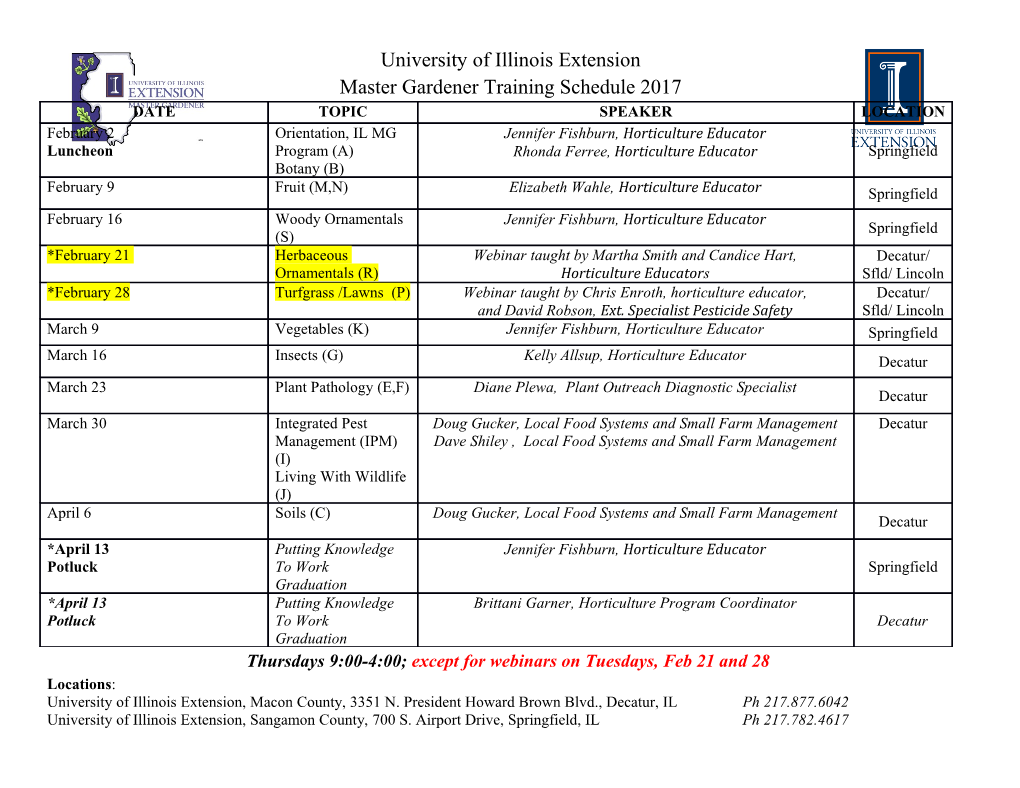
Speech Recognition Lecture 5: N-gram Language Models Mehryar Mohri Courant Institute and Google Research [email protected] Language Models Definition: probability distribution Pr[ w ] over sequences of words w = w1 ...wk. • Critical component of a speech recognition system. Problems: • Learning: use large text corpus (e.g., several million words) to estimate Pr[ w ] . Models in this course: n-gram models, maximum entropy models. • Efficiency: computational representation and use. Mehryar Mohri - Speech Recognition page 2 Courant Institute, NYU This Lecture n-gram models definition and problems Good-Turing estimate Smoothing techniques Evaluation Representation of n-gram models Shrinking LMs based on probabilistic automata Mehryar Mohri - Speech Recognition page 3 Courant Institute, NYU N-Gram Models Definition: an n-gram model is a probability distribution based on the nth order Markov assumption ∀i, Pr[wi | w1 ...wi−1]=Pr[wi | hi], |hi|≤n − 1. • Most widely used language models. Consequence: by the chain rule, k k Pr[w]=! Pr[wi | w1 ...wi−1]=! Pr[wi | hi]. i=1 i=1 Mehryar Mohri - Speech Recognition page 4 Courant Institute, NYU Maximum Likelihood Likelihood: probability of observing sample under distribution p ∈ P , which, given the independence assumption is m Pr[x1,...,xm]=! p(xi). i=1 Principle: select distribution maximizing sample probability m p! = argmax ! p(xi), ∈P p i=1 m or p! = argmax ! log p(xi). ∈P p i=1 Mehryar Mohri - Speech Recognition page 5 Courant Institute, NYU Example: Bernoulli Trials Problem: find most likely Bernoulli distribution, given sequence of coin flips H, T, T, H, T, H, T, H, H, H, T, T, . , H. Bernoulli distribution: p(H)=θ, p(T )=1− θ. Likelihood: l(p) = log θN(H)(1 − θ)N(T ) = N(H) log θ + N(T ) log(1 − θ). Solution: l is differentiable and concave; dl(p) N(H) N(T ) N(H) = =0 θ = . dθ θ − 1 θ ⇔ N(H)+N(T ) Mehryar Mohri - Speech Recognition − page 6 Courant Institute, NYU Maximum Likelihood Estimation Definitions: • n-gram: sequence of n consecutive words. • S : sample or corpus of size m . c w ...w • ( 1 k ) : count of sequence w 1 ...w k . ML estimates: for c ( w 1 ...w n − 1 ) =0! , c(w1 ...wn) Pr[wn|w1 ...wn−1]= . c(w1 ...wn−1) • But, c(w1 ...wn)=0 =⇒ Pr[wn|w1 ...wn−1]=0! Mehryar Mohri - Speech Recognition page 7 Courant Institute, NYU N-Gram Model Problems Sparsity: assigning probability zero to sequences not found in the sample = ⇒ speech recognition errors. • Smoothing: adjusting ML estimates to reserve probability mass for unseen events. Central techniques in language modeling. • Class-based models: create models based on classes (e.g., DAY) or phrases. Representation: for | Σ | =100 , 000 , the number of bigrams is 10 10 , the number of trigrams 10 15 ! • Weighted automata: exploiting sparsity. Mehryar Mohri - Speech Recognition page 8 Courant Institute, NYU Smoothing Techniques Typical form: interpolation of n-gram models, e.g., trigram, bigram, unigram frequencies. Pr[w3|w1w2]=α1c(w3|w1w2)+α2c(w3|w2)+α3c(w3). Some widely used techniques: • Katz Back-off models (Katz, 1987). • Interpolated models (Jelinek and Mercer, 1980). • Kneser-Ney models (Kneser and Ney, 1995). Mehryar Mohri - Speech Recognition page 9 Courant Institute, NYU Good-Turing Estimate (Good, 1953) Definitions: • Sample S of m words drawn from vocabulary Σ . • c ( x ) : count of word x in S . • S k : set of words appearing k times. • M k : probability of drawing a point in S k . Good-Turing estimate of M k : k k S G +1 S ( +1)| k+1|. k = m | k+1| = m Mehryar Mohri - Speech Recognition page 10 Courant Institute, NYU Properties Theorem: the Good-Turing estimate is an estimate of M k with small bias, for small values of k/m : k +1 E[Gk]=E[Mk]+O( ). S S m Proof: E[Mk]= Pr[x] Pr[x ∈ Sk] S x!∈Σ m = Pr[x] Pr[x]k(1 − Pr[x])m−k " k # x!∈Σ m m = Pr[x]k+1(1 − Pr[x])m−(k+1) k (1 − Pr[x]) "k +1# $m% x!∈Σ k+1 k +1 m $ % k +1 = E |Sk | − E Mk = E[Gk] − E[Mk ]. m − k & +1 +1 ) m − k m − k +1 ' ( ' ( Mehryar Mohri - Speech Recognition page 11 Courant Institute, NYU Properties Proof (cont.): thus, ! ! ! k k +1 ! !E[Mk] − E[Gk]! = ! E[Gk] − E[Mk+1]! !S S ! !m − k S m − k ! k +1 ≤ . (0 ≤ E[Gk], E[Mk+1] ≤ 1) m − k S In particular, for k =0 , ! ! 1 !E[Mk] − E[Gk]! ≤ . !S S ! m It can be proved using McDiarmid’s inequality that with probability at least 1 − δ , (McAllester and Schapire, 2000), 1 log( δ ) M0 G0 + O . ≤ m Mehryar Mohri - Speech Recognition page 12 Courant Institute, NYU Good-Turing Count Estimate Definition: let r = c ( w 1 ...w k ) and n r = | S r |, then Gr m nr+1 c∗(w ...w )= × =(r +1) . 1 k S n | r| r Mehryar Mohri - Speech Recognition page 13 Courant Institute, NYU Simple Method (Jeffreys, 1948) Additive smoothing: add δ ∈ [0 , 1] to the count of each n-gram. c(w1 ...wn)+δ Pr[wn|w1 ...wn−1]= . c(w1 ...wn−1)+δ|Σ| • Poor performance (Gale and Church, 1994). • Not a principled attempt to estimate or make use of an estimate of the missing mass. Mehryar Mohri - Speech Recognition page 14 Courant Institute, NYU Katz Back-off Model (Katz, 1987) Idea: back-off to lower order model for zero counts. c wn−1 w wn−1 w wn−1 • if ( 1 )=0 , then Pr[ n | 1 ]=Pr[ n | 2 ] . otherwise, • n c(w1 ) n dc wn c w > n−1 ( 1 ) n−1 if ( 1 ) 0 wn w c w Pr[ | 1 ]= ( 1 ) n−1 β Pr[wn|w2 ] otherwise. where d k is a discount coefficient such that 1 if k>K; dk = (k +1)nk+1 ≈ otherwise. knk Katz suggests K =5 . Mehryar Mohri - Speech Recognition page 15 Courant Institute, NYU Discount Coefficient With the Good-Turing estimate, the total probability mass for unseen n-grams is G 0 = n 1 /m . n dc(wn)c(w )/m =1 n1/m 1 1 − c(wn)>0 1 d kn = m n ⇔ k k − 1 k>0 d kn = kn n ⇔ k k k − 1 k>0 k>0 (1 d ) kn = n . ⇔ − k k 1 k>0 K (1 d ) kn = n . ⇔ − k k 1 k=1 Mehryar Mohri - Speech Recognition page 16 Courant Institute, NYU Discount Coefficient k∗ Solution: a search with 1 − d k = µ (1 − ) leads to k K − ∗ µ !(1 k /k) knk = n1. k=1 K ⇔ − (k +1)nk+1 µ !(1 ) knk = n1. knk k=1 K ⇔ − µ ![knk (k +1)nk+1]=n1. k=1 ⇔ µ[n1 − (K +1)nK+1]=n1. ⇔ 1 µ = K n 1 − ( +1) K+1 n1 ∗ k (K+1)nK+1 k − n ⇔ 1 dk = K n . 1 − ( +1) K+1 n1 Mehryar Mohri - Speech Recognition page 17 Courant Institute, NYU Interpolated Models (Jelinek and Mercer, 1980) Idea: interpolation of different order models. Pr[w3|w1w2]=αc(w3|w1w2)+βc(w3|w2)+(1− α − β)c(w3), with 0 ≤ α,β ≤ 1. • α and β are estimated by using held-out samples. • sample split into two parts for training higher- order and lower order models. • optimization using expectation-maximization (EM) algorithm. • deleted interpolation: k-fold cross-validation. Mehryar Mohri - Speech Recognition page 18 Courant Institute, NYU Kneser-Ney Model Idea: combination of back-off and interpolation, but backing-off to lower order model based on counts of contexts. Extension of absolute discounting. max {0,c(w1w2w3) − D} c(·w3) Pr[w3|w1w2]= + α , c(w1w2) ! c(·w3) where D is a constant. Modified version (Chen and Goodman, 1998): D function of c ( w 1 w 2 w 3 ) . Mehryar Mohri - Speech Recognition page 19 Courant Institute, NYU Evaluation Average log-likelihood of test sample of size N: N 1 L(p)= log p[w h ], h n 1. N 2 k | k | k| ≤ − k=1 Perplexity : the perplexity PP ( q ) of the model is defined as (‘average branching factor’) Lˆ(q) PP(q)=2− . • For English texts, typically PP ( q ) ∈ [50 , 1000] and 6 L(q) 10 bits. ≤ ≤ Mehryar Mohri - Speech Recognition page 20 Courant Institute, NYU In Practice Evaluation: an empirical observation based on the word error rate of a speech recognizer is often a better evaluation than perplexity. n-gram order: typically n = 3, 4, or 5. Higher order n-grams typically do not yield any benefit. Smoothing: small differences between Back-off, interpolated, and other models (Chen and Goodman, 1998). Special symbols: beginning and end of sentences, start and stop symbols. Mehryar Mohri - Speech Recognition page 21 Courant Institute, NYU The set of states of the WFA representing a backoff or </s>/1.101 interpolated model is defined by associating a state qh to a/0.405 a/0.287 </s> each sequence of length less than n found in the corpus: a !/4.856 </s>/1.540 a/1.108 Q = {qh : |h| < n and c(h) > 0} a/0.441 /0.356 !/0.231 ! b Its transition set E is defined as the union of the following <s> b/0.693 b/1.945 set of failure transitions: Figure 3: Example of representation of a bigram model {(qwh! , φ, − log(αh), qh! ) : qwh! ∈ Q} with failure transitions. and the following set of regular transitions: cost of the invalid path to the state – the sum of the two transition costs (0.672) – is lower than the cost of the true {(qh, w, − log(Pr(w|h)), nhw) : qwh ∈ Q} path.
Details
-
File Typepdf
-
Upload Time-
-
Content LanguagesEnglish
-
Upload UserAnonymous/Not logged-in
-
File Pages33 Page
-
File Size-