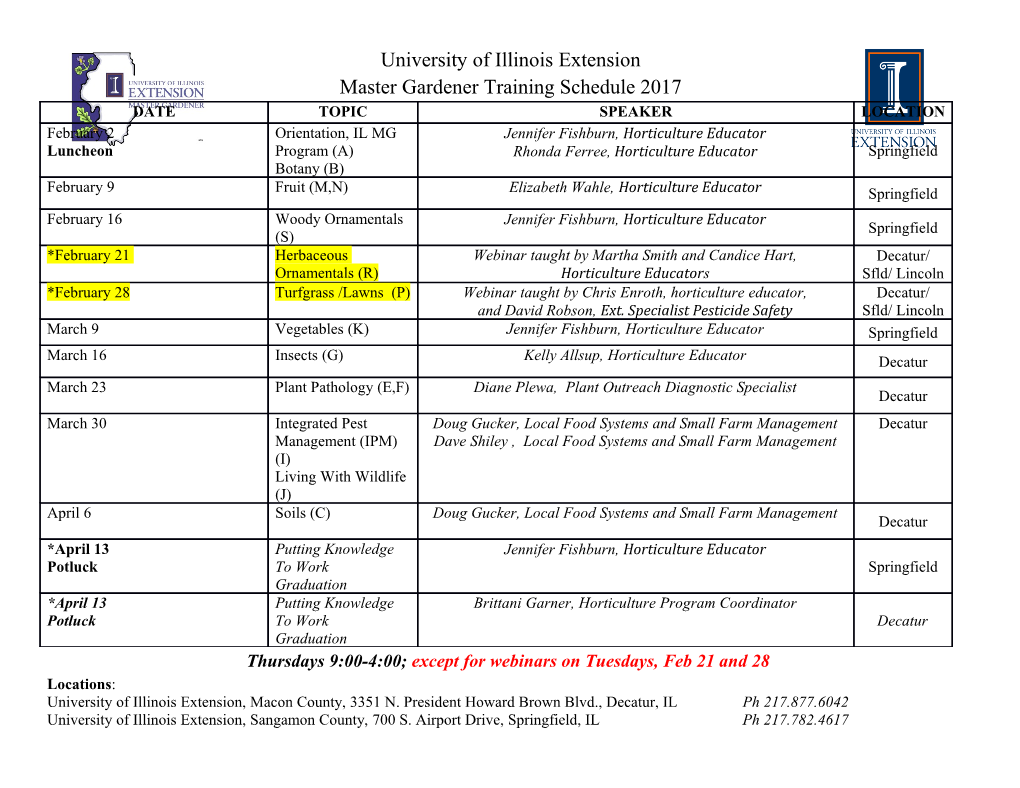
High-Performance Deep Learning and Machine Learning on Modern HPC Systems HPC-AI Advisory Council Australia Conference (Sept ’20) by Dhabaleswar K. (DK) Panda The Ohio State University E-mail: [email protected] Follow us on http://www.cse.ohio-state.edu/~panda https://twitter.com/mvapich High-End Computing (HEC): PetaFlop to ExaFlop 100 PetaFlops in 415 Peta 2017 Flops in 2020 (Fugaku in Japan with 7.3M cores 1 ExaFlops Expected to have an ExaFlop system in 2021! Network Based Computing Laboratory HPC-AI-Australia (Sept ‘20) 2 AI, Deep Learning & Machine Learning • Machine Learning (ML) with many traditional applications – K-means – Random Forest – Linear Regression – Nearest Neighbor • Deep Learning (DL) – A subset of Machine Learning that uses Deep Neural Networks (DNNs) • Based on learning data representation • Examples Convolutional Neural Networks, Recurrent Neural Networks, Hybrid Networks Courtesy: https://hackernoon.com/difference-between-artificial-intelligence-machine-learning- and-deep-learning-1pcv3zeg, https://blog.dataiku.com/ai-vs.-machine-learning-vs.-deep-learning Network Based Computing Laboratory HPC-AI-Australia (Sept ‘20) 3 Key Phases of Deep Learning • Deep Learning has two major tasks 1. Training of the Deep Neural Network 2. Inference (or deployment) that uses a trained DNN • DNN Training – Training is a compute/communication intensive process – can take days to weeks – Faster training is necessary! • Faster training can be achieved by – Using Newer and Faster Hardware – But, there is a limit! – Can we use more GPUs or nodes? • The need for Parallel and Distributed Training Network Based Computing Laboratory HPC-AI-Australia (Sept ‘20) 4 Introduction to Dask and GPU-based Data Science • Dask is a popular task-based distributed computing framework: – Scales Python applications from laptops to high-end systems – Builds a task-graph that is executed lazily on parallel hardware – Natively extends popular data processing libraries like numPy, Pandas • NVIDIA RAPIDS framework is a GPU-based data science ecosystem around Dask: – Aims to hide low-level complexities of the CUDA framework – cuPy/cuDF/cuML are GPU-counterparts of numPy/Pandas/Scikit-learn • The Dask Distributed library supports parallel and distributed execution: – Built using the asyncio package that allows execution of asynchronous/non- blocking/concurrent operations called coroutines: • These are defined using async and invoked using await Network Based Computing Laboratory HPC-AI-Australia (Sept ‘20) 5 cuML: GPU-accelerated Machine Learning Library • Collection of ML algorithms and mathematical primitives for GPUs: – Maintains a similar interface to Scikit-learn – Hides the complexities of CUDA programming from data scientists • Scales out using the Dask ecosystem • cuML supports execution on a variety of platforms: – A single GPU – Single-Node Multi-GPUs (SNMG) – Multi-Node Multi-GPUs (MNMG) Image Courtesy: https://rapids.ai/about.html Network Based Computing Laboratory HPC-AI-Australia (Sept ‘20) 6 Scale-up and Scale-out Desired • Scale-up: Intra-node Communication – Many improvements like: NCCL2 • NVIDIA cuDNN, cuBLAS, NCCL, etc. • CUDA Co-operative Groups cuDNN MPI • Scale-out: Inter-node Communication MKL-DNN – DL and ML Frameworks – most are optimized for single-node only up Performance – Distributed (Parallel) Execution is an - gRPC emerging trend Scale Hadoop Scale-out Performance Network Based Computing Laboratory HPC-AI-Australia (Sept ‘20) 7 Broad Challenge: Exploiting HPC for DL and ML How to efficiently Scale-up and scale-out Deep Learning (DL) and Machine Learning (ML) frameworks and take advantage of heterogeneous High Performance Computing (HPC) resources? Network Based Computing Laboratory HPC-AI-Australia (Sept ‘20) 8 Programming Models and Runtimes for Multi-Petaflop and Exaflop Systems: Challenges Application Kernels/Applications (HPC, DL, and ML) Co-Design Middleware Opportunities and Programming Models Challenges MPI, PGAS (UPC & OpenSHMEM), CUDA, OpenMP, OpenACC, Hadoop, across Various Spark (RDD, DAG), TensorFlow, PyTorch, DASK, cuML, etc. Layers Communication Library or Runtime for Programming Models Performance Point-to-point Collective Energy- Synchronization I/O and Fault Communication Communication Awareness and Locks File Systems Tolerance Scalability Resilience Networking Technologies Multi-/Many-core Accelerators (InfiniBand, 40/100/200GigE, Architectures (GPU and FPGA) RoCE, Omni-Path, EFA, and Slingshot) Network Based Computing Laboratory HPC-AI-Australia (Sept ‘20) 9 Overview of the MVAPICH2 Project • High Performance open-source MPI Library • Support for multiple interconnects – InfiniBand, Omni-Path, Ethernet/iWARP, RDMA over Converged Ethernet (RoCE), and AWS EFA • Support for multiple platforms – x86, OpenPOWER, ARM, Xeon-Phi, GPGPUs (NVIDIA and AMD (upcoming)) • Started in 2001, first open-source version demonstrated at SC ‘02 • Used by more than 3,100 organizations in 89 countries • Supports the latest MPI-3.1 standard • More than 827,000 (> 0.8 million) downloads from the • http://mvapich.cse.ohio-state.edu OSU site directly • Additional optimized versions for different systems/environments: • Empowering many TOP500 clusters (June ‘20 ranking) – MVAPICH2-X (Advanced MPI + PGAS), since 2011 – 4th , 10,649,600-core (Sunway TaihuLight) at NSC, Wuxi, China – MVAPICH2-GDR with support for NVIDIA GPGPUs, since 2014 – 8th, 448, 448 cores (Frontera) at TACC – MVAPICH2-MIC with support for Intel Xeon-Phi, since 2014 – 12th, 391,680 cores (ABCI) in Japan – MVAPICH2-Virt with virtualization support, since 2015 – 18th, 570,020 cores (Nurion) in South Korea and many others – MVAPICH2-EA with support for Energy-Awareness, since 2015 – MVAPICH2-Azure for Azure HPC IB instances, since 2019 • Available with software stacks of many vendors and – MVAPICH2-X-AWS for AWS HPC+EFA instances, since 2019 Linux Distros (RedHat, SuSE, OpenHPC, and Spack) • Tools: • Partner in the 8th ranked TACC Frontera system – OSU MPI Micro-Benchmarks (OMB), since 2003 • Empowering Top500 systems for more than 15 years – OSU InfiniBand Network Analysis and Monitoring (INAM), since 2015 Network Based Computing Laboratory HPC-AI-Australia (Sept ‘20) 10 Architecture of MVAPICH2 Software Family (for HPC, DL, and ML) High Performance Parallel Programming Models Message Passing Interface PGAS Hybrid --- MPI + X (MPI) (UPC, OpenSHMEM, CAF, UPC++) (MPI + PGAS + OpenMP/Cilk) High Performance and Scalable Communication Runtime Diverse APIs and Mechanisms Point-to- Remote Collectives Energy- I/O and Fault Active Introspection point Job Startup Memory Virtualization Algorithms Awareness File Systems Tolerance Messages & Analysis Primitives Access Support for Modern Networking Technology Support for Modern Multi-/Many-core Architectures (InfiniBand, iWARP, RoCE, EFA, Omni-Path) (Intel-Xeon, OpenPower, Xeon-Phi, ARM, NVIDIA & AMD GPUs) Transport Protocols Modern Features Transport Mechanisms Modern Features SR- Multi Shared RC XRC UD DC SHARP2* ODP CMA IVSHMEM XPMEM MCDRAM* NVLink CAPI* IOV Rail Memory * Upcoming Network Based Computing Laboratory HPC-AI-Australia (Sept ‘20) 11 Network Based Computing Computing Laboratory Based Network Number of Downloads 900000 100000 200000 300000 400000 500000 600000 700000 800000 0 MVAPICH2 Release Timeline and Release and Timeline DownloadsMVAPICH2 Sep-04 Mar-05 Sep-05 Mar-06 MV 0.9.4 Sep-06 Mar-07 MV2 0.9.0 Sep-07 Mar-08 Sep-08 MV2 0.9.8 Mar-09 Sep-09 MV2 1.0 HPC Mar-10 - Sep-10 AI MV 1.0 - Australia (Sept (Sept ‘20) Australia Mar-11 MV2 1.0.3 Sep-11 MV 1.1 Timeline Mar-12 Sep-12 Mar-13 MV2 1.4 Sep-13 Mar-14 MV2 1.5 Sep-14 Mar-15 MV2 1.6 Sep-15 Mar-16 MV2 1.7 Sep-16 MV2 1.8 Mar-17 Sep-17 MV2 1.9 Mar-18 MV2-GDR 2.0b Sep-18 MV2 Virt 2.2MV2-MIC 2.0 Mar-19 MV2-Azure 2.3.2 MV2-AWS 2.3 Sep-19 MV2 2.3.4 MV2-GDR 2.3.4 12 Mar-20 MV2-X 2.3 OSU INAM 0.9.6 Accelerating DL and ML Applications with MVAPICH2 MPI Libraries • MPI-driven Deep Learning – CPU-based Deep Learning – GPU-based Deep Learning • Out-of-core DNN training • Exploiting Hybrid (Data and Model) Parallelism • Use-Case: AI-Driven Digital Pathology • High-Performance MPI runtime for DASK • MPI-driven Acceleration of cuML Algorithms • Commercial Support and Products Network Based Computing Laboratory HPC-AI-Australia (Sept ‘20) 13 High-Performance Distributed Data Parallel Training with TensorFlow • gRPC – Officially available and supported – Open-source – can be enhanced by others – Accelerated gRPC (add RDMA to gRPC) • gRPC+X – Use gRPC for bootstrap and rendezvous – Actual communication is in “X” – X MPI, Verbs, GPUDirect RDMA (GDR), etc. • No-gRPC – Baidu – the first one to use MPI Collectives for TF – Horovod – Use NCCL, or MPI, or any other future library (e.g. IBM DDL support recently added) A. A. Awan, J. Bedorf, C-H Chu, H. Subramoni, and DK Panda., “Scalable Distributed DNN Training using TensorFlow and CUDA-Aware MPI: Characterization, Designs, and Performance Evaluation”, CCGrid’19 Network Based Computing Laboratory HPC-AI-Australia (Sept ‘20) 14 MVAPICH2 (MPI)-driven Infrastructure for ML/DL Training ML/DL Applications ML/DL Applications TensorFlow PyTorch MXNet PyTorch Horovod Torch.distributed DeepSpeed MVAPICH2 or MVAPICH2-X MVAPICH2-GDR for MVAPICH2 or MVAPICH2-X MVAPICH2-GDR for for CPU Training GPU Training for CPU Training GPU Training More details available from: http://hidl.cse.ohio-state.edu Network Based Computing Laboratory HPC-AI-Australia (Sept ‘20) 15 MVAPICH2 Software Family (CPU-Based Deep Learning) High-Performance Parallel
Details
-
File Typepdf
-
Upload Time-
-
Content LanguagesEnglish
-
Upload UserAnonymous/Not logged-in
-
File Pages66 Page
-
File Size-