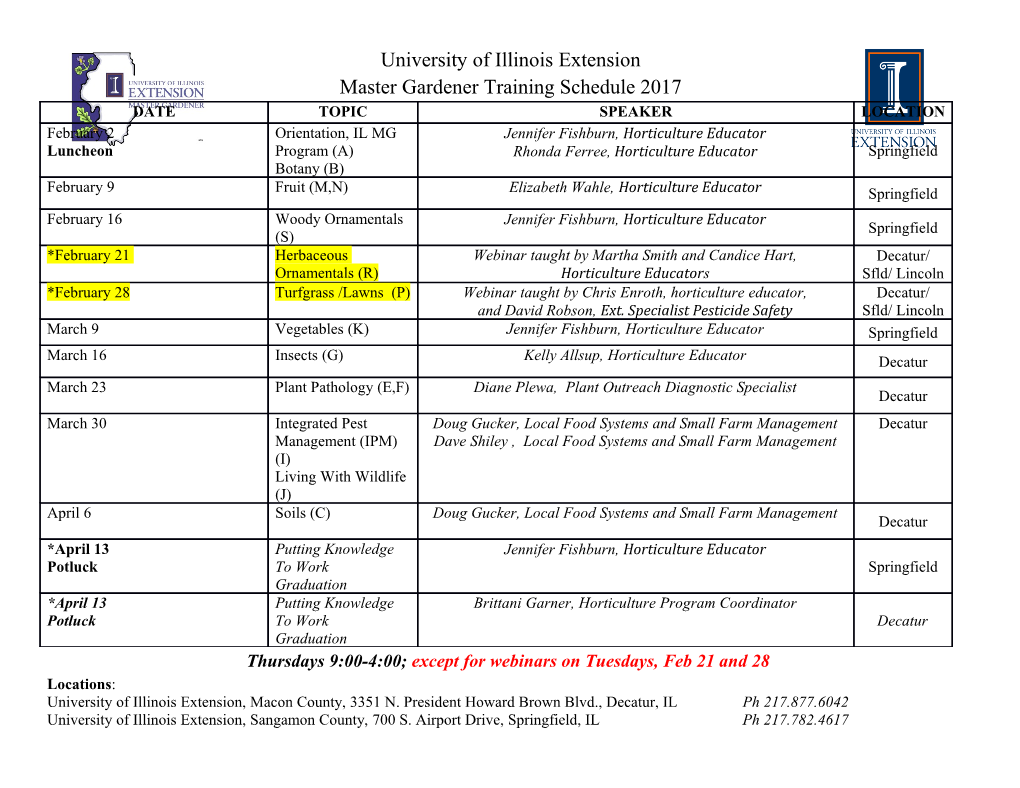
Arthur CHARPENTIER - Multivariate Distributions Multivariate Distributions: A brief overview (Spherical/Elliptical Distributions, Distributions on the Simplex & Copulas) A. Charpentier (Université de Rennes 1 & UQàM) Université de Rennes 1 Workshop, November 2015. http://freakonometrics.hypotheses.org @freakonometrics 1 Arthur CHARPENTIER - Multivariate Distributions Geometry in Rd and Statistics T P The standard inner product is < x, y >`2 = x y = i xiyi. Hence, x ⊥ y if < x, y >`2 = 0. 1 1 The Euclidean norm is kxk =< x, x > 2 = Pn x2 2 . `2 `2 i=1 i d d The unit sphere of R is Sd = {x ∈ R : kxk`2 = 1}. If x = {x1, ··· , xn}, note that the empirical covariance is Cov(x, y) =< x − x, y − y >`2 and Var(x) = kx − xk`2 . T For the (multivariate) linear model, yi = β0 + β1 xi + εi, or equivalently, yi = β0+ < β1, xi >`2 +εi @freakonometrics 2 Arthur CHARPENTIER - Multivariate Distributions The d dimensional Gaussian Random Vector T If Z ∼ N (0, I), then X = AZ + µ ∼ N (µ, Σ) where Σ = AA . Conversely (Cholesky decomposition), if X ∼ N (µ, Σ), then X = LZ + µ for T 1 some lower triangular matrix L satisfying Σ = LL . Denote L = Σ 2 . With Cholesky decomposition, we have the particular case (with a Gaussian distribution) of Rosenblatt (1952)’s chain, f(x1, x2, ··· , xd) = f1(x1) · f2|1(x2|x1) · f3|2,1(x3|x2, x1) ··· ··· fd|d−1,··· ,2,1(xd|xd−1, ··· , x2, x1). 1 1 T −1 d f(x; µ, Σ) = d 1 exp − (x − µ) Σ (x − µ) for all x ∈ R . (2π) 2 |Σ| 2 2 | {z } kxkµ,Σ @freakonometrics 3 Arthur CHARPENTIER - Multivariate Distributions The d dimensional Gaussian Random Vector T −1 Note that kxkµ,Σ = (x − µ) Σ (x − µ) is the Mahalanobis distance. d Define the ellipsoid Eµ,Σ = {x ∈ R : kxkµ,Σ = 1} Let X1 µ1 Σ11 Σ12 X = ∼ N , X2 µ2 Σ21 Σ22 then −1 −1 X1|X2 = x2 ∼ N (µ1 + Σ12Σ22 (x2 − µ2) , Σ11 − Σ12Σ22 Σ21) X1 ⊥⊥ X2 if and only if Σ12 = 0. Further, if X ∼ N (µ, Σ), then AX + b ∼ N (Aµ + b, AΣAT). @freakonometrics 4 Arthur CHARPENTIER - Multivariate Distributions The Gaussian Distribution, as a Spherical Distributions If X ∼ N (0, I), then X = R · U, where 2 2 2 1 R = kXk`2 ∼ χ (d) 0 ● −1 and −2 −2 −1 −2 0 −1 U = X/kXk ∼ U(S ), 1 `2 d 0 1 2 2 with R ⊥⊥ U. @freakonometrics 5 Arthur CHARPENTIER - Multivariate Distributions The Gaussian Distribution, as an Elliptical Distributions 1 If X ∼ N (µ, Σ), then X = µ + R · Σ 2 · U, where | {z } 2 2 2 1 R = kXk`2 ∼ χ (d) 0 ● −1 −2 −2 and −1 −2 0 −1 U = X/kXk ∼ U(S ), 0 1 `2 d 1 2 2 with R ⊥⊥ U. @freakonometrics 6 Arthur CHARPENTIER - Multivariate Distributions Spherical Distributions T T Let M denote an orthogonal matrix, M M = MM = I. X has a spherical distribution if X =L MX. E.g. in R2, cos(θ) − sin(θ) X1 L X1 = sin(θ) cos(θ) X2 X2 d T L For every a ∈ R , a X = kak`2 Yi for any i ∈ {1, ··· , d}. Further, the generating function of X can be written T [eit X ] = ϕ(tTt) = ϕ(ktk2 ), ∀t ∈ d, E `2 R for some ϕ : R+ → R+. @freakonometrics 7 Arthur CHARPENTIER - Multivariate Distributions Uniform Distribution on the Sphere Actually, more complex that it seems... x1 = ρ sin ϕ cos θ x2 = ρ sin ϕ sin θ x3 = ρ cos ϕ with ρ > 0, ϕ ∈ [0, 2π] and θ ∈ [0, π]. If Φ ∼ U([0, 2π]) and Θ ∼ U([0, π]), we do not have a uniform distribution on the sphere... see https://en.wikibooks.org/wiki/Mathematica/Uniform_Spherical_Distribution, http://freakonometrics.hypotheses.org/10355 @freakonometrics 8 Arthur CHARPENTIER - Multivariate Distributions Spherical Distributions ● ● ● ● 2 ● ● ● ● ● ● ● ● ● ● ● ● ● ● ● ● ● ● ● ● ● ● ● ● ● ● ● ●● ●●● ●●● ● ● 1 ●● ●● ● ●●● ●●● ●●● ● ● ● ● ● ● ● ● ● ●●●● ● ● ● ● ●● ● ● ●● ● ●● ●●● ● ● ● ● ● ● ● ● ● ●● ● ● ● ● ●● ● ● ● ● ● ● ● ●● ● ● ● ● ● ● ● ● ● Random vector X as a spherical distribution if ● ●● ● ● ● ● ● ● ●● ● ● ● ● ● ● ● ● ● ● ● ● ● ● ● ● ● ● ● 0 ● ●● ● ● ● ● ● ● ● ● ● ● ● ● ● ● ● ● ● ● ● ● ● ● ● ● ● ● ● ● ● ● ● ● ● ● ● ● ● ● ● ● ● ● ●● ● ● ● ●● ● ●● ● ● ●● ● ● ● ● ● ● ● ●● ● ● ●● ● ● ●● ● ●● ●● ● ● ●● ● ●●● ● ● ●● ●● ● ● ● ● ● ●●●● ● ● ● ● ● ● ● ● ● ●●●● ● ● ●●● ● ● ●●● ● ● ●● ● ●● ●● ● ● ● ●●●●●● ●●●●● ● ● ● ●●●●●●●● ● ● ● ● ● ● ● ● −1 ● ● ● ● ● ● ● ● ● ● ● ● ● ● X = R · U ● ● ● ● −2 ● ● ● −2 −1 0 1 2 where R is a positive random variable and U is uniformly d distributed on the unit sphere of R , Sd, with R ⊥⊥ U ● ● ● 0.02 ● 2 ● ● ● 0.04 ● ● ● ● ● ● ● ● ● ● ● ● ● ● ● ● ● 0.08 ● ● ● ● ● ● ● ● 1 ● ● ● ● ● ● ● ● ● ● ● ● ● ● ● ● ● ● ● 0.14 ● ● ●● ● ● ● ● ● ● ● ● ● ● ● ● ●● ● ● ● ● ● ● ● ● ● ● ● ● ● ● ● ● 0 ● ● ● ● ● ● ● ● ● ● ● ● ● ● ● ● ● ● ● ● ● ● ● ● ● ● ● ● ● ● ● ● ● ● ● ● ●● ● ● ● ● ● ● ● ● ● ● ● ●● ● ● ● ● ● ● ● ● ● ● ●0.12 ● ● ● ● ● ● ● ● ● ● ●● ● ● ● ● ● ● ● ● ● ● ● ● ● ● ● ● ● ● E.g. X ∼ N (0, ). −1 ● ● ● ● ● ● I ● ● 0.06 ● ● ● ● ● ● ● ● ● ● −2 ● ● ● −2 −1 0 1 2 @freakonometrics 9 Arthur CHARPENTIER - Multivariate Distributions Elliptical Distributions 2 ● ● ● ● ● ● ● ● ● ● ● ● ● ● ● ● ● ● ● ● ● 1 ● ● ● ● ● ● ● ● ●●●● ●● ● ● ●●● ●●●● ● ● ● ● ● ●●● ● ● ●● ● ●● ● ● ● ●●● ● ●●● ● ● ● ●● ● ● ● ●●●● ● ● ● ● ● ●● ● ● ● ●● ●● ● ● ●● ● ● ● ● Random vector X as a elliptical distribution if ●● ● ● ● ● ● ● ● ● ● ● ● ●● ● ● ● ● ●● ● ●● ● ● ● ● ●● ● ● ● ●● ● ● ● ● ● ● ● ● ● ● ● ● ● ● ● ● ●● ● ● ● 0 ● ● ● ● ● ● ● ● ● ●● ● ● ● ● ●● ● ● ● ● ● ● ● ● ● ● ● ● ● ● ● ●● ● ● ● ● ● ● ● ● ● ●● ● ● ● ● ● ●● ● ● ● ● ● ● ●● ● ● ● ● ● ● ● ● ● ● ●● ● ● ● ●●● ● ● ●● ● ● ● ●●●●● ● ● ● ● ● ● ●●●● ● ● ●● ● ● ● ● ● ●●● ● ● ●● ● ●● ●●●● ●● ● ● ● ●●● ●● ● ●●●●●● ● ● ●● ●●●●● ● ● ● ● ● ● ● ● ● ● ● ● ● ● ● ● ● ● ● −1 ● ● ● ● ● ● ● ● X = µ + R · A · U ● ● −2 0 −2 −1 0 1 2 where A satisfies AA = Σ, U(Sd), with R ⊥⊥ U. Denote 1 Σ 2 = A. 2 ● ● ● ● 0.02 ● ● 0.04 ● ● ● ● ● 0.06● ● ● ● ● ● ● ● ● ● 1 ● ● ● ● ● ● ● ● ● ● ● ● 0.12● ● ● ● ● ● ● ● ● ● ● ● ● ● ● ● ● ● ● ● ● ● ● ● ● ● ● ● ●● ●● ● ● ● ●● ● ● ● ● ● ● ● ● ● ● ● ● ● ● ● ● ● ● ● ● ● 0 ● ● ● ● ● ● ● ● ● ● ● ● ● ● ● ● ● ● ● ● ● ● ● ● ● ● ● ● ● ● ● ● ● ● ● ● ● ● ● ● ● ● ● ● ● ● ● ● ● ● ● ● ● ● 0.14 ● ● ●● ● ● ● ● ● ● ● ● ● ● ● ● ● ● ● ● ● ● ● ● ● ● ● ● ● ● ● ● ● ● ● ● ● 0.08 ● ● ● ● ● ● E.g. X ∼ N (µ, Σ). −1 ● ● ● ● ● ● ● ● ● ● −2 −2 −1 0 1 2 @freakonometrics 10 Arthur CHARPENTIER - Multivariate Distributions Elliptical Distributions 1 X = µ + RΣ 2 U where R is a positive random variable, U ∼ U(Sd), with U ⊥⊥ R. If X ∼ FR, then X ∼ E(µ, Σ,FR). Remark Instead of FR it is more common to use ϕ such that itTX itTµ T d E[e ] = e ϕ(t Σt), t ∈ R . 0 E[X] = µ and Var[X] = −2ϕ (0)Σ q 1 T −1 f(x) ∝ 1 f( (x − µ) Σ (x − µ)) |Σ| 2 where f : R+ → R+ is called radial density. Note that dF (r) ∝ rd−1f(r)1(x > 0). @freakonometrics 11 Arthur CHARPENTIER - Multivariate Distributions Elliptical Distributions If X ∼ E(µ, Σ,FR), then T AX + b ∼ E(Aµ + b, AΣA ,FR) If X1 µ1 Σ11 Σ12 X = ∼ E , ,FR X2 µ2 Σ21 Σ22 then −1 −1 X1|X2 = x2 ∼ E(µ1 + Σ12Σ22 (x2 − µ2) Σ11 − Σ12Σ22 Σ21,F1|2) where 2 1 F1|2 is the c.d.f. of (R − ?) 2 given X2 = x2. @freakonometrics 12 Arthur CHARPENTIER - Multivariate Distributions Mixtures of Normal Distributions Let Z ∼ N (0, I). Let W denote a positive random variable, Z ⊥⊥ W . Set √ 1 X = µ + W Σ 2 Z, so that X|W = w ∼ N (µ, wΣ). E[X] = µ and Var[X] = E[W ]Σ itTX itTµ− 1 W tTΣt) d E[e ] = E e 2 , t ∈ R . i.e. X ∼ E(µ, Σ, ϕ) where ϕ is the generating function of W , i.e. ϕ(t) = E[e−tW ]. If W has an inverse Gamma distribution, W ∼ IG(ν/2, ν/2), then X has a multivariate t distribution, with ν degrees of freedom. @freakonometrics 13 Arthur CHARPENTIER - Multivariate Distributions Multivariate Student t X ∼ t(µ, Σ, ν) if 1 Z X = µ + Σ 2 √ W /ν where Z ∼ N (0, I) and W ∼ χ2(ν), with Z ⊥⊥ W . Note that ν Var[X] = Σ if ν > 2. ν − 2 @freakonometrics 14 Arthur CHARPENTIER - Multivariate Distributions Multivariate Student t (r = 0.1, ν = 4), (r = 0.9, ν = 4), (r = 0.5, ν = 4) and (r = 0.5, ν = 10). @freakonometrics 15 Arthur CHARPENTIER - Multivariate Distributions On Conditional Independence, de Finetti & Hewitt Instead of X =L MX for any orthogonal matrix M, consider the equality for any permutation matrix M, i.e. L (X1, ··· ,Xd) = (Xσ(1), ··· ,Xσ(d)) for any permutation of {1, ··· , d} E.g. X ∼ N (0, Σ) with Σi,i = 1 and Σi,j = ρ when i 6= j. Note that necessarily 1 ρ = Corr(X ,X ) = − . i j d − 1 From de Finetti (1931), X1, ··· ,Xd, ··· are exchangeable {0, 1} variables if and only if there is a c.d.f. Π on [0, 1] such that Z 1 xT1 n−xT1 P[X = x] = θ [1 − θ] dΠ(θ), 0 i.e. X1, ··· ,Xd, ··· are (conditionnaly) independent given Θ ∼ Π. @freakonometrics 16 Arthur CHARPENTIER - Multivariate Distributions On Conditional Independence, de Finetti & Hewitt-Savage More generally, from Hewitt & Savage (1955) random variables X1, ··· ,Xd, ··· are exchangeable if and only if there is F such that X1, ··· ,Xd, ··· are (conditionnaly) independent given F. E.g. popular shared frailty models. Consider lifetimes T1, ··· ,Td, with Cox-type proportional hazard µi(t) = Θ · µi,0(t), so that θ P[Ti > t|Θ = θ] = F i,0(t) Assume that lifetimes are (conditionnaly) independent given Θ. @freakonometrics 17 Arthur CHARPENTIER - Multivariate Distributions d The Simplex Sd ⊂ R ( d ) d X Sd = x = (x1, x2, ··· , xd) ∈ R xi > 0, i = 1, 2, ··· , d; xi = 1 . i=1 Henre, the simplex here is the set of d-dimensional probability vectors. Note that d Sd = {x ∈ R+ : kxk`1 = 1} Remark Sometimes the simplex is ( d−1 ) ˜ d−1 X Sd−1 = x = (x1, x2, ··· , xd−1) ∈ R xi > 0, i = 1, 2, ··· , d; xi≤1 . i=1 T Note that if x˜ ∈ S˜d−1, then (x˜, 1 − x˜ 1) ∈ Sd. d If h : R+ → R+ is homogeneous of order 1, i.e. h(λx) = λ · h(x) for all λ > 0. Then x x h(x) = kxk`1 · h where ∈ Sd. kxk`1 kxk`1 @freakonometrics 18 Arthur CHARPENTIER - Multivariate Distributions Compositional Data and Geometry
Details
-
File Typepdf
-
Upload Time-
-
Content LanguagesEnglish
-
Upload UserAnonymous/Not logged-in
-
File Pages52 Page
-
File Size-