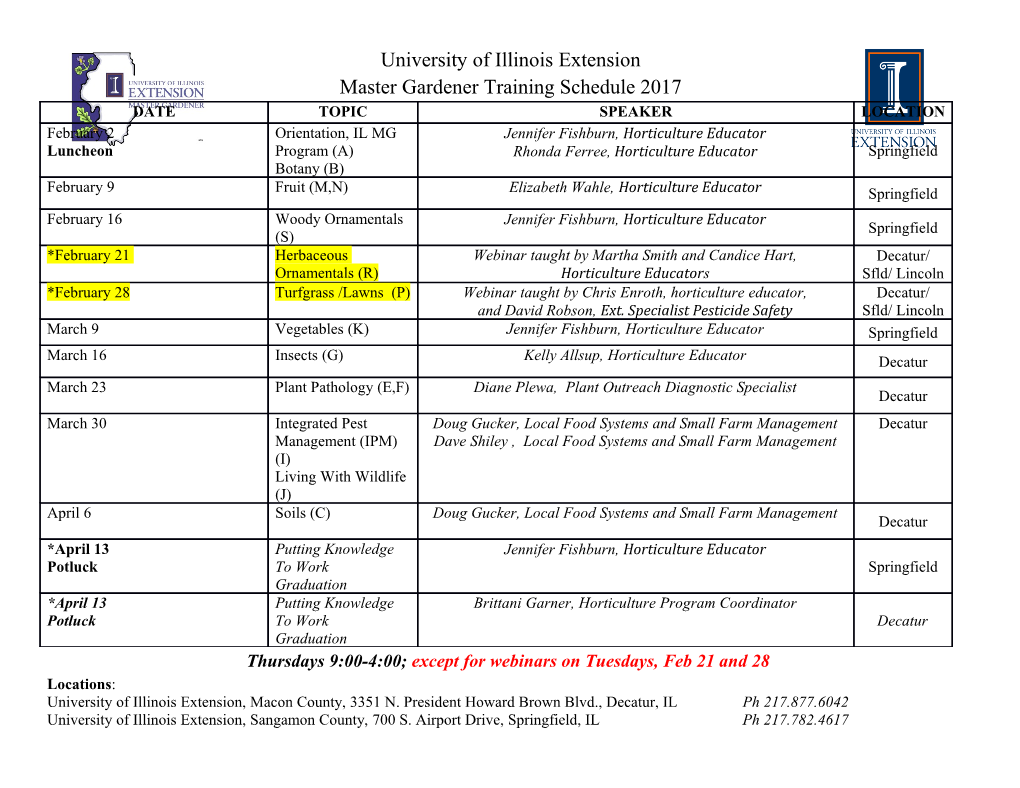
Statistics 203: Introduction to Regression and Analysis of Variance Fixed vs. Random Effects Jonathan Taylor - p. 1/19 Today’s class ● Today’s class ■ Random effects. ● Two-way ANOVA ● Random vs. fixed effects ■ ● When to use random effects? One-way random effects ANOVA. ● Example: sodium content in ■ beer Two-way mixed & random effects ANOVA. ● One-way random effects model ■ ● Implications for model Sattherwaite’s procedure. ● One-way random ANOVA table ● Inference for µ· ● 2 Estimating σµ ● Example: productivity study ● Two-way random effects model ● ANOVA tables: Two-way (random) ● Mixed effects model ● Two-way mixed effects model ● ANOVA tables: Two-way (mixed) ● Confidence intervals for variances ● Sattherwaite’s procedure - p. 2/19 Two-way ANOVA ● Today’s class ■ Second generalization: more than one grouping variable. ● Two-way ANOVA ● Random vs. fixed effects ■ ● When to use random effects? Two-way ANOVA model: observations: ● Example: sodium content in ≤ ≤ ≤ ≤ ≤ ≤ beer (Yijk); 1 i r; 1 j m; 1 k nij : r groups in first ● One-way random effects model grouping variable, m groups ins second and nij samples in ● Implications for model ● One-way random ANOVA (i; j)-“cell”: table ● Inference for µ· 2 ● 2 Estimating σµ Yijk = µ + αi + βj + (αβ)ij + "ijk; "ijk ∼ N(0; σ ): ● Example: productivity study ● Two-way random effects ■ model Constraints: ● ANOVA tables: Two-way ◆ r (random) αi = 0 ● i=1 Mixed effects model ◆ Pm ● Two-way mixed effects model j=1 βj = 0 ● ANOVA tables: Two-way Pm (mixed) ◆ (αβ) = 0; 1 ≤ i ≤ r ● Confidence intervals for j=1 ij variances ◆ Pr ● Sattherwaite’s procedure (αβ)ij = 0; 1 ≤ j ≤ m: Pi=1 - p. 3/19 Random vs. fixed effects ● Today’s class ■ In ANOVA examples we have seen so far, the categorical ● Two-way ANOVA ● Random vs. fixed effects variables are well-defined categories: below average fitness, ● When to use random effects? ● Example: sodium content in long duration, etc. beer ● One-way random effects ■ model In some designs, the categorical variable is “subject”. ● Implications for model ● One-way random ANOVA ■ Simplest example: repeated measures, where more than table ● Inference for µ· one (identical) measurement is taken on the same individual. ● Estimating σ2 µ ■ ● Example: productivity study In this case, the “group” effect αi is best thought of as ● Two-way random effects model random because we only sample a subset of the entire ● ANOVA tables: Two-way (random) population of subjects. ● Mixed effects model ● Two-way mixed effects model ● ANOVA tables: Two-way (mixed) ● Confidence intervals for variances ● Sattherwaite’s procedure - p. 4/19 When to use random effects? ● Today’s class ■ A “group” effect is random if we can think of the levels we ● Two-way ANOVA ● Random vs. fixed effects observe in that group to be samples from a larger population. ● When to use random effects? ● Example: sodium content in ■ beer Example: if collecting data from different medical centers, ● One-way random effects model “center” might be thought of as random. ● Implications for model ● One-way random ANOVA ■ Example: if surveying students on different campuses, table ● Inference for µ· “campus” may be a random effect. ● 2 Estimating σµ ● Example: productivity study ● Two-way random effects model ● ANOVA tables: Two-way (random) ● Mixed effects model ● Two-way mixed effects model ● ANOVA tables: Two-way (mixed) ● Confidence intervals for variances ● Sattherwaite’s procedure - p. 5/19 Example: sodium content in beer ● Today’s class ■ How much sodium is there in North American beer? How ● Two-way ANOVA ● Random vs. fixed effects much does this vary by brand? ● When to use random effects? ● Example: sodium content in ■ beer Observations: for 6 brands of beer, researchers recorded the ● One-way random effects model sodium content of 8 12 ounce bottles. ● Implications for model ● One-way random ANOVA ■ Questions of interest: what is the “grand mean” sodium table ● Inference for µ· content? How much variability is there from brand to brand? ● Estimating σ2 µ ■ ● Example: productivity study “Individuals” in this case are brands, repeated measures are ● Two-way random effects model the 8 bottles. ● ANOVA tables: Two-way (random) ● Mixed effects model ● Two-way mixed effects model ● ANOVA tables: Two-way (mixed) ● Confidence intervals for variances ● Sattherwaite’s procedure - p. 6/19 One-way random effects model ● Today’s class ■ Suppose we take n identical measurements from r subjects. ● Two-way ANOVA ● Random vs. fixed effects ■ ● When to use random effects? Yij ∼ µ· + αi + "ij ; 1 ≤ i ≤ r; 1 ≤ j ≤ n ● Example: sodium content in ■ 2 beer " ∼ N(0; σ ); 1 ≤ i ≤ r; 1 ≤ j ≤ n ● One-way random effects ij model ■ 2 ● Implications for model αi ∼ N(0; σµ); 1 ≤ i ≤ r: ● One-way random ANOVA table ■ ● Inference for µ· We might be interested in the population mean, µ·: CIs, is it ● 2 Estimating σµ zero? etc. ● Example: productivity study ● Two-way random effects ■ Alternatively, we might be interested in the variability across model ● ANOVA tables: Two-way subjects, 2 : CIs, is it zero? (random) σµ ● Mixed effects model ● Two-way mixed effects model ● ANOVA tables: Two-way (mixed) ● Confidence intervals for variances ● Sattherwaite’s procedure - p. 7/19 Implications for model ● Today’s class ■ In random effects model, the observations are no longer ● Two-way ANOVA ● Random vs. fixed effects independent (even if "’s are independent). In fact ● When to use random effects? ● Example: sodium content in beer 0 0 2 0 2 0 ● One-way random effects Cov(Yij; Yi j ) = σµδi;i + σ δj;j : model ● Implications for model ■ ● One-way random ANOVA In more complicated mixed effects models, this makes MLE table ● Inference for µ· more complicated: not only are there parameters in the ● 2 Estimating σµ ● Example: productivity study mean, but in the covariance as well. ● Two-way random effects ■ model In ordinary least squares regression, the only parameter to ● ANOVA tables: Two-way 2 2 (random) estimate is σ because the covariance matrix is σ I. ● Mixed effects model ● Two-way mixed effects model ● ANOVA tables: Two-way (mixed) ● Confidence intervals for variances ● Sattherwaite’s procedure - p. 8/19 One-way random ANOVA table ● Today’s class Source SS df E(MS) ● Two-way ANOVA r 2 Treatments SST R = n Y · − Y ·· r − 1 σ2 + nσ2 ● Random vs. fixed effects Pi=1 “ i ” µ ● When to use random effects? r n − 2 − 2 Error SSE = i=1 j=1(Yij Y i·) (n 1)r σ ● P P Example: sodium content in ■ beer Only change here is the expectation of SST R which reflects ● One-way random effects model randomness of α ’s. ● Implications for model i ● One-way random ANOVA ■ table ANOVA table is still useful to setup tests: the same F ● Inference for µ· ● 2 statistics for fixed or random will work here. Estimating σµ ● Example: productivity study ■ 2 ● Two-way random effects Under H0 : σµ = 0, it is easy to see that model ● ANOVA tables: Two-way (random) MST R ● Mixed effects model ∼ Fr−1;(n−1)r: ● Two-way mixed effects model MSE ● ANOVA tables: Two-way (mixed) ● Confidence intervals for variances ● Sattherwaite’s procedure - p. 9/19 Inference for µ· ● Today’s class ■ ● Two-way ANOVA We know that E(Y ··) = µ·, and can show that ● Random vs. fixed effects ● When to use random effects? 2 2 ● Example: sodium content in nσµ + σ beer Var(Y ··) = : ● One-way random effects rn model ● Implications for model ■ ● One-way random ANOVA Therefore, table ● Inference for µ· Y ·· − µ· ● 2 Estimating σµ ∼ tr−1 ● Example: productivity study SST R ● Two-way random effects q (r−1)rn model ● ANOVA tables: Two-way ■ (random) Why r − 1 degrees of freedom? Imagine we could record an ● Mixed effects model ● Two-way mixed effects model infinite number of observations for each individual, so that ● ANOVA tables: Two-way (mixed) Y i· ! µi. ● Confidence intervals for variances ■ · ● Sattherwaite’s procedure To learn anything about µ we still only have r observations (µ1; : : : ; µr). ■ Sampling more within an individual cannot narrow the CI for µ·. - p. 10/19 2 Estimating σµ ● Today’s class ■ From the ANOVA table ● Two-way ANOVA ● Random vs. fixed effects ● When to use random effects? 2 E(SST R=(r − 1)) − E(SSE=((n − 1)r)) ● Example: sodium content in σ = : beer µ ● One-way random effects n model ● Implications for model ■ Natural estimate: ● One-way random ANOVA table ● Inference for µ· 2 SST R=(r − 1) − SSE=((n − 1)r) ● 2 Estimating σµ Sµ = ● Example: productivity study n ● Two-way random effects model ■ ● ANOVA tables: Two-way Problem: this estimate can be negative! One of the (random) ● Mixed effects model difficulties in random effects model. ● Two-way mixed effects model ● ANOVA tables: Two-way (mixed) ● Confidence intervals for variances ● Sattherwaite’s procedure - p. 11/19 Example: productivity study ● Today’s class ■ Imagine a study on the productivity of employees in a large ● Two-way ANOVA ● Random vs. fixed effects manufacturing company. ● When to use random effects? ● Example: sodium content in ■ beer Company wants to get an idea of daily productivity, and how ● One-way random effects model it depends on which machine an employee uses. ● Implications for model ● ■ One-way random ANOVA Study: take m employees and r machines, having each table ● Inference for µ· employee work on each machine for a total of n days. ● Estimating σ2 µ ■ ● Example: productivity study As these employees are not all employees, and these ● Two-way random effects model machines are not all machines it makes sense to think of ● ANOVA tables: Two-way (random) both the effects of machine and employees (and ● Mixed effects model ● Two-way mixed effects model interactions) as random. ● ANOVA tables: Two-way (mixed) ● Confidence intervals for variances ● Sattherwaite’s procedure - p.
Details
-
File Typepdf
-
Upload Time-
-
Content LanguagesEnglish
-
Upload UserAnonymous/Not logged-in
-
File Pages19 Page
-
File Size-