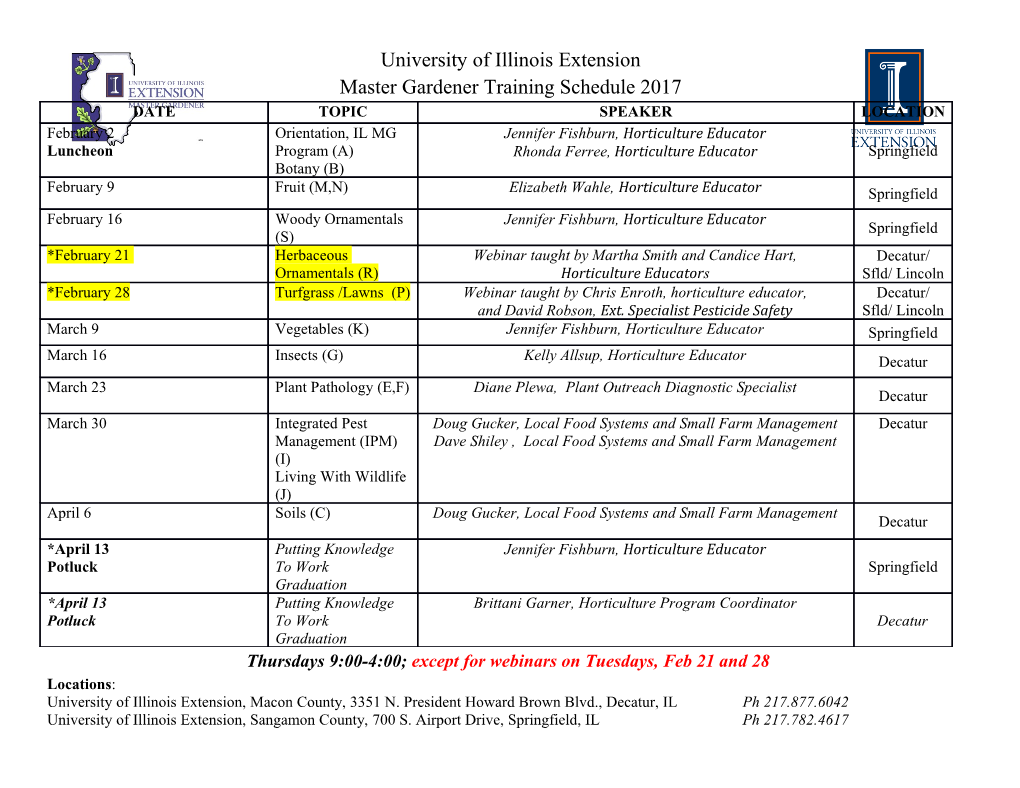
Masarykova univerzita Fakulta}w¡¢£¤¥¦§¨ informatiky !"#$%&'()+,-./012345<yA| Usability improvements of OptaPlanner Benchmarker Diploma thesis Bc. Matej Čimbora Brno, January 2015 Declaration Hereby I declare, that this paper is my original authorial work, which I have worked out by my own. All sources, references and literature used or excerpted during elaboration of this work are properly cited and listed in complete reference to the due source. Bc. Matej Čimbora Advisor: doc. RNDr. Tomáš Pitner, Ph.D. ii Acknowledgement I would like to thank doc. RNDr. Tomáš Pitner, Ph.D. and RNDr. Filip Nguyen for valuable advisory and comments. I owe special thanks to Geoffrey de Smet and Lukáš Petrovický for guidance and help during development process. iii Abstract This thesis is dedicated to improvements of OptaPlanner’s benchmark- ing functionality. Selected usability issues are presented, together with proposed solutions, while the main attention is paid on result persis- tence and comparison. In addition, a short introduction into constraint programming, together with an overview of the main features of Opta- Planner engine is included. iv Keywords OptaPlanner, benchmarking, constraint programming, optimization, Java v Contents 1 Introduction ...........................3 1.1 Goals .............................3 1.2 Structure ..........................4 2 Constraint programming ...................5 3 Configuration and basic usage ...............8 3.1 Modeling the problem ...................8 3.2 Solver configuration ..................... 10 3.2.1 Solution evaluation . 12 3.2.2 Termination . 15 3.3 Running the solver ..................... 15 4 Supported algorithms ..................... 17 4.1 Local search algorithms ................... 17 4.1.1 Hill climbing . 17 4.1.2 Simulated annealing . 18 4.1.3 Tabu search . 18 4.1.4 Late acceptance . 19 4.2 Construction heuristics ................... 19 4.2.1 First fit . 19 4.2.2 Weakest fit . 20 4.2.3 Allocate entity from Queue . 20 4.2.4 Cheapest insertion . 20 4.3 Other ............................ 20 5 Benchmarking ......................... 21 5.1 Report document ...................... 21 5.2 Configuration ........................ 23 6 Result persistence and recovery .............. 25 6.1 Result persistence ...................... 26 6.2 Resume functionality .................... 30 6.2.1 Usability issues . 33 7 Result aggregation ....................... 34 7.1 User interface ........................ 36 7.1.1 Benchmark selection area . 36 7.1.2 Menu bar . 38 7.1.3 Information panel . 39 7.1.4 Exception handling . 41 1 7.1.5 Real world usage . 42 8 Usage demonstration ..................... 43 8.1 Problem description ..................... 43 8.2 Scenario ........................... 43 8.3 Running the benchmarker ................. 44 8.4 Data persistence verification ................ 45 8.5 Result aggregation ..................... 45 9 Conclusion ............................ 50 9.1 Future work ......................... 50 A Source code locations ..................... 51 A.1 Result persistence and recovery .............. 51 A.2 Aggregator GUI ....................... 51 2 1 Introduction Resource allocation plays a vital role in business environment. Ability to allocate limited amount of resources, such as employees, or computa- tional power in an effective manner to meet company objectives is one of the factors, determining potential of its success. This area relates closely to problematics of constraint satisfaction, originating as a branch of ar- tificial intelligence, dating back to 1960s [18]. Rapid development of information technologies in the past decades enabled to tackle such problems utilizing computational resources, using specialized solvers. Popularity of the field is proved by numerous available products (e.g. OptaPlanner [12], IBM ILOG [7], Geocode [3], SICStus [14], Choco [1], etc.). Furthermore, the area of constraint programming has been in- cluded as a part of Java Specification Request (JSR 331)[9]. This thesis is dedicated to OptaPlanner, a lightweight, embeddable planning en- gine that optimizes planning problems[13]. It provides a way to solve the problems in a sophisticated way, combining constructing heuristic and metaheuristic algorithms with Java-based, or rule engine score cal- culation, while maintaining scalability of the solving process. There are numerous use case OptaPlanner is able to handle (e.g. Vehicle routing, Bin packing, Job shop sheduling, etc.). The product was formerly a part of Drools suite and was known as Drools Planner. As of version 6.0.0.Beta1 it exists as a standalone project, belonging to KIE group [10]. It is released under Apache Software License 2.0. 1.1 Goals Optimization of planning problems can be approached in different ways, depending on the type of algorithm used. OptaPlanner provides a fea- ture, which can ease related decision process, named benchmarker. Its purpose is to qualitatively compare how different solving algorithms perform on selected problems and provide comparison overview, incor- porating charts and various statistics. As the benchmarking process may take a long time to finish, a failure in the process, leading to loss of results is undesired. The first goal is to handle this use case by result persistence, enabling even partial results to be reused after the bench- marker crashes. The second goal relates closely to the aforementioned 3 1. Introduction persistence functionality. With the partial results of benchmarker pro- cess stored, it is possible to provide a tool for their further processing. This motivated creation of Swing-based application, allowing compar- ison of the results, originating even from different benchmarker pro- cesses. 1.2 Structure The first chapter Constraint programming briefly describes related field of constraint satisfaction, providing formal definitions and overview of basic terms. The following chapter Configuration and basic usage pro- vides description of the way OptaPlanner approaches constraint satis- faction problems. It covers solving procedure and the most notable con- figuration options. Chapter Supported algorithms contains an overview of majority of OptaPlanner’s heuristic and metaheuristic algorithms. The next chapter Benchmarking describes motivation of benchmarker usage, including examples of charts, generated as a part of its summary document. Result persistence and recovery and Result aggregation are chapters dedicated to newly introduced features. The chapters deal with implementation details, providing code samples, where necessary. Chapter Usage demonstration shows an example usage of aggregator application, developed to improve benchmarker result comparison. The last chapter, Conclusion briefly summarizes the results of this thesis, proposing further enhancements and development of the current state of implementation. 4 2 Constraint programming “Constraint programming is a powerful paradigm for solv- ing combinatorial search problems that draws on a wide range of techniques from artificial intelligence, computer science, databases, programming languages and operations research.” [18] Constraint programming has emerged as a branch of artificial intel- ligence, and currently has many applications in real world information systems (e.g. planning, scheduling). It is based on the concept of deci- sion variables and constraints, which restrict the values that individual variables can take. Its advantage lies in the ability to represent prob- lems in natural way, with variables directly corresponding to problem entities, leading to relatively simple formulation of the problem and better understanding of the solution [11]. This chapter provides a brief introduction into related problematics, starting with definition of the Constraint satisfaction problem [24]. Definition 1 (Constraint satisfaction problem). Constraint satisfac- tion problem (CSP) is defined as a triple (X, D, C), where • X = {x1, ..., xn} is a finite set of variables, • D = {D1, ..., Dn} is a finite set of values (domain), • C = {c1, ..., cm}, ci ⊆ Di1 ×...×Dik is a finite set of constraints. Solution of a CSP is an assignment of a single value from the domain to each variable such that no constraint is violated [17]. Definition 2 (Solution). (d1, ..., dn) ∈ D1 × ... × Dn is a solution of (X, D, C), if: •∀ ci ∈ C defined on xi1 , ..., xik :(di1 , ..., dik ) ∈ ci. Constraint satisfaction problems may have multiple number of so- lutions — in that case the problem is referred to as satisfiable. On the other hand, a problem with no possible solutions is called unsatisfiable. In cases, where the problem is over-constrained (it is not possible to 5 2. Constraint programming satisfy all constraints at the same time), or some solutions are qualita- tively better than the other, it is often advantageous to introduce a level of distinction between individual solutions. This motivates definition of Constraint Statisfaction Optimization Problems [23]. Definition 3 (Constraint Satisfaction Optimization Problem). Con- straint Satisfaction Optimization Problem is defined as a quadriple (X, D, Ch,Cs), where • X = {x1, ..., xn} is a finite set of variables, • D = {D1, ..., Dn} is a finite set of values (domain), • Ch = {C1, ..., Cn}, Ci ⊆ Di1 × ... × Dik is a finite set of hard constraints, where Ci is defined on Y ⊆ X, Yi = {xi1 , ..., xik }, + • Cs = {F1, ..., Fj}, Fj : Dj1 ×...×Djl → R is a finite set of soft constraints, where Fj defined on Qj ⊆ X, Qj = {xj1 , ..., xjl }. Additionally, an objective function is defined as follows: l ~ P ~ ~ ~ • F (d) = Fj(d[Qj), where
Details
-
File Typepdf
-
Upload Time-
-
Content LanguagesEnglish
-
Upload UserAnonymous/Not logged-in
-
File Pages60 Page
-
File Size-