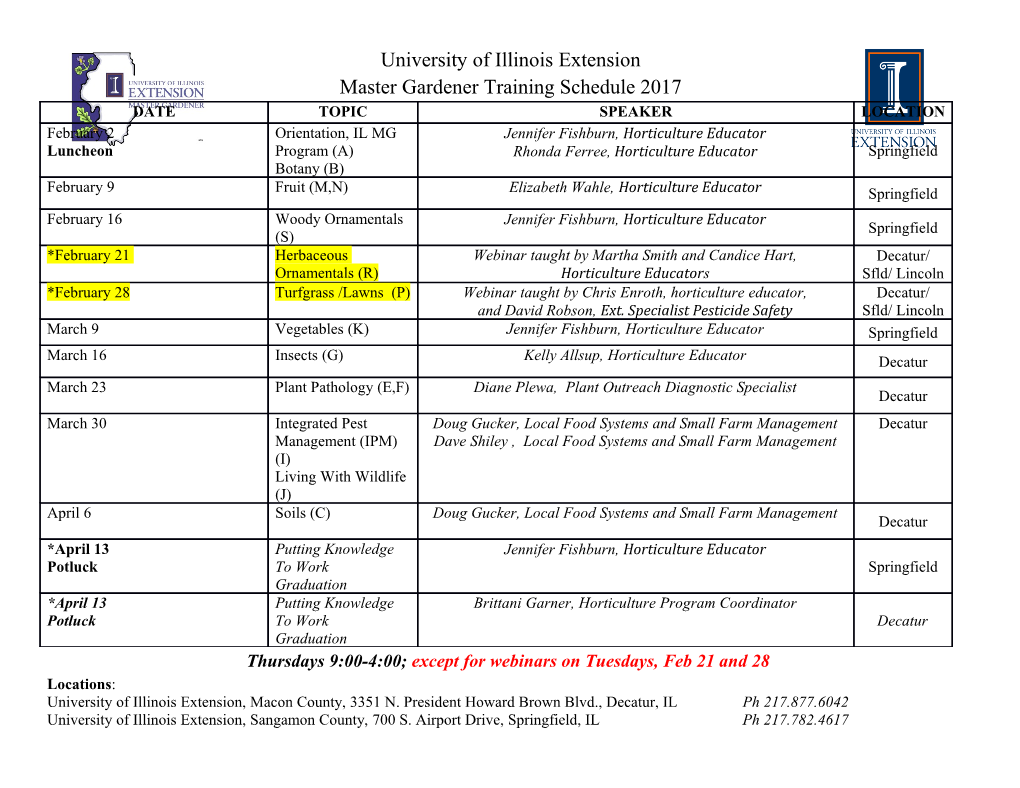
The Thirty-Third AAAI Conference on Artificial Intelligence (AAAI-19) Learning to Adaptively Scale Recurrent Neural Networks Hao Hu,1 Liqiang Wang,1 Guo-Jun Qi2 1University of Central Florida 2Huawei Cloud fhaohu, [email protected], [email protected] Abstract fit the temporal dynamics throughout the time. Although pat- terns in different scale levels require distinct frequencies to Recent advancements in recurrent neural network (RNN) re- update, they do not always stick to a certain scale and could search have demonstrated the superiority of utilizing multi- vary at different time steps. For example, in polyphonic mu- scale structures in learning temporal representations of time series. Currently, most of multiscale RNNs use fixed scales, sic modeling, distinguishing different music styles demands which do not comply with the nature of dynamical temporal RNNs to model various emotion changes throughout music patterns among sequences. In this paper, we propose Adap- pieces. While emotion changes are usually controlled by the tively Scaled Recurrent Neural Networks (ASRNN), a sim- lasting time of notes, it is insufficient to model such patterns ple but efficient way to handle this problem. Instead of using using only fixed scales as the notes last differently at dif- predefined scales, ASRNNs are able to learn and adjust scales ferent time. Secondly, stacking multiple RNN layers greatly based on different temporal contexts, making them more flex- increases the complexity of the entire model, which makes ible in modeling multiscale patterns. Compared with other RNNs even harder to train. Unlike this, another group of multiscale RNNs, ASRNNs are bestowed upon dynamical multiscale RNNs models scale patterns through gate struc- scaling capabilities with much simpler structures, and are tures (Neil, Pfeiffer, and Liu 2016)(Campos et al. 2017)(Qi easy to be integrated with various RNN cells. The exper- iments on multiple sequence modeling tasks indicate AS- 2016). In such cases, additional control gates are learned to RNNs can efficiently adapt scales based on different sequence optionally update hidden for each time step, resulting in a contexts and yield better performances than baselines without more flexible sequential representations. Yet such modeling dynamical scaling abilities. strategy may not remember information which is more im- portant for future outputs but less related to current states. Introduction In this paper, we aim to model the underlying multi- Recurrent Neural Networks (RNNs) play a critical role in scale temporal patterns for time sequences while avoiding sequential modeling as they have achieved impressive per- all the weaknesses mentioned above. To do so, we present formances in various tasks (Campos et al. 2017)(Chang Adaptively Scaled Recurrent Neural Networks (ASRNNs), et al. 2017)(Chung, Ahn, and Bengio 2016)(Neil, Pfeiffer, a simple extension for existing RNN structures, which al- and Liu 2016). Yet learning long-term dependencies from lows them to adaptively adjust the scale based on tempo- long sequences still remains a very difficult task (Bengio, ral contexts at different time steps. Using the causal convo- Simard, and Frasconi 1994) (Hochreiter et al. 2001)(Ye et lution proposed by (Van Den Oord et al. 2016), ASRNNs al. 2017)(Hu et al. 2017). Among various ways that try to model scale patterns by firstly convolving input sequences handle this problem, modeling multiscale patterns seem to with wavelet kernels, resulting in scale-related inputs that be a promising strategy since many multiscale RNN struc- parameterized by the scale coefficients from kernels. After tures perform better than other non-scale modeling RNNs that, scale coefficients are sampled from categorical distri- in multiple applications (Koutnik et al. 2014)(Neil, Pfeif- butions determined by different temporal contexts. This is fer, and Liu 2016)(Chung, Ahn, and Bengio 2016)(Chang achieved by sampling Gumbel-Softmax (GM) distributions et al. 2017)(Campos et al. 2017)(Chang et al. 2014). Multi- instead, which are able to approximate true categorical dis- scale RNNs can be roughly divided into two groups based on tributions through the re-parameterization trick. Due to the their design philosophies. The first group trends to model- differentiable nature of GM, ASRNNs could learn to flexi- ing scale patterns with the hierarchical architectures and pre- bly determine which scale is most important to target outputs fixed scales for different layers. This may lead to at least two according to temporal contents at each time step. Compared disadvantages. First, the prefixed scale can not be adjusted to with other multiscale architectures, the proposed ASRNNs have several advantages. First, there is no fixed scale in the Copyright c 2019, Association for the Advancement of Artificial model. The subroutine for scale sampling can be trained to Intelligence (www.aaai.org). All rights reserved. select proper scales to dynamically model the temporal scale 3822 patterns. Second, ASRNNs can model multiscale patterns tiscale RNNs model scale patterns using control gates to within a single RNN layer, resulting in a much simpler struc- decide whether to update hidden states or not at a certain ture and easier optimization process. Besides, ASRNNs do time step. Such structures like phased LSTMs (Neil, Pfeif- not use gates to control the updates of hidden states. Thus fer, and Liu 2016) and skip RNNs (Campos et al. 2017), are there is no risk of missing information for future outputs. able to adjust their modeling scales based on current tem- To verify the effectiveness of ASRNNs, we conduct ex- poral contexts, leading to more reasonable and flexible se- tensive experiments on various sequence modeling tasks, in- quential representations. Recently, some multiscale RNNs cluding low density signal identification, long-term mem- like hierarchical multi-scale RNNs (Chung, Ahn, and Ben- orization, pixel-to-pixel image classification, music genre gio 2016), manage to combine the gate-controlling updating recognition and language modeling. Our results suggest that mechanism into hierarchical architectures and has made im- ASRNNs can achieve better performances than their non- pressive progress in language modeling tasks. Yet they still adaptively scaled counterparts and are able to adjust scales employ multi-layer structures which make the optimization according to various temporal contents. We organize the not be easy. rest paper like this: the first following section reviews rel- ative literatures, then we introduce ASRNNs with details in Adaptively Scaled Recurrent Neural Networks next section; after that the results for all evaluations are pre- In this section we introduce Adaptively Scaled Recurrent sented, and the last section concludes the paper. Neural Networks (ASRNNs), a simple but useful extension for various RNN cells that allows to dynamically adjust Related Work scales at each time step. An ASRNNs is consist of three As a long-lasting research topic, the difficulties of train- components: scale parameterization, adaptive scale learning ing RNNs to learn long-term dependencies are considered and RNN cell integration, which will be covered in follow- to be caused by several reasons. First, the gradient explod- ing subsections. ing and vanishing problems during back propagation make training RNNs very tough (Bengio, Simard, and Frasconi Scale Parameterization 1994) (Hochreiter et al. 2001). Secondly, RNN memory cells We begin our introduction for ASRNNs with scale param- usually need to keep both long-term dependencies and short- eterization. Suppose X = [x1; x2 ··· ; xT ] is an input se- term memories simultaneously, which means there should n quence where xt 2 R . At time t, instead of taking only always be trade-offs between two types of information. To the current frame x as input, ASRNNs compute an alterna- overcome such problems, some efforts aim to design more t tive scale-related input ~xt, which can be obtained by taking sophisticated memory cell structures. For example, Long- a causal convolution between the original input sequence X short term memory (LSTM) (Hochreiter and Schmidhuber and a scaled wavelet kernel function φjt . 1997) and gated recurrent unit (GRU) (Chung et al. 2014), More specifically, let J be the number of considered are able to capture more temporal information; while some scales. Consider a wavelet kernel φ of size K. At any time t, others attempt to develop better training algorithms and given a scale jt 2 f0; ··· ;J − 1g, the input sequence X is initialization strategies such as gradient clipping (Pascanu, i convolved with a scaled wavelet kernel φjt = φ( 2jt ). This Mikolov, and Bengio 2013), orthogonal and unitary weight yields the following scaled-related input ~x at time t optimization (Arjovsky, Shah, and Bengio 2016)(Le, Jaitly, t and Hinton 2015) (Wisdom et al. 2016)(Qi, Hua, and Zhang 2jt K−1 X i n 2009)(Wang et al. 2016)(Qi, Aggarwal, and Huang 2012) ~xt = (X ∗ φj )t = xt−iφ( ) 2 R (1) t 2jt etc. These techniques can alleviate the problem to some ex- i=0 tent (Tang et al. 2017)(Li et al. 2017)(Wang et al. 2012). Meanwhile, previous works like (Koutnik et al. 2014) where for any i 2 ft − 2jt K + 1; ··· ; t − 1g, we manually (Neil, Pfeiffer, and Liu 2016) (Chung, Ahn, and Bengio set xi = 0 iff i ≤ 0. And the causal convolution operator ∗ 2016) (Tang et al. 2007) (Hua and Qi 2008) suggest learning (Van Den Oord et al. 2016) is defined to avoid the resultant i temporal scale structures is also the key to this problem. This ~xt depending on future inputs. We also let φ( 2jt ) = 0 iff jt stands upon the fact that temporal data usually contains rich 2 - i. It is easy to see that ~xt can only contain information jt underlying multiscale patterns (Schmidhuber 1991)(Mozer from xt−i when i = 2 k; k 2 f1; ··· ;Kg. In other words, 1992) (El Hihi and Bengio 1996) (Lin et al. 1996) (Hu and there are skip connections between xt−2jt (k−1) and xt−2jt k Qi 2017).
Details
-
File Typepdf
-
Upload Time-
-
Content LanguagesEnglish
-
Upload UserAnonymous/Not logged-in
-
File Pages8 Page
-
File Size-