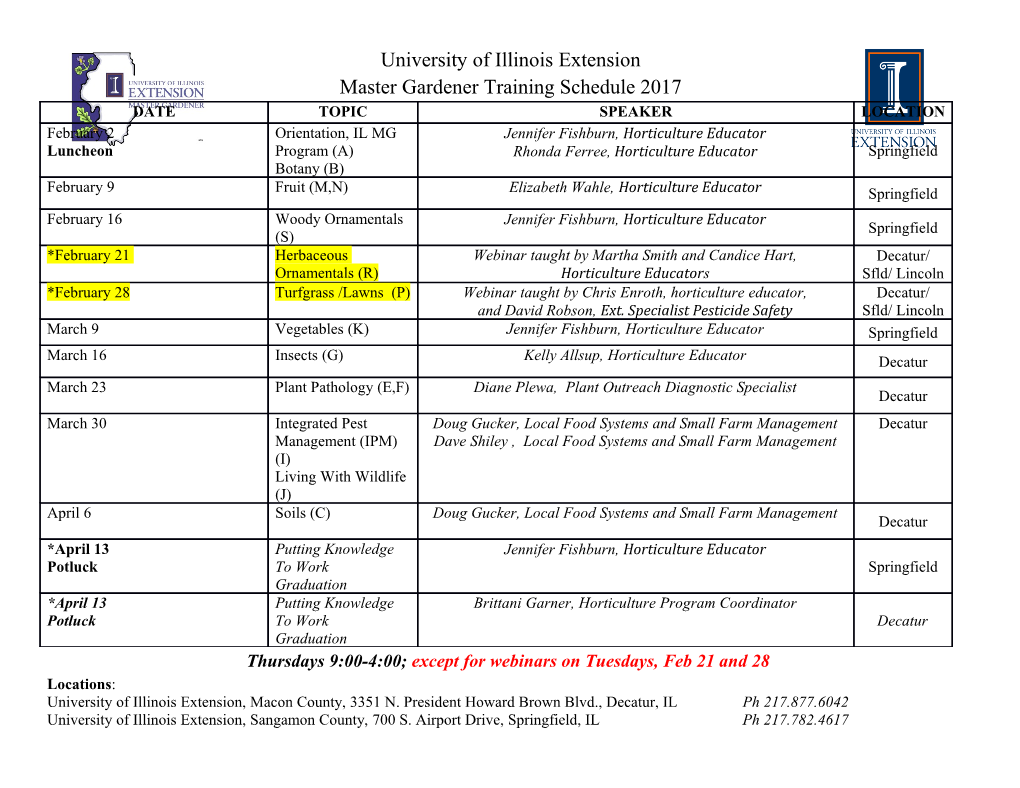
AN INFORMATION THEORETIC APPROACH TO THE EXPRESSIVENESS OF PROGRAMMING LANGUAGES by Joseph Ray Davidson Submitted in fulfilment of the requirements for the degree of Doctor of Philosophy University of Glasgow College of Science and Engineering School of Computing Science July 2015 The copyright in this thesis is owned by the author. Any quotation from the thesis or use of any of the information contained in it must acknowledge this thesis as the source of the quotation or information. Abstract The conciseness conjecture is a longstanding notion in computer science that programming languages with more built-in operators, that is more expressive languages with larger semantics, produce smaller programs on average. Chaitin defines the related concept of an elegant program such that there is no smaller program in some language which, when run, produces the same output. This thesis investigates the conciseness conjecture in an empirical manner. Influenced by the concept of elegant programs, we investigate several models of computation, and implement a set of functions in each programming model. The programming models are Turing Machines, λ-Calculus, SKI, RASP, RASP2, and RASP3. The information content of the programs and models are measured as characters. They are compared to investigate hypotheses relating to how the mean program size changes as the size of the semantics change, and how the relationship of mean program sizes between two models compares to that between the sizes of their semantics. We show that the amount of information present in models of the same paradigm, or model family, is a good indication of relative expressivity and aver- age program size. Models that contain more information in their semantics have smaller average programs for the set of tested functions. In contrast, the rela- tive expressiveness of models from differing paradigms, is not indicated by their relative information contents. RASP and Turing Machines have been implemented as Field Programmable Gate Array (FPGA) circuits to investigate hardware analogues of the hypotheses above. Namely that the amount of information in the semantics for a model directly influences the size of the corresponding FPGA circuit, and that the rela- tionship of mean circuit sizes between models is comparable to the relationship of mean program sizes. We show that the number of components in the circuits that realise the se- mantics and programs of the models correlates with the information required to implement the semantics and program of a model. However, the number of com- ponents to implement a program in a circuit for one model does not relate to the number of components implementing the same program in another model. This is in contrast to the more abstract implementations of the programs. Information is a computational resource and therefore follows the rules of Blum’s axioms. These axioms and the speedup theorem are used to obtain an alternate proof of the undecidability of elegance. This work is a step towards unifying the formal notion of expressiveness with the notion of algorithmic information theory and exposes a number of interesting research directions. A start has been made on integrating the results of the thesis with the formal framework for the expressiveness of programming languages. 2 Contents 1 Introduction 13 1.1 Motivation............................... 15 1.2 InvestigationOverview . 15 1.3 Hypotheses .............................. 16 1.4 Contributions ............................. 18 1.5 Structure ............................... 21 1.6 Publications.............................. 21 2 Literature Review 23 2.1 Computability............................. 23 2.1.1 HilbertandGödel. 23 2.1.2 Church and Turing . 26 2.2 Information and Algorithmic Theories . 29 2.2.1 Kolmogorov-Chaitin Complexity . 30 2.2.2 Elegance............................ 31 2.2.3 Other Measures of Complexity . 32 2.3 ModelsofComputation. 33 2.3.1 Imperative/Procedural Languages . 33 2.3.2 Functional Language . 41 2.4 Semantics ............................... 51 2.4.1 Structured Operational Semantics . 52 2.5 Expressiveness............................. 54 2.5.1 Formalisations. 55 2.5.2 Formalising Expressiveness . 56 2.5.3 The Conciseness Conjecture . 58 3 2.6 Conclusion............................... 58 3 Preliminaries 60 3.1 HypothesesRevisited. 60 3.1.1 BlumsAxioms......................... 60 3.1.2 The Semantic Information and Total Information Hypotheses 63 3.1.3 The Semantic Circuit and Total Circuit Hypotheses . 70 3.1.4 HypothesesSummary. 72 3.2 ComparisonMetrics. 73 3.3 Formats ................................ 74 3.3.1 Semantics ........................... 74 3.3.2 Turing Machines . 75 3.3.3 RASPmachines........................ 76 3.3.4 λ-calculus ........................... 76 3.3.5 SKIcombinators ....................... 78 3.4 Semantics ............................... 79 3.4.1 Turing Machines . 80 3.4.2 RASPMachines........................ 84 3.4.3 λ-calculus ........................... 92 3.4.4 SKI combinator calculus . 98 3.5 SemanticSizes............................. 100 4 Arithmetic, List and Universal Programs 103 4.1 PrimitiveandPartialRecursion . 103 4.2 TheArithmeticFunctions . 105 4.2.1 Addition............................ 106 4.2.2 Subtraction .......................... 108 4.2.3 Equality ............................ 110 4.2.4 Multiplication . 112 4.2.5 Division ............................ 114 4.2.6 Exponentiation . 117 4.3 FunctionsonaList .......................... 119 4.3.1 ListMembership . 119 4 4.3.2 LinearSearch ......................... 122 4.3.3 ReversingaList. 123 4.3.4 Statefully Reversing a List . 127 4.3.5 BubbleSort .......................... 130 4.4 UniversalMachines . 134 4.4.1 Universal Turing Machines . 135 4.4.2 Universal RASP Machines . 139 4.5 Results................................. 143 4.6 Conclusion............................... 144 5 Circuit Information 146 5.1 InfiniteRegress ............................ 146 5.2 Background .............................. 148 5.2.1 Architecture and Components . 149 5.3 Implementations ........................... 150 5.3.1 RASP ............................. 153 5.3.2 TM............................... 154 5.4 Results................................. 159 6 Analysis 163 6.1 OverallTrends ............................ 164 6.1.1 Arithmetic........................... 164 6.1.2 List .............................. 166 6.1.3 Universal ........................... 166 6.2 GroupedAnalysis........................... 167 6.2.1 RASPMachines........................ 169 6.2.2 RASPvsTM ......................... 171 6.2.3 SKI vs λ-calculus ....................... 172 6.2.4 RASPvsSKI ......................... 174 6.2.5 RASP vs λ-calculus...................... 175 6.2.6 TMvsSKI .......................... 176 6.2.7 TM vs λ-calculus ....................... 177 6.2.8 The SI and TI Hypotheses . 178 5 6.3 FPGAAnalysis ............................ 180 6.3.1 RASPsonFPGAs ...................... 187 6.3.2 RASPvsTM ......................... 189 6.3.3 The SC and TC Hypotheses . 190 6.4 FurtherObservations . 193 6.4.1 Phase Transitions . 193 6.4.2 Interpretation vs Evaluation Semantics . 195 6.5 Inputs ................................. 199 6.5.1 RASPs............................. 199 6.5.2 TM............................... 201 6.5.3 λ-Calculus........................... 202 6.5.4 SKI .............................. 203 6.5.5 Comparison .......................... 205 6.6 TheUTM ............................... 206 6.6.1 Neary’sUTM ......................... 206 6.6.2 Encodings ........................... 208 6.6.3 InputGrowth ......................... 213 6.7 Conclusions .............................. 214 7 Discussion and Conclusion 217 7.1 ThisWork............................... 217 7.1.1 Aims.............................. 217 7.1.2 Method ............................ 218 7.1.3 Results............................. 219 7.2 RelatedAspects............................ 223 7.2.1 Conservative Extensions . 223 7.2.2 Compilation . 228 7.2.3 Types ............................. 229 7.2.4 SemanticSchemes. 230 7.2.5 Related Minimalism . 232 7.3 FurtherInvestigations . 235 7.3.1 Formalism........................... 236 7.3.2 Program Equivalences . 238 6 7.3.3 InputSizes .......................... 239 7.3.4 Phase Transitions . 241 7.3.5 Symbol Grounding . 242 7.3.6 OtherWork .......................... 244 Bibliography 245 A The Busy Beaver Problem 254 A.1 TuringMachineBusyBeavers . 254 A.2 RASPBusyBeavers ......................... 255 A.3 Finding the Champions . 256 A.3.1 BruteForceMethods . 256 A.3.2 GeneticAlgorithms . 257 A.4 Reflection ............................... 259 A.4.1 LandscapeandFitness . 259 A.4.2 ArchitectureandSeeding. 260 B Full Programs 261 B.1 RASP ................................. 261 B.1.1 Addition............................ 261 B.1.2 Subtraction .......................... 262 B.1.3 Equality ............................ 262 B.1.4 Multiplication. 263 B.1.5 Division ............................ 264 B.1.6 Exponentiation . 265 B.1.7 ListMembership . 266 B.1.8 LinearSearch ......................... 267 B.1.9 ListReversal ......................... 268 B.1.10 StatefulListReversal. 269 B.1.11BubbleSort .......................... 270 B.1.12UniversalTM . 272 B.1.13UniversalRASP. 274 B.2 RASP2................................. 278 B.2.1 Addition............................ 279 7 B.2.2 Subtraction .......................... 279 B.2.3 Equality ............................ 279 B.2.4 Multiplication. 280 B.2.5 Division ...........................
Details
-
File Typepdf
-
Upload Time-
-
Content LanguagesEnglish
-
Upload UserAnonymous/Not logged-in
-
File Pages361 Page
-
File Size-