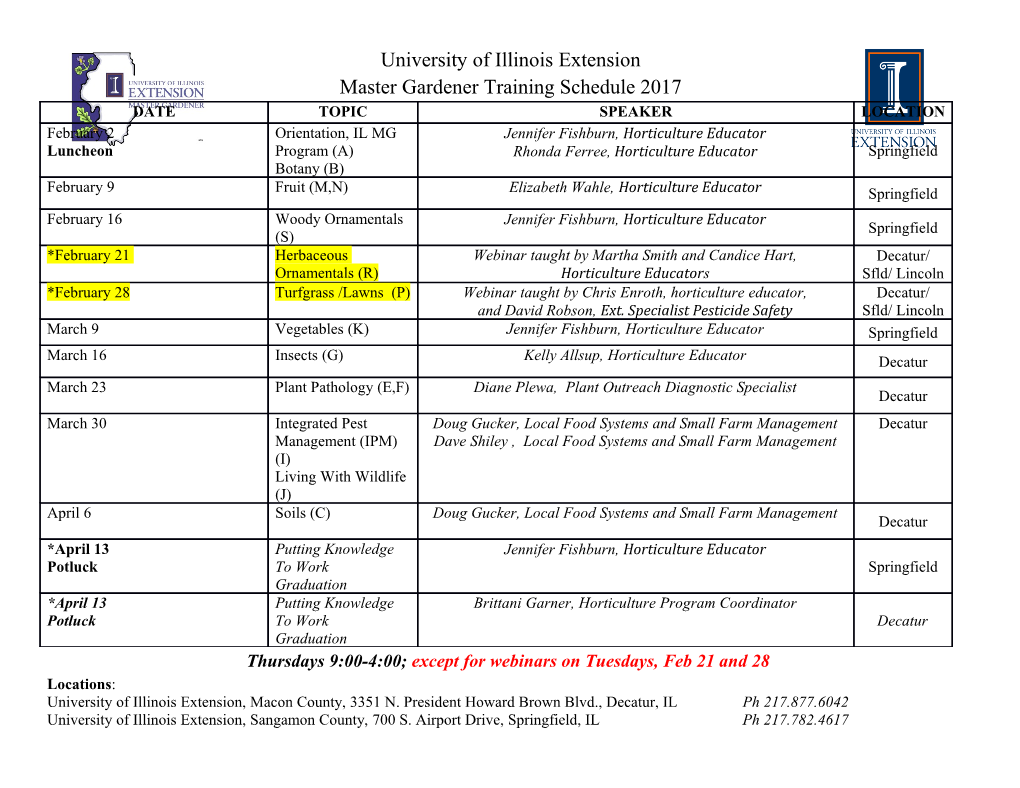
Exploring regulation in tissues with eQTL networks PNAS PLUS Maud Fagnya,b, Joseph N. Paulsona,b, Marieke L. Kuijjera,b, Abhijeet R. Sonawanec, Cho-Yi Chena,b, Camila M. Lopes-Ramosa,b, Kimberly Glassc, John Quackenbusha,b,d,1, and John Platiga,b,1 aDepartment of Biostatistics and Computational Biology, Dana-Farber Cancer Institute, Boston, MA 02115; bDepartment of Biostatistics, Harvard T. H. Chan School of Public Health, Boston, MA 02115; cChanning Division of Network Medicine, Brigham and Women’s Hospital and Harvard Medical School Boston, MA 02115; and dDepartment of Cancer Biology, Dana-Farber Cancer Institute, Boston, MA 02115 Edited by Jasper Rine, University of California, Berkeley, CA, and approved August 4, 2017 (received for review May 3, 2017) Characterizing the collective regulatory impact of genetic vari- biological pathways. In particular, we find three aspects of the ants on complex phenotypes is a major challenge in developing a eQTL network topology that inform tissue-level regulatory biol- genotype to phenotype map. Using expression quantitative trait ogy: (i) Communities—which are composed of SNPs and genes locus (eQTL) analyses, we constructed bipartite networks in which with a high density of within-group edges—are enriched for edges represent significant associations between genetic variants pathways, functionally related genes, and SNPs in tissue-specific and gene expression levels and found that the network structure active chromatin regions (actively transcribed and open regula- informs regulatory function. We show, in 13 tissues, that these tory regions); (ii) community hubs (core SNPs)—which are SNPs eQTL networks are organized into dense, highly modular commu- highly connected to genes in their community—are enriched for nities grouping genes often involved in coherent biological pro- active chromatin regions close to the transcriptional start site cesses. We find communities representing shared processes across and for GWAS association; and (iii) global hubs—which are con- tissues, as well as communities associated with tissue-specific pro- nected to many genes throughout the network—are enriched cesses that coalesce around variants in tissue-specific active chro- for distal elements such as nongenic enhancers and devoid of matin regions. Node centrality is also highly informative, with the GWAS association. The picture that emerges from analysis of global and community hubs differing in regulatory potential and the eQTL networks is a complex web of associations that reflects likelihood of being disease associated. the polygenic architecture across tissues and that provides a nat- ural framework for understanding both the shared and tissue- GTEx j expression quantitative trait locus j eQTL j bipartite networks j specific effects of genetic variants. These networks, along with GWAS SNP and gene network properties across all 13 tissues, are avail- able at networkmedicine.org:3838/eqtl/. ore than a decade after the sequencing of the human Results and Discussion Mgenome, our understanding of the relationship between genetic variation and complex traits remains limited. Genome- eQTL Networks Are Highly Modular. We downloaded RNA-Seq wide association studies (GWASs), which look for association data and imputed genotypes obtained from postmortem samples between common genetic variants and phenotypic traits, have coming from 50 tissue types and two cell lines from the GTEx resoundingly shown that complex phenotypes are influenced by version 6.0 dataset (phs000424.v6.p1, 2015-10-05 release) from many variants of relatively small effect size (1, 2), the overwhelm- the database of Genotypes and Phenotypes (dbGaP) (approved ing majority of which (∼93%) lie in noncoding regions of the protocol no. 9112). After quality control and preprocessing (see genome (3, 4). Those single-nucleotide polymorphisms (SNPs) SI Appendix, Fig. S1 and Materials and Methods for details of associated with complex traits are enriched for variants likely to RNA-Seq and genotyping data preprocessing and tissue-specific affect gene expression, as measured by expression quantitative trait locus (eQTL) analysis (5), suggesting that they influence Significance phenotypes through changes in gene regulation (6, 7). Identify- ing the regulatory role of these variants likely also depends on A core tenet in genetics is that genotype influences pheno- the tissues relevant to the phenotype. For example, eQTL iden- type. In an individual, the same genome can be expressed in tified in skeletal muscle and adipose tissues for type 2 diabetes substantially different ways, depending on the tissue. Expres- (T2D) have been shown to explain a greater proportion of the sion quantitative trait locus (eQTL) analysis, which associates disease heritability than those identified across tissues (8). Fur- genetic variants at millions of locations across the genome thermore, variants far from the transcriptional start site (TSS) of with the expression levels of each gene, can provide insight a gene, trans-eQTL, explain more of the heritability of T2D than into genetic regulation of phenotype. In each of 13 tissues those near the gene, cis-eQTL, and there is mounting evidence we performed an eQTL analysis, represented significant asso- for the importance of these variants in a variety of phenotypes ciations as edges in a network, and explored the structure (9–11). That trans-eQTL, which can influence hundreds of genes of those networks. We found clusters of eQTL linked to in humans (12), might have an impact on the phenotype is con- shared functions across tissues and tissue-specific clusters sistent with similar observations in model organisms (13). How- linked to tissue-specific functions, driven by genetic variants ever, large-scale detection of trans-eQTL across populations and with tissue-specific regulatory potential. Our findings provide tissues (14) has only recently become feasible in humans, and unique insight into the genotype–phenotype relationship. our understanding of how multiple cis- and trans-eQTL influ- ence gene expression and cellular functions in different tissues Author contributions: M.F., J.N.P., M.L.K., A.R.S., C.-Y.C., C.M.L.-R., K.G., J.Q., and J.P. is incomplete. designed research; M.F. and J.P. performed research; M.F., J.N.P., J.Q., and J.P. analyzed We performed a systems genetics analysis of the regulatory data; and M.F., J.N.P., M.L.K., A.R.S., C.-Y.C., C.M.L.-R., K.G., J.Q., and J.P. wrote the paper. effects of common [minor allele frequency (MAF) >5%] vari- The authors declare no conflict of interest. ants in 13 tissues collected by the Genotype–Tissue Expression This article is a PNAS Direct Submission. (GTEx) consortium. By constructing tissue-level eQTL networks Freely available online through the PNAS open access option. from cis- and trans-eQTL, where each significant SNP–gene asso- 1To whom correspondence may be addressed. Email: [email protected] or ciation within a tissue is cast as an edge, we find that these net- [email protected]. works provide insight into the shared and tissue-specific regu- This article contains supporting information online at www.pnas.org/lookup/suppl/doi:10. SYSTEMS BIOLOGY latory roles of common variants and their collective impact on 1073/pnas.1707375114/-/DCSupplemental. www.pnas.org/cgi/doi/10.1073/pnas.1707375114 PNAS j Published online August 29, 2017 j E7841–E7850 Downloaded by guest on September 25, 2021 gene filtering), we retained 29,242 genes (15, 16) and 5,096,867 regions into 15 chromatin states based on epigenetic marks mea- SNPs across all tissues. For statistical power purposes, we consid- sured in a specific tissue or cell line; these classifications were ered only tissues for which we had both RNA-Seq and imputed available for eight tissues (adipose subcutaneous, artery aorta, genotyping data for at least 200 individuals (SI Appendix, Fig. fibroblast cell line, esophagus mucosa, heart left ventricle, lung, S2). Twelve tissues and one cell line met all criteria (SI Appendix, skeletal muscle, and whole blood) (20). Correcting for local Fig. S1 and Table S1). linkage disequilibrium, we found that trans-eQTL are signifi- For each of the 13 tissues, we tested for association between cantly more likely to be located in regulatory and actively tran- SNP genotypes and gene expression levels both in cis and in trans, scribed regions (TSSs and their flanking regions, enhancers) in correcting for reported sex, age, ethnic background, and the top at least seven of the eight tissues and significantly less likely three principal components obtained using genotyping data (SI to fall into quiescent regions and constitutive heterochromatin Appendix, Figs. S1 and S3 and Materials and Methods). Including (Dataset S1). This is confirmed by P values combined across SNPs within 1 Mb of each gene, we found between 285,283 and tissues obtained using conditional logistic regression (stratified 691,333 significant cis-eQTL (5,301–11,035 genes) and between by tissue; Dataset S1). In addition, we found that a major- 7,151 and 15,183 significant trans-eQTL (326–955 genes) at a ity (50–66%) of trans-eQTL were also associated with genes false discovery rate (FDR) of 5% in each tissue. Despite dif- in cis. ferences in normalization, number and type of covariates, and For each of the 13 tissues, we represented the significant eQTL eQTL P-value calculation, on average 73% of our cis-eQTL were as a bipartite network, with nodes representing either SNPs or also detected by the GTEx project in each tissue (minimum 70% genes and edges representing significant SNP–gene associations in artery aorta and maximum 76% in thyroid; SI Appendix, Table (for example, heart left ventricle tissue; Fig. 1A). Each network S2). Consistent with previous reports (17–19), we find most cis- was composed of a “giant connected component” (GCC) plus eQTL are located around TSSs, with 50% of SNPs located within additional small connected components. To increase the size of ∼16,000 bp of the nearest TSS (14,767 bp for whole blood and the GCC and because network centrality measures are more 17,109 bp for thyroid).
Details
-
File Typepdf
-
Upload Time-
-
Content LanguagesEnglish
-
Upload UserAnonymous/Not logged-in
-
File Pages10 Page
-
File Size-