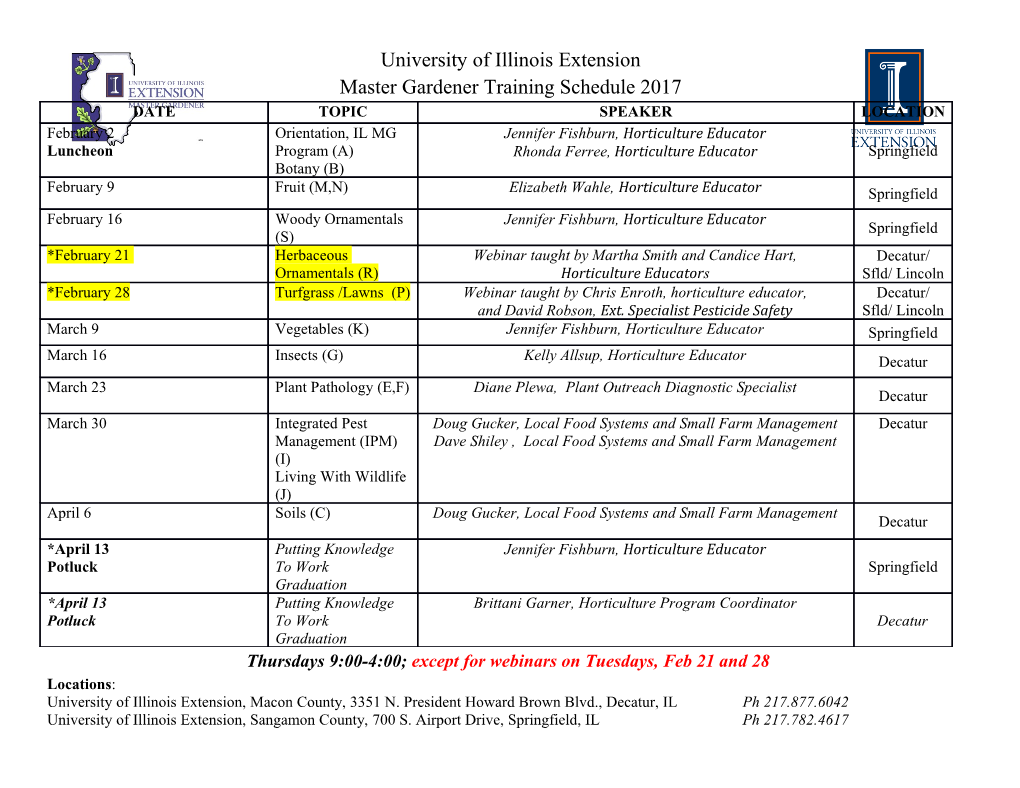
Research and Evaluation Making sense of Mendelian randomisation and its use in health research A short overview Sean Harrison, Laura Howe and Alisha R. Davies Suggested citation Harrison S1, Howe L1, Davies AR2 (2020). Making sense of Mendelian randomisation and its use in public health research. Cardiff: Public Health Wales NHS Trust & Bristol University 1 MRC Integrative Epidemiology Unit, University of Bristol 2 Public Health Wales NHS Trust Funding statement This work is part of a project entitled ‘Social and Economic consequences of health: causal inference methods and longitudinal, intergenerational data’, which is part of the Health Foundation’s Social and Economic Value of Health Programme (grant ID: 807293). © 2020 Public Health Wales NHS Trust Copyright in the typographical arrangement, design and layout belongs to Public Health Wales NHS Trust. Acknowledgements We would like to thank the Health Foundation Social and Economic Value of Health Steering Group for their comments on earlier drafts. ISBN 978-1-78986-154-79 Research and Evaluation Division Tel: +44 (0)29 2022 7744 Knowledge Directorate Email: [email protected] Public Health Wales NHS Trust Number 2 Capital Quarter @PHREWales Tyndall Street @PublicHealthWales Cardiff CF10 4BZ www.bristol.ac.uk/integrative-epidemiology/mr-methods Contents 1. Introduction 4 2. Why do we need to understand cause and effect? 5 2.1 What is confounding? 6 2.2 What is reverse causality? 6 2.3 Approaches to overcome confounding and reverse causality, and the limitations 6 3. What is Mendelian randomisation and how might it help? 7 3.1 What is a genetic variant? 7 3.2 When can Mendelian randomisation be applied? 9 3.3 What are the strengths of Mendelian randomisation compared to traditional study designs? 9 3.4 What are the limitations of Mendelian randomisation? 10 3.5 Key considerations when interpreting the results 12 4. Where has Mendelian randomisation influenced the discussion or provided answers? 16 4.1 Alcohol and Cardiovascular Disease 16 4.2 C-reactive Protein and Coronary Heart Disease 18 4.3 Body-mass index and Hospital Costs 18 5. Conclusions 19 6. A dictionary of terms for Mendelian randomisation: what does it all mean? 20 7. References 22 Making sense of Mendelian randomisation and its use in health research 3 1. Introduction In this overview, we explain the principles of Mendelian randomisation, and Population health research seeks to illustrate how it is better understand the causes and being used to consequences of ill health. It generates challenge and inform evidence to help inform decision making population health. and action to protect good health, and ensures the capacity to benefit is equitable across all individuals irrespective of circumstances. The most common studies which explore the relationship between causes (i.e. exposures) This short report is intended for a and outcomes are observational in design. non-specialist audience (including but not They examine the characteristics of individuals, limited to health economists, academics their experiences and surroundings using in other areas of expertise, public health routinely available information (e.g. health practitioners or policy makers) to increase and non-health data) or data collected for awareness of the approach, its strengths and the specific question (e.g. in surveys). But this weaknesses and its application. approach faces two key challenges (reverse causality and confounding – both described in This is not a full review of Mendelian more detail below) which may limit our ability randomisation, nor is it a technical reference to identify whether there is a true association document, but we have provided references between exposure and outcome. Mendelian for further reading for those who are randomisation is a different statistical interested in learning more. approach harnessing the information contained within our genome to help overcome these challenges. 4 2. Why do we need to understand cause and effect? Understanding the relationships Figure 1. Confounding and reverse between cause (i.e. exposure) and causality in the association between effect (i.e. outcome) is essential to obesity and education outcomes informing policy and practice. Figure 1a Reliable evidence is needed to help Educational understand what we need to focus Obesity Outcomes efforts on, if intervening could lead to benefits, and the potential magnitude of the benefits of intervention. Question: does obesity cause poor educational outcomes, does poor education cause obesity, For example, an observational study may find or does something else cause both? that obesity is associated with poor educational outcomes. However, this conclusion may Figure 1b not be correct if there are other factors associated with both exposure and outcome. For example, people living in less affluent Deprivation areas have lower educational attainment, and are also at higher risk of obesity (Figure 1a). The higher risk of obesity amongst those Educational Obesity with lower education attainment is likely to Outcomes be due, in part, to deprivation (the challenge termed ‘confounding’) (Figure 1b). A further complication is that association may be in the Confounding: deprivation causes both obesity and poor educational outcomes, making it seem other direction i.e. poor educational outcome like obesity causes poor educational outcomes. is the cause of obesity, due to low self-esteem and increased uptake of health harming behaviours leading to obesity (the challenge Figure 1c termed ‘reverse causality’), (Figure 1c). Educational Obesity Outcomes Reverse causality: poor education causes obesity, rather than obesity causing poor educational outcomes. Making sense of Mendelian randomisation and its use in health research 5 2.1. What is confounding? 2.3. Approaches to overcome Confounding variables are factors that we confounding and reverse causality, know about, we can measure and control for and the limitations in statistical analysis. However, even when The gold standard method to address both we know about a confounder, we are unlikely confounding and causality is a randomised to have measured it perfectly, especially controlled trial (RCT). RCTs usually work by for complex things such as socioeconomic randomly splitting a sample of participants circumstances. There will also be confounders into two or more groups, giving one group we do not know about, have not measured and the exposure (usually an intervention), and have not considered. This means there is still the other group(s) (control group) a different some confounding (residual confounding) in exposure (or intervention). An intervention most observational studies. can be a drug, a diet, information; anything that might change the individual’s outcome in some 2.2. What is reverse causality? way. Because which group a participant ends up Reverse causality is assigning a direction to an in is random, the only difference between the association between two variables incorrectly, two groups should be whether they received for example by stating that lung cancer causes the intervention or not, which means there cigarette smoking, rather than the reverse. should be no residual confounding. In addition, Causality is very difficult to determine for many there can be no reverse causality, as the associations, even when confounding is not intervention clearly comes before the outcome an issue. In the example above (Figure 1), did of interest. obesity lead to poor educational outcomes, However, for many research questions, it is or did poor educational outcomes lead to impossible or unethical to randomly assign the obesity? Statistical tests can, in general, only exposure. For example, it would not be possible tell you whether two variables are associated nor acceptable to randomly allocate obesity. – to determine causality, you need to consider study design, biological plausibility, and other relevant information. 6 3. What is Mendelian randomisation and how might it help? Mendelian randomisation is a 3.1. What is a genetic variant? statistical approach that uses genetics Genetic variants are small parts of the genome to provide information about the (Box 1) which can be closely related to human relationship between an exposure characteristics (e.g. height, weight, blood and outcome (a type of instrumental pressure) and health conditions (e.g. diabetes, variable analysis) (1). coronary heart disease, asthma). There can be multiple genetic variants for a single The combination of genetic variants a person characteristic or health condition; for example, receives from their parents is randomly assigned over 900 variants are known to affect our at conception - making Mendelian randomisation body mass index (BMI) (3). Collectively these a natural experiment which is potentially less genetic variants explain only a small amount of likely than observational studies to be subject to the variation in BMI across a population (6% in confounding and reverse causality, and relatively total), they are not deterministic (i.e. all people quicker and easier than RCT designs to complete with genetic variants that predispose to high a large scale research study. BMI do not necessarily have a high BMI), and their existence does not negate the important Mendelian randomisation as an approach has environmental influences on BMI. Nonetheless become widespread in the past decade. The they can be used to help understand the expansion of large scale genome wide association consequences of BMI, since people who
Details
-
File Typepdf
-
Upload Time-
-
Content LanguagesEnglish
-
Upload UserAnonymous/Not logged-in
-
File Pages23 Page
-
File Size-