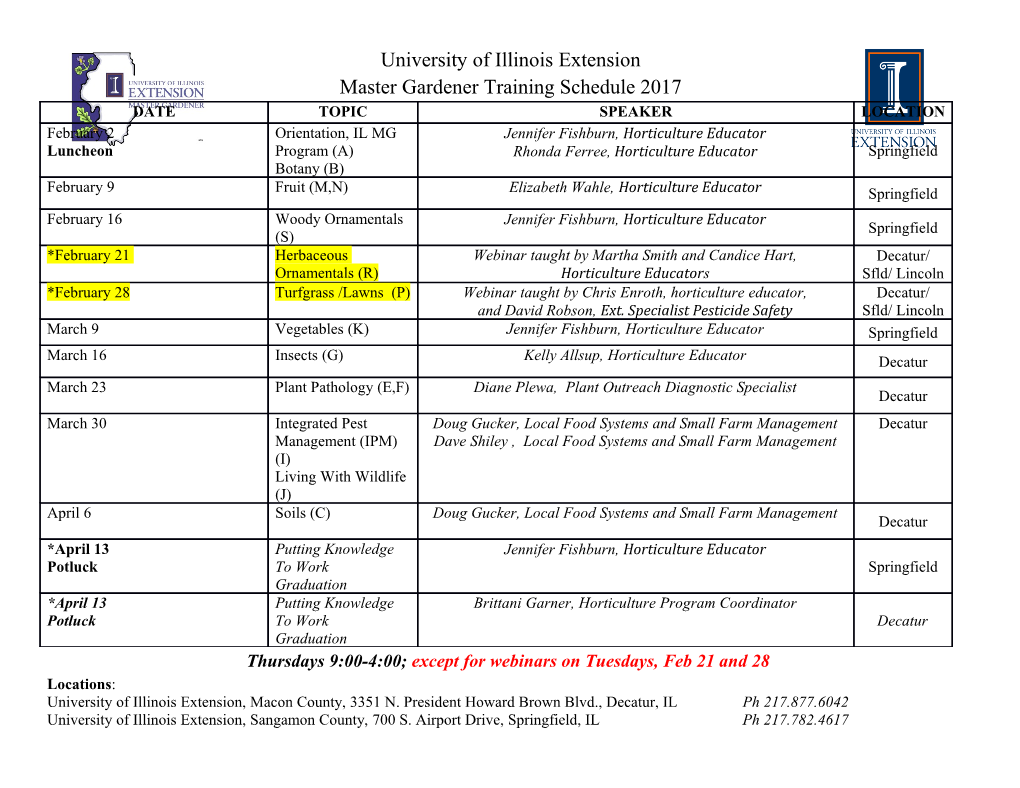
Rapid Data Quality Assessment Using Data Profiling David Loshin Knowledge Integrity, Inc. www.knowledge-integrity.com © 2010 Knowledge Integrity, Inc. 1 www.knowledge-integrity.com (301)754-6350 David Loshin, Knowledge Integrity Inc. David Loshin, president of Knowledge Integrity, Inc, (www.knowledge-integrity.com), is a recognized thought leader and expert consultant in the areas of data governance, data quality methods, tools, and techniques, master data management, and business intelligence. David is a prolific author regarding BI best practices, either via the expert channel at www.b-eye-network.com, “Ask The Expert” at Searchdatamanagement.techtarget.com, as well as numerous books on BI and data quality. His most recent book, “Master Data Management,” has been endorsed by data management industry leaders, and his valuable MDM insights can be reviewed at www.mdmbook.com. David can be reached at [email protected]. MDM Component Model © 2010 Knowledge Integrity, Inc. 2 www.knowledge-integrity.com (301)754-6350 1 Business-Driven Information Requirements Driver Benefit Information Requirement Increased revenue, increased share, cross- Unified master customer data, Customer sell/up-sell, segmentation, targeting, retention, matching/linkage, centralized analytics, Intelligence customer satisfaction, ease of doing business quality data, eliminate redundancy Compliance, privacy, risk management, accurate Data quality, semantic consistency Risk & response to audits, prevent fraud across business processes, consistency, Compliance availability Reduced M&A costs, lowered costs, streamlined STP, eliminate redundant data, Operational processes, increased volumes, increased functionality, licenses, rules/policy- Efficiency throughput, optimized promotions driven Faster onboarding, reduced vendor count, spend Matching/linkage, vendor management, Supplier management, improved supply chain 3rd party data integration Management management Product design, improved product and brand Unified product data, matching/linkage, Product management time to market, product centralized analytics Performance performance, better manufacturing processes Increased employee productivity, reduced Centralized analytics, unified employee Organizational reconciliations data, inspection, monitoring, control Performance © 2010 Knowledge Integrity, Inc. 3 www.knowledge-integrity.com (301)754-6350 Assessing the Quality of Data Important questions: Unified master customer data, What are the most critical business matching/linkage, centralized analytics, quality data, eliminate redundancy issues attributable to poor data quality? Data quality, semantic consistency across business processes, consistency, What constitutes “poor” data quality? availability How is data quality measured? STP, eliminate redundant data, What are the levels of acceptability? functionality, licenses, rules/policy- driven How are data issues managed? What remediation and correction Matching/linkage, vendor management, 3rd party data integration actions are feasible? How can we know when the data has Unified product data, matching/linkage, been improved? centralized analytics How is data quality improvement related to business process Centralized analytics, unified employee data, inspection, monitoring, control performance? © 2010 Knowledge Integrity, Inc. 4 www.knowledge-integrity.com (301)754-6350 2 Addressing the Problem To effectively ultimately address data quality, we must be able to manage the Identification of business client data quality expectations Definition of contextual metrics Assessment of levels of data quality Track issues for process management Determination of best opportunities for improvement Elimination of the sources of problems Continuous measurement of improvement against baseline © 2010 Knowledge Integrity, Inc. 5 www.knowledge-integrity.com (301)754-6350 Data Quality Assessment for Business Improvement Identify key business objectives and corresponding metrics Identify specific data issues related to known business impacts Correlate discovered issues to business impacts Data profiling and analysis Understand what you are working with, provide quantified metrics Improve automated matching/linkage Reduce false positives, expand universe of identifying attributes, reduce need for manual intervention Institute managed data quality Collect organizational data requirements, data inspection and control, incident management, data quality scorecards © 2010 Knowledge Integrity, Inc. 6 www.knowledge-integrity.com (301)754-6350 3 Analysis Process: Correlating Business and Data Issues Business Impact Analysis Empirical Analysis Identify business data issues Statistical analysis of actual Prioritize impacts existing data Identify critical data Identification of potential elements anomalies Correlate data dependencies Validation of known and business impacts expectations Engage business subject Data profiling matter experts Create Analyze/profile monitoring data system Data quality, Validity, & Assess Transformation Recommend business data rules data quality remediation expectations © 2010 Knowledge Integrity, Inc. 7 www.knowledge-integrity.com (301)754-6350 Tasks Review business process, use cases, data dependence and issues with customers’ data Isolate and quantify business impacts Identify critical mandatory elements and data quality expectations Examples: customers appear only once in data set Information product mapping Identify source tables Profile critical attributes from source data Report potential anomalies Review potential anomalies with clients to De-emphasize criticality (“Low priority”) Isolate for further review and analysis (“potential problem”) Select for remediation (“definite problem”) © 2009 Knowledge Integrity, Inc. 8 www.knowledge-integrity.com (301)754-6350 4 Data Quality Assessment – Process Business Plan Prepare Analyze Synthesize Review Process • Select business • Review system • List data sets • Data extraction • Review •Present anomalies process for review docs anomalies • Critical data • Data profiling •Verify criticality • Assess scope • Review existing elements • Describe issues DQ issues • Data analysis •Prioritize issues • Acquire sys docs • Proposed • Prepare report • Collate bus- measures • Drill-down •Suggest action • Identify iness impacts items business • Prepare DQ • Note findings impacts • Information tools •Review next production steps • Assess existing flow DQ process •Develop action plan • Project Plan © 2010 Knowledge Integrity, Inc. 9 www.knowledge-integrity.com (301)754-6350 Planning Identify team, tasks, resources, level of effort Create plan for data quality assessment process © 2010 Knowledge Integrity, Inc. 10 www.knowledge-integrity.com (301)754-6350 5 Business Process Evaluation Evaluate business impacts attributable to data flaws Select the specific business process(es) associated with those business impacts Review application system documentation Map business flows and production of information “products” © 2010 Knowledge Integrity, Inc. 11 www.knowledge-integrity.com (301)754-6350 Soliciting Business Data Quality Expectations Very straightforward interview questions: How do you use {customer, supplier, product, …} data? What are the biggest data issues impeding business success? Why are those the most critical problems? What do you do when you come across a data problem? What are your expectations when you report a problem? Provide any other perceptions about data quality © 2010 Knowledge Integrity, Inc. 12 www.knowledge-integrity.com (301)754-6350 6 Potential Business Impacts •Regulatory or Legislative risk •Health risk •System Development risk •Privacy risk •Information Integration Risk •Competitive risk •Investment risk •Fraud Detection Increased Risk •Detection and correction •Prevention •Spin control •Delayed/lost collections •Scrap and rework •Customer attrition Decreased Revenues Increased Costs •Penalties •Lost opportunities •Overpayments •Increased cost/volume Confidence Low •Increased resource costs •System delays •Increased workloads •Increased process times •Organizational trust issues •Impaired forecasting •Impaired decision-making •Inconsistent management reporting •Lowered predictability © 2010 Knowledge Integrity, Inc. 13 www.knowledge-integrity.com (301)754-6350 Categorizing Data Quality Impacts Monetary Confidence Risk Operating Costs Trust Regulatory Revenue Decisions Credit Opportunities Control Investment Cash Flow Forecasting Competitiveness Charges Reporting Development Budget/Spend Leakage Compliance Satisfaction Productivity Government Customer Workloads Industry Supplier Throughput Privacy Employee Processing Time Health Market Quality © 2010 Knowledge Integrity, Inc. 14 www.knowledge-integrity.com (301)754-6350 7 Classifying Business Impacts Impact Category Examples of issues for review Operational Efficiency Time and costs of cleansing data or processing corrections Inaccurate performance measurements for employees Inability to identify suppliers for spend analysis Risk/Compliance Missing data leads to inaccurate credit risk Regulatory compliance violations Revenue Lost opportunity cost Identification of high value opportunities Productivity Decreased ability for straight-through processing via automated services Procurement Efficiency Improved ease-of-use for staff (sales, call center, etc.) Improved ease of interaction for requestors and approver Reduced time from order to delivery Performance Impaired
Details
-
File Typepdf
-
Upload Time-
-
Content LanguagesEnglish
-
Upload UserAnonymous/Not logged-in
-
File Pages15 Page
-
File Size-