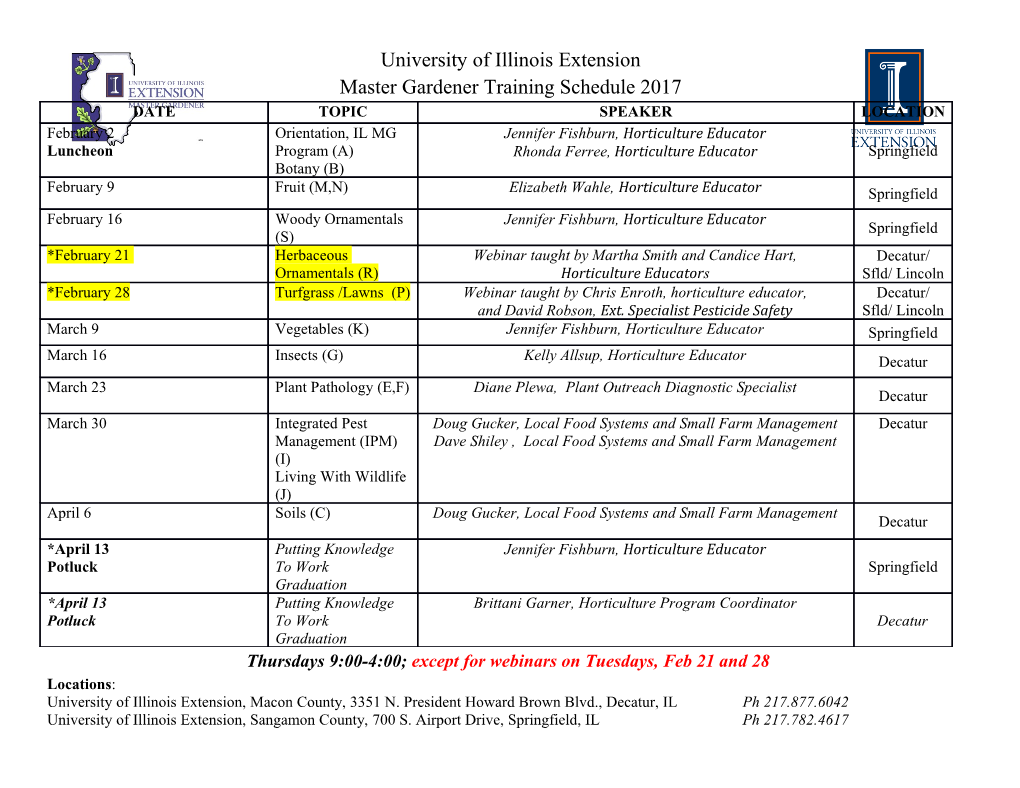
Deep Probabilistic Regression of Elements of SO(3) using Quaternion Averaging and Uncertainty Injection Valentin Peretroukhin, Brandon Wagstaff, and Jonathan Kelly University of Toronto [email protected], [email protected], [email protected] Abstract HydraNet q1 <latexit sha1_base64="anqzf7dHa0EqnrCL0OfX9zzF89g=">AAACK3icjVDLSsNAFJ34tj6rSzeDRXBjSFRQdwU3bgQFq0JTys301g5OHp25kZaQ33CrP+DXuFLc+h9O0y5UXHhg4Mw598UJUyUNed6bMzU9Mzs3v7BYWVpeWV1br25cmyTTAhsiUYm+DcGgkjE2 SJLC21QjRKHCm/D+dOTfPKA2MomvaJhiK4K7WHalALJSEJwDaTnI+0Xbb6/XPPekBB+To8MJOfG573olamyCi3bVWQw6icgijEkoMKbpeym1ctAkhcKiEmQGUxD3cIdNS2OI0LTy8uiC71ilw7uJti8mXqrfO3KIjBlGoa2MgHrmtzcS//KaGXWPW7mM04wwFuNF3Uxx SvgoAd6RGgWpoSUgtLS3ctEDDYJsTj+2DFLQBgteCcppeT+TNss9BYSDPdNLNImMjGt/xf/Cu953/QPXuzys1b1JjAtsi22zXeazI1ZnZ+yCNZhgKXtkT+zZeXFenXfnY1w65Ux6NtkPOJ9fXsupUA==</latexit> SO(3) Consistent estimates of rotation are crucial to vision- q2 <latexit sha1_base64="X7QWZITLf+jirqY71Mk1DFiYv6Q=">AAACLXicbVDLSgMxFM34rPWtSzfBIrixzKig7gQXuhBUtK3QVsmktzaYmYzJjbQM/Q+3+gN+jQtB3PobptNBfB0InJxzX5wwkcKg7796I6Nj4xOThani9Mzs3PzC4lLVKKs5VLiSSl+GzIAUMVRQoITLRAOLQgm18PZg4NfuQRuh4gvsJdCM2E0s2oIzdNJV41jAoVY2OT9Jt/rXCyW/7Gegf0mQkxLJcXq96E01WorbCGLkkhlTD/wEmynTKLiEfrFhDSSM37IbqDsaswhMM83O7tM1p7RoW2n3YqSZ+r0jZZExvSh0lRHDjvntDcT/vLrF9m4zFXFiEWI+XNS2kqKigwxoS2jgKHuOMK6Fu5XyDtOMo0vqx5ZuwrSBPi02smnpnRUuzQ3JELobpqM0coum7H7D8PYy0CHZ2c7JXvAVXnWzHGyV/bPt0r6fx1ggK2SVrJOA7JB9ckROSYVwoskDeSRP3rP34r1578PSES/vWSY/4H18AqPcqVc=</latexit> <latexit sha1_base64="h3mNhBeZiXCMiG0iPSkfppXmRYA=">AAACK3icjVDLSsNAFJ34tj6rSzeDRXBjSFRQdwU3bgQFq0JTys301g5OHp25kZaQ33CrP+DXuFLc+h9O0y5UXHhg4Mw598UJUyUNed6bMzU9Mzs3v7BYWVpeWV1br25cmyTTAhsiUYm+DcGgkjE2 SJLC21QjRKHCm/D+dOTfPKA2MomvaJhiK4K7WHalALJSEJwDaTnI+0V7v71e89yTEnxMjg4n5MTnvuuVqLEJLtpVZzHoJCKLMCahwJim76XUykGTFAqLSpAZTEHcwx02LY0hQtPKy6MLvmOVDu8m2r6YeKl+78ghMmYYhbYyAuqZ395I/MtrZtQ9buUyTjPCWIwXdTPF KeGjBHhHahSkhpaA0NLeykUPNAiyOf3YMkhBGyx4JSin5f1M2iz3FBAO9kwv0SQyMq79Ff8L73rf9Q9c7/KwVvcmMS6wLbbNdpnPjlidnbEL1mCCpeyRPbFn58V5dd6dj3HplDPp2WQ/4Hx+AWCHqVE=</latexit> based motion estimation in augmented reality and robotics. … q, Σq <latexit sha1_base64="tZdxszg9zZNK/NhmJnp5g+eeSCw=">AAACRXicbZDPThsxEMa9QFsI/RPaIxerKVIPJdptkQCpB6ReekEC0QSkbBTNOpPEwmtv7FmUaLUvwNNwpS/QZ+hDcKt6bZ3NCpW2n2Tp8zcej/1LMiUdheH3YGV17dHjJ+sbjc2nz56/aG697DqT W4EdYZSxFwk4VFJjhyQpvMgsQpooPE8uPy3q51donTT6C80z7Kcw1nIkBZCPBs038TGCLuIuCjK2mJblOx4fA1k5K+IzOU6hHEwHzVbYDivxf01UmxardTLYCjbioRF5ipqEAud6UZhRvwBLUigsG3HuMANxCWPseashRdcvqu+UfMcnQz4y1i9NvEr/7CggdW6eJv5k CjRxf9cW4f9qvZxGB/1C6iwn1GI5aJQrToYv2PChtB6DmnsDwkr/Vi4mYEGQJ/hgyiwD67Dkjbi6rZjm0lPeVUA423UTY0nk5Np+V1bwDivxpdnfq81hdA+v+74dfWiHp3uto481xnW2zV6ztyxi++yIfWYnrMMEu2Y37JZ9Db4Fd8GP4Ofy6EpQ97xiDxT8+g2w9rNf </latexit> qh In this work, we present a method to extract probabilistic <latexit sha1_base64="KUVseSJeBDABueVivQQqnZmSEw4=">AAACK3icjVDLSsNAFJ34tj6rSzeDRXBjSFRQdwU3bgQFq0JTys301g5OHp25kZaQ33CrP+DXuFLc+h9O0y5UXHhg4Mw598UJUyUNed6bMzU9Mzs3v7BYWVpeWV1br25cmyTTAhsiUYm+DcGgkjE2 SJLC21QjRKHCm/D+dOTfPKA2MomvaJhiK4K7WHalALJSEJwDaTnI+0W7116vee5JCT4mR4cTcuJz3/VK1NgEF+2qsxh0EpFFGJNQYEzT91Jq5aBJCoVFJcgMpiDu4Q6blsYQoWnl5dEF37FKh3cTbV9MvFS/d+QQGTOMQlsZAfXMb28k/uU1M+oet3IZpxlhLMaLupni lPBRArwjNQpSQ0tAaGlv5aIHGgTZnH5sGaSgDRa8EpTT8n4mbZZ7CggHe6aXaBIZGdf+iv+Fd73v+geud3lYq3uTGBfYFttmu8xnR6zOztgFazDBUvbIntiz8+K8Ou/Ox7h0ypn0bLIfcD6/AL4vqYc=</latexit> estimates of rotation from deep regression models. First, Σ <latexit sha1_base64="fBF9yLlyYOeEEMmZny3OW4GfbXw=">AAACMHicjVDLSitBFOzxbfRq1KWbxiC4cZi5V1B3ghs3gqJRIRPCmc5J0tjzuN2nJWHIl7jVH/BrdCVu/Qo7kyxUXFjQUF11XlScK2koCF68qemZ2bn5hcXK0vKfldXq2vqVyawWWBeZyvRNDAaV TLFOkhTe5BohiRVex7fHI//6DrWRWXpJgxybCXRT2ZECyEmt6mp0CqRlv4guZDeBYataC/zDEnxM9vcm5DDkoR+UqLEJzlpr3mLUzoRNMCWhwJhGGOTULECTFAqHlcgazEHcQhcbjqaQoGkW5eVDvu2UNu9k2r2UeKl+7iggMWaQxK4yAeqZ795I/MlrWOocNAuZ5pYw FeNFHas4ZXwUA29LjYLUwBEQWrpbueiBBkEurC9b+jlog0NeicppxX8rXaC7Cgj7u6aXaRKWjO9+vwzv6q8f/vOD873aUTCJcYFtsi22w0K2z47YCTtjdSaYZffsgT16T96z9+q9jUunvEnPBvsC7/0Dm/Sq6w==</latexit> T?, Σ? we build on prior work and develop a multi-headed net- <latexit sha1_base64="NWYXWbGgVEsdc5qIXsxaq7W7mwQ=">AAACSHicbVDLbtNAFB0bCm2gkJYlmxERgkVj2SVSW6mLSmy6QSqiaSrFUXQ9uUlGGT+Yua4SWf4Evqbb9gf6B/0Ldqi7ThyDaOFII5055z5mTpQpacj3bx33ydO1Z8/XNxovXm6+et3c2j4zaa4F dkWqUn0egUElE+ySJIXnmUaII4W9aPZ56fcuUBuZJqe0yHAQwySRYymArDRsfgi/AGk5L07LYWgI9A7/rYTf5CSGWh42W77nV+D/kqAmLVbjZLjlbISjVOQxJiQUGNMP/IwGBWiSQmHZCHODGYgZTLBvaQIxmkFR/ajk760y4uNU25MQr9S/OwqIjVnEka2MgabmsbcU /+f1cxrvDwqZZDlhIlaLxrnilPJlPHwkNQpSC0tAaGnfysUUNAiyIT7YMs9AGyx5I6ymFd9zaYNuKyCct8001SRyMp69lVV4BxX4iux1anIQ/AnvbNcLPnn+107r6LCOcZ29Ze/YRxawPXbEjtkJ6zLBfrBLdsWunRvnp/PLuVuVuk7d84Y9gOveA1DUs6g=</latexi t> work structure we name HydraNet that can account for both Fused estimate Sensor data aleatoric and epistemic uncertainty. Second, we extend Hy- T, ΣT draNet to targets that belong to the rotation group, SO(3), <latexit sha1_base64="XoJsl6Acp9wI3OH7J/V/yM7Kucw=">AAACRXicbVBNT9tAEF1Dy0dKIcCxl1VDJQ4lsgEJkDggcekFiaoJIMVRNN5MklXWa7M7Roks/wF+DVf6B/ob+iN6q3ptN45blZYnrfTmvZmd3RelSlry/a/ewuKLl0vLK6u1V2uv1zfqm1tXNsmM wLZIVGJuIrCopMY2SVJ4kxqEOFJ4HY3PZ/71HRorE92iaYrdGIZaDqQAclKvvhNeIOg8vAAycpK3iuI9/12En+QwhqLX6tUbftMvwf8nQUUarMJlb9NbDfuJyGLUJBRY2wn8lLo5GJJCYVELM4spiDEMseOohhhtNy+/U/B3TunzQWLc0cRL9e+JHGJrp3HkOmOgkf3X m4nPeZ2MBsfdXOo0I9RivmiQKU4Jn2XD+9KgIDV1BISR7q1cjMCAIJfgky2TFIzFgtfC8rb8NpMu5T0FhJM9O0oMiYxs01VFGd5JCT4nR4cVOQn+hHe13wwOmv7Hw8bZaRXjCnvD3rJdFrAjdsY+sEvWZoLdswf2yD57X7xv3nfvx7x1watmttkTeD9/AU2Psyc=</la texit> SE(3) by regressing unit quaternions and using the tools of ro- Classical odometry <latexit sha1_base64="Hq8ax/xU8L8MmvyBsM+jfgLwLTU=">AAACLXicbVDLSgMxFM34rPWtSzfBIrixzKig7gQRXbhQtK3QVsmktzaYmYzJjbQM/Q+3+gN+jQtB3PobptNBfB0InJxzX5wwkcKg7796I6Nj4xOThani9Mzs3PzC4lLVKKs5VLiSSl+GzIAUMVRQoITLRAOLQgm18PZg4NfuQRuh4gvsJdCM2E0s2oIzdNJV40TAkVY2OT9Mt/rXCyW/7Gegf0mQkxLJcXq96E01WorbCGLkkhlTD/wEmynTKLiEfrFhDSSM37IbqDsaswhMM83O7tM1p7RoW2n3YqSZ+r0jZZExvSh0lRHDjvntDcT/vLrF9m4zFXFiEWI+XNS2kqKigwxoS2jgKHuOMK6Fu5XyDtOMo0vqx5ZuwrSBPi02smnpnRUuzQ3JELobpqM0coum7H7D8PYy0CHZ2c7JXvAVXnWzHGyV/bPt0r6fx1ggK2SVrJOA7JB9ckxOSYVwoskDeSRP3rP34r1578PSES/vWSY/4H18ApJmqU0=</latexit> tation averaging and uncertainty injection onto the mani- fold to produce three-dimensional covariances. Finally, we Figure 1: Our model outputs a probabilistic representation present results and analysis on a synthetic dataset, learn of rotation as a unit quaternion and a full covariance ma- consistent orientation estimates on the 7-Scenes dataset, trix. A consistent uncertainty estimate allows us to improve and show how we can use our learned covariances to classical estimators using pose-graph optimization. fuse deep estimates of relative orientation with classical stereo visual odometry to improve localization on the KITTI less, the representational power of deep regression algo- dataset. rithms makes them an attractive option to complement clas- sical motion estimation when these latter methods perform poorly (e.g., under diverse lighting conditions or low scene 1. Introduction texture). By endowing deep regression models with a useful notion of uncertainty, we can account for out-of-training- Accounting for position and orientation, or pose, is at the distribution errors (a critical step to ensure safe operation) heart of many computer vision algorithms like visual odom- and fuse these models with classical methods using proba- etry, structure from motion, and SLAM. By tracking mo- bilistic factor graphs. In this work, we choose to focus on tion, these algorithms form the basis of visual localization rotation regression, since many motion algorithms are sen- pipelines in autonomous ground and aerial vehicles, visual sitive to rotation errors [18], and good rotation initializa- mapping, and augmented reality. tions can be critical to robust optimization [3]. Our novel Recent work [4, 15, 12] has attempted to transfer the suc- contributions are cess of deep neural networks in many areas of computer 1. a deep network structure we call HydraNet that builds vision to the task of camera pose estimation. These ap- on prior work [14, 17, 16] to build covariance matrices proaches, however, can produce arbitrarily poor pose esti- that account for both epistemic and aleatoric uncer- mates if sensor data differs from what is observed during tainty [13], training (i.e., it is ‘out of training distribution’) and their monolithic nature makes them difficult to debug. Further, 2. a formulation that extends HydraNet to means and co- despite much research effort, classical motion estimation variances defined on the rotation group SO(3), algorithms, like stereo visual odometry, still achieve state- of-the-art performance in nominal conditions1. Neverthe- 3. and open-source code for SO(3) regression2. 1Based on the KITTI odometry leaderboard [6] at the time of writing. 2https://github.com/utiasSTARS/so3_learning 432183 2. Approach where Log (·), represents the capitalized matrix logarithm · [19], and F the Frobenius norm. In the context of Equa- 2.1. HydraNet tion (1), using the angular metric leads to the Karcher mean, To endow deep networks with uncertainty, we extend which requires an iterative solver and has no known analytic prior work on multi-headed networks [17] and ensembles of expression. Applying the chordal metric leads to an analytic networks trained through bootstrapped training sets [14] to expression for the average but requires the use of Singular a single network structure we call HydraNet (see Figure 1). Value Decomposition. Using the quaternionic metric, how- HydraNet is composed of
Details
-
File Typepdf
-
Upload Time-
-
Content LanguagesEnglish
-
Upload UserAnonymous/Not logged-in
-
File Pages4 Page
-
File Size-