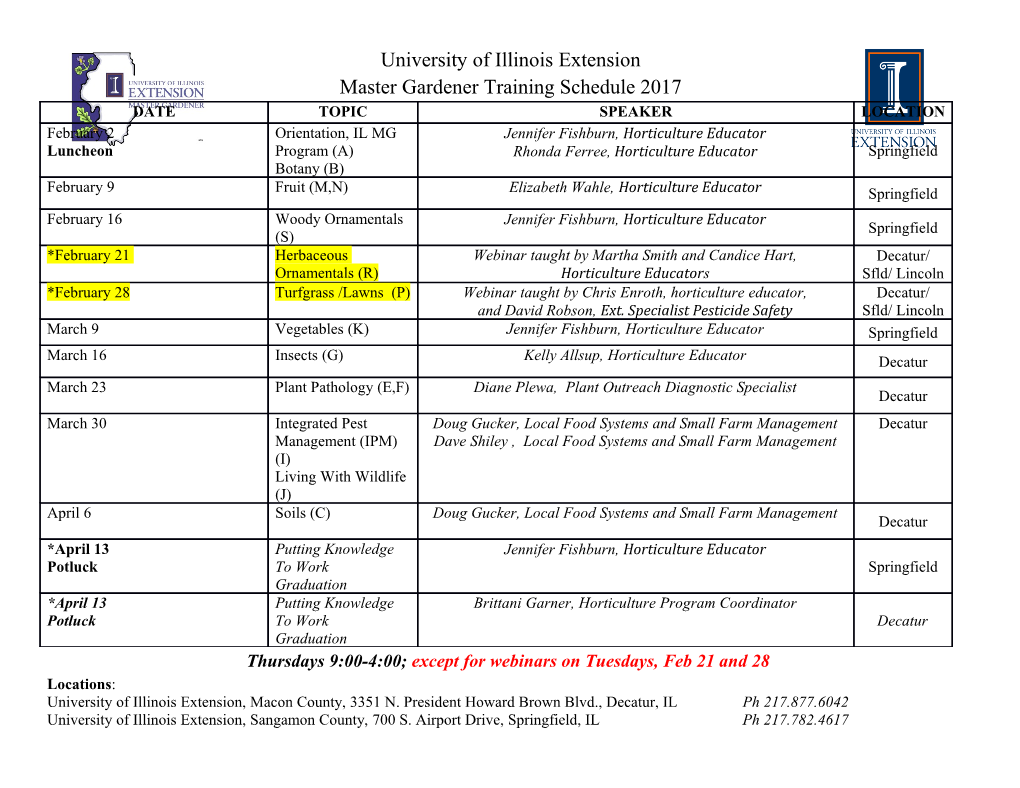
Math 541: Statistical Theory II Fisher Information and Cram¶er-RaoBound Instructor: Songfeng Zheng In the parameter estimation problems, we obtain information about the parameter from a sample of data coming from the underlying probability distribution. A natural question is: how much information can a sample of data provide about the unknown parameter? This section introduces such a measure for information, and we can also see that this information measure can be used to ¯nd bounds on the variance of estimators, and it can be used to approximate the sampling distribution of an estimator obtained from a large sample, and further be used to obtain an approximate con¯dence interval in case of large sample. In this section, we consider a random variable X for which the pdf or pmf is f(xjθ), where θ is an unknown parameter and θ 2 £, with £ is the parameter space. 1 Fisher Information Motivation: Intuitively, if an event has small probability, then the occurrence of this event brings us much information. For a random variable X » f(xjθ), if θ were the true value of the parameter, the likelihood function should take a big value, or equivalently, the derivative log-likelihood function should be close to zero, and this is the basic principle of maximum likelihood estimation. We de¯ne l(xjθ) = log f(xjθ) as the log-likelihood function, and @ f 0(xjθ) l0(xjθ) = log f(xjθ) = @θ f(xjθ) where f 0(xjθ) is the derivative of f(xjθ) with respect to θ. Similarly, we denote the second order derivative of f(xjθ) with respect to θ as f 00(xjθ). According to the above analysis, if l0(Xjθ) is close to zero, then it is expected, thus the random variable does not provide much information about θ; on the other hand, if jl0(Xjθ)j or [l0(Xjθ)]2 is large, the random variable provides much information about θ. Thus, we can use [l0(Xjθ)]2 to measure the amount of information provided by X. However, since X is a random variable, we should consider the average case. Thus, we introduce the following de¯nition: Fisher information (for θ) contained in the random variable X is de¯ned as: Z © 0 2ª 0 2 I(θ) = Eθ [l (Xjθ))] = [l (xjθ))] f(xjθ)dx: (1) 1 2 We assume that we can exchange the order of di®erentiation and integration, then Z Z @ f 0(xjθ)dx = f(xjθ)dx = 0 @θ Similarly, Z Z @2 f 00(xjθ)dx = f(xjθ)dx = 0 @θ2 It is easy to see that Z Z Z f 0(xjθ) E [l0(Xjθ)] = l0(xjθ)f(xjθ)dx = f(xjθ)dx = f 0(xjθ)dx = 0 θ f(xjθ) Therefore, the de¯nition of Fisher information (1) can be rewritten as 0 I(θ) = Varθ [l (Xjθ))] (2) Also, notice that · ¸ @ f 0(xjθ) f 00(xjθ)f(xjθ) ¡ [f 0(xjθ)]2 f 00(xjθ) l00(xjθ) = = = ¡ [l0(xjθ)]2 @θ f(xjθ) [f(xjθ)]2 f(xjθ) Therefore, Z · ¸ Z f 00(xjθ) © ª E [l00(xjθ)] = ¡ [l0(xjθ)]2 f(xjθ)dx = f 00(xjθ)dx ¡ E [l0(Xjθ)]2 = ¡I(θ) θ f(xjθ) θ Finally, we have another formula to calculate Fisher information: Z · ¸ @2 I(θ) = ¡E [l00(xjθ)] = ¡ log f(xjθ) f(xjθ)dx (3) θ @θ2 To summarize, we have three methods to calculate Fisher information: equations (1), (2), and (3). In many problems, using (3) is the most convenient choice. Example 1: Suppose random variable X has a Bernoulli distribution for which the pa- rameter θ is unknown (0 < θ < 1). We shall determine the Fisher information I(θ) in X. The point mass function of X is f(xjθ) = θx(1 ¡ θ)1¡x for x = 1 or x = 0: Therefore l(xjθ) = log f(xjθ) = x log θ + (1 ¡ x) log(1 ¡ θ) 3 and x 1 ¡ x x 1 ¡ x l0(xjθ) = ¡ and l00(xjθ) = ¡ ¡ θ 1 ¡ θ θ2 (1 ¡ θ)2 Since E(X) = θ, the Fisher information is E(X) 1 ¡ E(X) 1 1 1 I(xjθ) = ¡E[l00(xjθ)] = + = + = θ2 (1 ¡ θ)2 θ 1 ¡ θ θ(1 ¡ θ) Example 2: Suppose that X » N(¹; σ2), and ¹ is unknown, but the value of σ2 is given. ¯nd the Fisher information I(¹) in X. For ¡1 < x < 1, we have 1 (x ¡ ¹)2 l(xj¹) = log f(xj¹) = ¡ log(2¼σ2) ¡ 2 2σ2 Hence, x ¡ ¹ 1 l0(xj¹) = and l00(xj¹) = ¡ σ2 σ2 It follows that the Fisher information is 1 I(¹) = ¡E[l00(xj¹)] = σ2 If we make a transformation of the parameter, we will have di®erent expressions of Fisher information with di®erent parameterization. More speci¯cally, let X be a random variable for which the pdf or pmf is f(xjθ), where the value of the parameter θ is unknown but must lie in a space £. Let I0(θ) denote the Fisher information in X. Suppose now the parameter θ is replaced by a new parameter ¹, where θ = Á(¹), and Á is a di®erentiable function. Let I1(¹) denote the Fisher information in X when the parameter is regarded as ¹. We will have 0 2 I1(¹) = [Á (¹)] I0[Á(¹)]. Proof: Let g(xj¹) be the p.d.f. or p.m.f. of X when ¹ is regarded as the parameter. Then g(xj¹) = f[xjÁ(¹)]. Therefore, log g(xj¹) = log f[xjÁ(¹)] = l[xjÁ(¹)]; and @ log g(xj¹) = l0[xjÁ(¹)]Á0(¹): @¹ It follows that (· ¸ ) @ 2 ³ ´ I (¹) = E log g(Xj¹) = [Á0(¹)]2E fl0[XjÁ(¹)]g2 = [Á0(¹)]2I [Á(¹)] 1 @¹ 0 This will be veri¯ed in exercise problems. 4 Suppose that we have a random sample X1; ¢ ¢ ¢ ;Xn coming from a distribution for which the pdf or pmf is f(xjθ), where the value of the parameter θ is unknown. Let us now calculate the amount of information the random sample X1; ¢ ¢ ¢ ;Xn provides for θ. Let us denote the joint pdf of X1; ¢ ¢ ¢ ;Xn as Yn fn(xjθ) = f(xijθ) i=1 then Xn Xn ln(xjθ) = log fn(xjθ) = log f(xijθ) = l(xijθ): i=1 i=1 and 0 0 fn(xjθ) ln(xjθ) = (4) fn(xjθ) We de¯ne the Fisher information In(θ) in the random sample X1; ¢ ¢ ¢ ;Xn as Z Z © 0 2ª 0 2 In(θ) = Eθ [ln(Xjθ)] = ¢ ¢ ¢ [ln(Xjθ)] fn(xjθ)dx1 ¢ ¢ ¢ dxn which is an n-dimensional integral. We further assume that we can exchange the order of di®erentiation and integration, then we have Z Z @ f 0 (xjθ)dx = f (xjθ)dx = 0 n @θ n and, Z Z @2 f 00(xjθ)dx = f (xjθ)dx = 0 n @θ2 n It is easy to see that Z Z 0 Z 0 0 fn(xjθ) 0 Eθ[ln(Xjθ)] = ln(xjθ)fn(xjθ)dx = fn(xjθ)dx = fn(xjθ)dx = 0 (5) fn(xjθ) Therefore, the de¯nition of Fisher information for the sample X1; ¢ ¢ ¢ ;Xn can be rewritten as 0 In(θ) = Varθ [ln(Xjθ))] : It is similar to prove that the Fisher information can also be calculated as 00 In(θ) = ¡Eθ [ln(Xjθ))] : From the de¯nition of ln(xjθ), it follows that Xn 00 00 ln(xjθ) = l (xijθ): i=1 5 Therefore, the Fisher information " # Xn Xn 00 00 00 In(θ) = ¡Eθ [ln(Xjθ))] = ¡Eθ l (Xijθ) = ¡ Eθ [l (Xijθ)] = nI(θ): i=1 i=1 In other words, the Fisher information in a random sample of size n is simply n times the Fisher information in a single observation. Example 3: Suppose X1; ¢ ¢ ¢ ;Xn form a random sample from a Bernoulli distribution for which the parameter θ is unknown (0 < θ < 1). Then the Fisher information In(θ) in this sample is n I (θ) = nI(θ) = : n θ(1 ¡ θ) 2 Example 4: Let X1; ¢ ¢ ¢ ;Xn be a random sample from N(¹; σ ), and ¹ is unknown, but 2 the value of σ is given. Then the Fisher information In(θ) in this sample is n I (¹) = nI(¹) = : n σ2 2 Cram¶er-RaoLower Bound and Asymptotic Distri- bution of Maximum Likelihood Estimators Suppose that we have a random sample X1; ¢ ¢ ¢ ;Xn coming from a distribution for which the pdf or pmf is f(xjθ), where the value of the parameter θ is unknown. We will show how to used Fisher information to determine the lower bound for the variance of an estimator of the parameter θ. ^ ^ Let θ = r(X1; ¢ ¢ ¢ ;Xn) = r(X) be an arbitrary estimator of θ. Assume Eθ(θ) = m(θ), and ^ 0 the variance of θ is ¯nite. Let us consider the random variable ln(Xjθ) de¯ned in (4), it was 0 ^ 0 shown in (5) that Eθ[ln(Xjθ)] = 0. Therefore, the covariance between θ and ln(Xjθ) is n o ^ 0 ^ ^ 0 0 0 Covθ[θ; ln(Xjθ)] = Eθ [θ ¡ Eθ(θ)][ln(Xjθ) ¡ Eθ(ln(Xjθ))] = Eθ f[r(X) ¡ m(θ)]ln(Xjθ)g = E [r(X)l0 (Xjθ)] ¡ m(θ)E [l0 (Xjθ)] = E [r(X)l0 (Xjθ)] Z θ Z n θ n θ n 0 = ¢ ¢ ¢ r(x)ln(xjθ)fn(xjθ)dx1 ¢ ¢ ¢ dxn Z Z 0 = ¢ ¢ ¢ r(x)fn(xjθ)dx1 ¢ ¢ ¢ dxn (Use Equation 4) Z Z @ = ¢ ¢ ¢ r(x)f (xjθ)dx ¢ ¢ ¢ dx @θ n 1 n @ = E [θ^] = m0(θ) (6) @θ θ 6 By Cauchy-Schwartz inequality and the de¯nition of In(θ), n o2 ^ 0 ^ 0 ^ Covθ[θ; ln(Xjθ)] · Varθ[θ]Varθ[ln(Xjθ)] = Varθ[θ]In(θ) i.e., 0 2 ^ ^ [m (θ)] · Varθ[θ]In(θ) = nI(θ)Varθ[θ] Finally, we get the lower bound of variance of an arbitrary estimator θ^ as [m0(θ)]2 Var [θ^] ¸ (7) θ nI(θ) The inequality (7) is called the information inequality, and also known as the Cram¶er-Rao inequality in honor of the Sweden statistician H.
Details
-
File Typepdf
-
Upload Time-
-
Content LanguagesEnglish
-
Upload UserAnonymous/Not logged-in
-
File Pages13 Page
-
File Size-