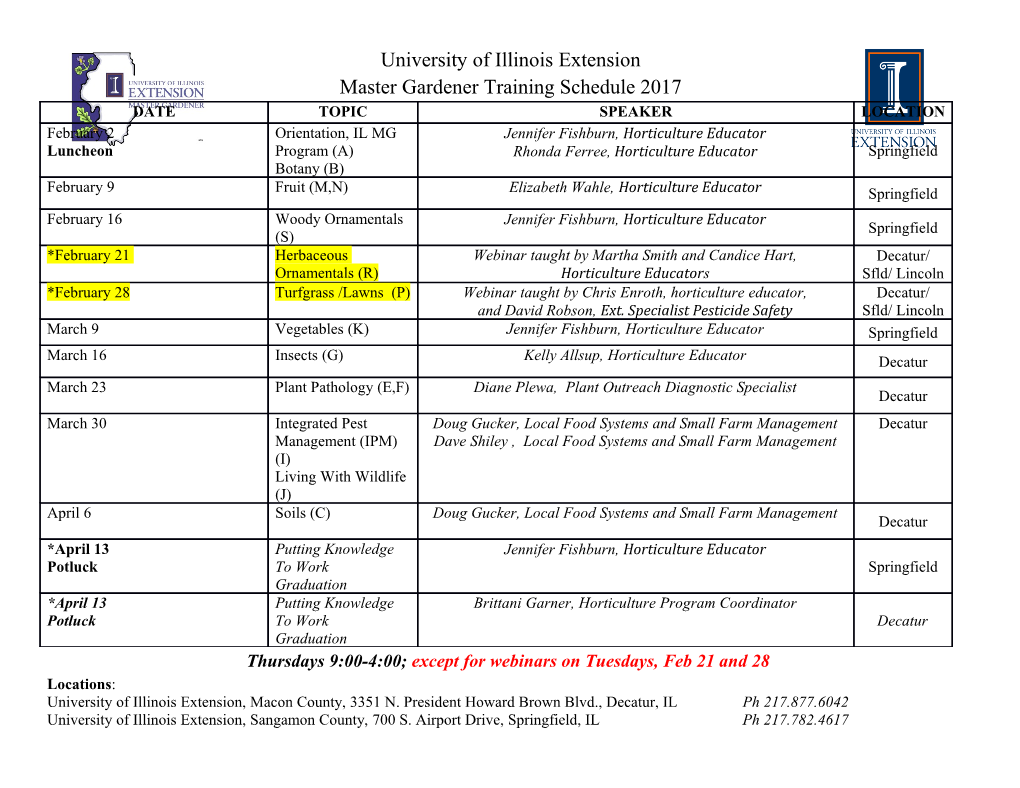
Journal of the American College of Cardiology Vol. 37, No. 4, 2001 © 2001 by the American College of Cardiology ISSN 0735-1097/01/$20.00 Published by Elsevier Science Inc. PII S0735-1097(01)01109-3 Development and Validation of the Ontario Acute Myocardial Infarction Mortality Prediction Rules Jack V. Tu, MD, PHD, FRCPC,* Peter C. Austin, PHD,* Randy Walld, BSc,† Leslie Roos, PHD,† Jean Agras, PHD,‡ Kathryn M. McDonald, MM§ Toronto and Winnipeg, Canada; and Stanford, California OBJECTIVES To develop and validate simple statistical models that can be used with hospital discharge administrative databases to predict 30-day and one-year mortality after an acute myocardial infarction (AMI). BACKGROUND There is increasing interest in developing AMI “report cards” using population-based hospital discharge databases. However, there is a lack of simple statistical models that can be used to adjust for regional and interinstitutional differences in patient case-mix. METHODS We used linked administrative databases on 52,616 patients having an AMI in Ontario, Canada, between 1994 and 1997 to develop logistic regression statistical models to predict 30-day and one-year mortality after an AMI. These models were subsequently validated in two external cohorts of AMI patients derived from administrative datasets from Manitoba, Canada, and California, U.S. RESULTS The 11-variable Ontario AMI mortality prediction rules accurately predicted mortality with an area under the receiver operating characteristic (ROC) curve of 0.78 for 30-day mortality and 0.79 for one-year mortality in the Ontario dataset from which they were derived. In an independent validation dataset of 4,836 AMI patients from Manitoba, the ROC areas were 0.77 and 0.78, respectively. In a second validation dataset of 112,234 AMI patients from California, the ROC areas were 0.77 and 0.78 respectively. CONCLUSIONS The Ontario AMI mortality prediction rules predict quite accurately 30-day and one-year mortality after an AMI in linked hospital discharge databases of AMI patients from Ontario, Manitoba and California. These models may also be useful to outcomes and quality measurement researchers in other jurisdictions. (J Am Coll Cardiol 2001;37:992–7) © 2001 by the American College of Cardiology Because management of coronary disease affects millions of In Ontario, we published the first hospital-specific AMI patients worldwide, the assessment of the outcomes of acute “report card” in Canada in 1999 (7). This report contained myocardial infarction (AMI) using population-based hos- information on the 30-day and one-year risk-adjusted pital discharge data bases is an important activity. AMI mortality rates for 52,616 AMI patients at 167 hospitals in “report cards” listing hospital-specific rates have been pub- Ontario between April 1, 1994, and March 31, 1997. As licly released in several U.S. states and some countries in part of the development of the Ontario AMI report, we Europe (1–4). However, to properly conduct these studies, created a simple 11-variable prediction rule using the an appropriate statistical model must be developed to adjust secondary diagnosis fields in the Ontario hospital discharge data bases to adjust for regional and interinstitutional See page 998 differences in AMI case mix. To evaluate the potential usefulness of this model to clinicians and researchers in for patient case-mix differences between institutions. A few other jurisdictions, we tested the model in two completely statistical models that have already been implemented using independent datasets of AMI patients in another Canadian administrative databases to predict AMI mortality are not province, Manitoba, and a large area of the U.S., the state of widely used because they require many variables and com- California. This study describes the derivation and valida- plex models and often have not been validated in other tion of the “Ontario AMI mortality prediction rules.” jurisdictions (3,5,6). METHODS From the *Institute for Clinical Evaluative Sciences, Toronto, Ontario, Canada; Data sources. The Ontario data for the study were taken Department of Medicine and Public Health Sciences, University of Toronto, Toronto, Ontario, Canada; †Manitoba Centre for Health Policy and Evaluation, from the Ontario Myocardial Infarction Database (OMID), Department of Community Health Sciences, Faculty of Medicine, University of which links together all of Ontario’s major health care Manitoba, Winnipeg, Manitoba, Canada; ‡National Bureau of Economic Research, administrative databases to create a large database for and the §Center for Primary Care and Outcomes Research, Department of Medicine, Stanford University, Stanford, California. Supported in part by an operating grant monitoring the quality of AMI care in Ontario. For the from the Canadian Institutes of Health Research, the National Institute on Aging, present study, we linked data on all patients discharged TECH grant AG17154, and the Heart and Stroke Foundation of Manitoba. Dr. Tu with a most responsible diagnosis of an AMI (Interna- is supported by a Canada Research Chair in Health Services Research. Manuscript received March 31, 2000; revised manuscript received August 2, 2000, tional Classification of Diseases [ICD]-9 code 410) in accepted December 14, 2000. Ontario between fiscal year 1994 and 1996 (April 1, 1994, JACC Vol. 37, No. 4, 2001 Tu et al. 993 March 15, 2001:992–7 Ontario Acute Myocardial Infarction Mortality Prediction Rules Potential risk factors for prediction model development. Abbreviations and Acronyms Forty-three potential candidate variables in addition to age AMI ϭ acute myocardial infarction and gender were considered for inclusion in the AMI ICD ϭ International Classification of Diseases mortality prediction rules (Table 1). These candidate vari- ϭ OMID Ontario Myocardial Infarction Database ables were taken from a list of risk factors used to develop OR ϭ odds ratio ROC ϭ receiver operating characteristic previous report cards in the California Hospital Outcomes TECH ϭ Technological Change in Health Care Project and Pennsylvania Health Care Cost Containment Council AMI “report card” projects (3,5). Each of these comorbidities was created using appropriate ICD-9 codes to March 31, 1997). The index hospitalization data were from the 15 secondary diagnosis fields in OMID. The obtained from the Canadian Institute for Health Informa- Ontario discharge data are based on ICD-9 codes rather than ICD-9-CM codes used in the U.S., so the U.S. codes tion hospital discharge database while long-term follow-up were truncated. Some risk factors used in these two projects data were obtained through the Ontario Registered Persons do not have an ICD-9 coding analog (e.g., infarct subtype, Database, which records the vital status of all Ontario race) and therefore were not included in our analysis. The residents. The Ontario discharge data contain 15 secondary frequency of each of these 43 comorbidities was calculated, diagnosis fields coded using the ICD ninth revision codes and any comorbidity with a prevalence of Ͻ1% was ex- and 15 corresponding diagnosis type indicators that indicate cluded from further analysis. Comorbidities that the authors whether a diagnosis is a preexisting comorbidity or a felt were not clinically plausible predictors of AMI mortality complication (Type II diagnosis) occurring after hospital were also excluded. The remaining variables were then admission. Only diagnoses coded as comorbidities were entered into a multivariate logistic regression model and used in the present study. backward stepwise regression was used to eliminate variables Two independent cohorts of AMI patients were created until only variables significant at the p Ͻ 0.05 level were left using similar methods from administrative databases in in the final model. The discrimination of the resulting Manitoba at the Manitoba Centre for Health Care Policy models was calculated by measuring the area under the and Evaluation, and in California from the California Office receiver operating characteristic (ROC) curve (10). of Statewide Health Planning and Development hospital Prediction model validation. The logistic regression mod- discharge database. els were developed to predict 30-day mortality and one-year In the California dataset, the “most responsible diagno- mortality in the Ontario dataset. Separate regression coef- sis” is specified as the “principal” diagnosis, and secondary ficients were fit for 30-day and one-year mortality. We also diagnosis codes do not distinguish between complications determined the prevalence of each of the comorbidities and comorbid conditions. using the Deyo adaption of the Charlson comorbidity index Inclusion/exclusion criteria. Similar inclusion/exclusion score (11) and calculated disease-specific regression coeffi- criteria were used to create the linked AMI datasets in the cients for the Charlson comorbidities so that we could three jurisdictions. Patients were included in the AMI compare the predictive performance of the Charlson model with those in the Ontario AMI mortality prediction rules. cohorts if they were admitted with a “most responsible” The resulting coefficients from the Ontario models were diagnosis (ICD-9 code 410) of AMI in Ontario or Mani- then applied in the independent Manitoba and California toba, or a “principal” diagnosis of AMI in California. AMI datasets to evaluate the generalizability of the models. Previous studies have shown the similarities of these two The areas under the ROC curves were compared in both
Details
-
File Typepdf
-
Upload Time-
-
Content LanguagesEnglish
-
Upload UserAnonymous/Not logged-in
-
File Pages6 Page
-
File Size-