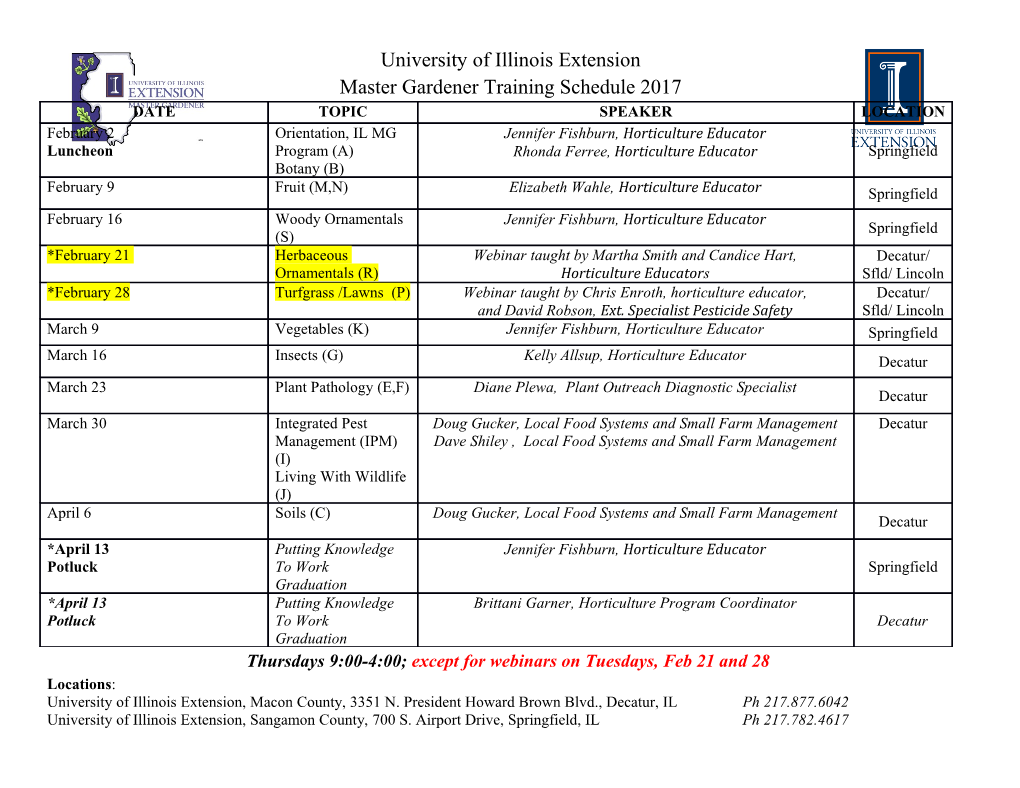
Rules of Engagement: Competitive Coevolutionary Dynamics in Computational Systems by John Peter Cartlidge Submitted in accordance with the requirements for the degree of Doctor of Philosophy. The University of Leeds School of Computing June 2004 The candidate confirms that the work submitted is his own and that the appropriate credit has been given where reference has been made to the work of others. This copy has been supplied on the understanding that it is copyright material and that no quotation from the thesis may be published without proper acknowledgement. Abstract Given that evolutionary biologists have considered coevolutionary interactions since the dawn of Darwinism, it is perhaps surprising that coevolution was largely overlooked during the formative years of evolutionary computing. It was not until the early 1990s that Hillis' seminal work thrust coevolution into the spotlight. Upon attempting to evolve fixed-length sorting networks, a problem with a long and competitive history, Hillis found that his standard evolutionary algorithm was producing sub-standard networks. In response, he decided to reciprocally evolve a population of test- lists against the sorting network population; thus producing a coevolutionary system. The result was impressive; coevolution not only outperformed evolution, but the best network it discovered was only one comparison longer than the best-known solution. For the first time, a coevolutionary algorithm had been successfully applied to problem-solving. Pre-Hillis, the shortcomings of standard evolutionary algorithms had been understood for some time: whilst defining an adequate fitness function can be as challenging as the problem one is hoping to solve, once achieved, the accumulation of fitness-improving mutations can push a population towards local optima that are difficult to escape. Co- evolution offers a solution. By allowing the fitness of each evolving individual to vary (through competition) with other reciprocally evolving individuals, coevolution removes the requirement of a fitness yardstick. In conjunction, the reciprocal adaptations of each individual begin to erode local optima as soon as they appear. However, coevolution is no panacea. As a problem-solving tool, coevolutionary al- gorithms suffer from some debilitating dynamics, each a result of the relative fitness as- sessment of individuals. In a single-, or multi-, population competitive system, coevo- lution may stabilize at a suboptimal equilibrium, or mediocre stable state; analogous to the traditional problem of local optima. Populations may become highly specialized in an unanticipated (and undesirable) manner; potentially resulting in brittle solutions that are fragile to perturbation. The system may cycle; producing dynamics similar to the children's game rock-paper-scissors. Disengagement may occur, whereby one population out-performs another to the extent that individuals cannot be discriminated on the basis of fitness alone; thus removing selection pressure and allowing populations to drift. Finally, coevolution's relative fitness assessment renders traditional visualization techniques (such as the graph of fitness over time) obsolete; thus exacerbating each of the above problems. This thesis attempts to better understand and address the problems of coevolution through the design and analysis of simple coevolutionary models. “Reduced virulence”— a novel technique specifically designed to tackle disengagement—is developed. Empirical results demonstrate the ability of reduced virulence to combat disengagement both in sim- i ple and complex domains, whilst outperforming the only known competitors. Combin- ing reduced virulence with diversity maintenance techniques is also shown to counteract mediocre stability and over-specialization. A critique of the CIAO plot—a visualization technique developed to detect coevo- lutionary cycling—highlights previously undocumented ambiguities; experimental evi- dence demonstrates the need for complementary visualizations. Extending the scope of visualization, a first exploration into coevolutionary steering is performed; a technique al- lowing the user to interact with a coevolutionary system during run-time. Using a simple model incorporating reduced virulence, the coevolutionary steering demonstration high- lights the future potential of such tools for both research and education. The role of neutrality in coevolution is discussed in detail. Whilst much emphasis is placed upon neutral networks in the evolutionary computation literature, the nature of coevolutionary neutrality is generally overlooked. Preliminary ideas for modelling coevolutionary neutrality are presented. Finally, whilst this thesis is primarily aimed at a computing audience, strong reference to evolutionary biology is made throughout. Exemplifying potential crossover, the CIAO plot, a tool previously unused in biology, is applied to a simulation of E. Coli, with results confirming empirical observations of real bacteria. ii Acknowledgements Thankfully, throughout my PhD I have received tremendous help and support from many colleagues, friends and family: to each and every one, I am eternally grateful. For love, support and patience throughout, I want to thank Helen Pickup and Mum. For technical help, I want to give particular thanks to Martin Thompson and Lee Bulmer: without Martin's graphical expertise and computer support, the steering interface would have failed to leave the drawing board; without Lee's coding skills, I'd still be sorting through reams of data by hand. I would like to thank Steven Walker for preliminary CIAO plot investigations (Walker, 2000) and Dave Gordon for drawing my attention to similari- ties between reduced virulence and the Laffer Curve. Special thanks goes to Dave Harris, Simon Lessels and Hywel Williams for guidance, support and general input throughout; nobody else has had to endure my ranting quite so much! Thanks to the Biosystems group at the University of Leeds, both past and present, for being a great group to work with; and the Biosystems reading group for many hours of stimulating conversation and drinking. Thanks to all those from outside Leeds whose conversations and opinions have helped to direct my research, including (especially) Richard Watson, Jordan Pollack, Dave Cliff, Peter Todd and Geoffrey Miller. A big thanks to Neil Currums for giving me time when- ever I needed it, Tom Bysouth for accommodation during my many last-minute visits and Tom Barnes-Lawrence for access to his computer. Finally, none deserves acknowledge- ment more than Seth Bullock, my supervisor and mentor, without whom this thesis would not have been possible: thanks, Seth. To the one who would have enjoyed it most. iii Declarations Some parts of the work presented in this thesis have been published in the following articles: Cartlidge, J. & Bullock, S. (2002), “Learning lessons from the common cold: How reducing parasite virulence improves coevolutionary optimization”. In Congress on Evolutionary Computation, pp. 1420–1425. IEEE Press, Piscataway NJ. Bullock, S., Cartlidge, J. & Thompson, M. (2002), “Prospects for comptational steering of evolutionary computation”, in Bilotta, E., et al. (Eds.) Workshop Proceedings, Artificial Life VIII, pp 131–137. MIT Press, Cambridge MA. Cartlidge, J. & Bullock, S. (2003), “Caring Versus Sharing: How to Maintain Engagement and Diversity in Coevolving Populations”, in Banzhaf, W., Christaller, T., Dittrich, P., Kim, J., & Ziegler, J. (Eds.) Seventh European Conference on Artificial Life, pp 299–308. Springer-Verlag, Berlin, Germany. Cartlidge, J. & Bullock, S. (2004a), “Combating coevolutionary disengagement by reducing parasite virulence”, Evolutionary Computation, 12(2), 193–222. Cartlidge, J. & Bullock, S. (2004b), “Unpicking tartan CIAO plots: Understanding irregular coevolutionary cycling”, Adaptive Behaviour, 12(2), 69–92. iv Contents 1 Introduction 1 1.1 Outline . 1 1.1.1 The Problem . 2 1.1.2 Motivation . 3 1.1.3 Overview of Results . 3 1.1.4 Methodology . 4 1.2 How to Read this Thesis . 5 1.3 A History of the Coevolutionary Perspective . 5 1.3.1 Interspecific Interaction . 6 1.3.2 The Pre-Darwinian Perspective . 7 1.3.3 Darwin's Entangled Bank . 8 1.3.4 Challenges . 10 1.3.5 Post-Synthesis Developments . 11 1.3.5.1 The Evolutionary Synthesis . 11 1.3.5.2 New Techniques: A New Perspective . 13 1.3.5.3 The Coining of Coevolution . 16 1.4 Summary . 18 2 Review of Evolutionary Computation 19 2.1 Artificial Evolution . 19 2.2 Genetic Algorithms (GAs) . 21 2.2.1 A Simple Generational GA . 22 2.2.2 Worked Example . 23 2.2.3 Modes of Variation . 24 2.2.3.1 Mutation . 24 2.2.3.2 Recombination . 25 2.2.4 Assessment and Selection . 26 2.3 Realism . 29 v 2.3.1 Fixed Population Size . 31 2.3.2 Genotype-Phenotype Mapping . 31 2.3.3 Static Environment . 32 2.4 Summary . 33 3 Coevolutionary Computation 34 3.1 Background . 34 3.2 Defining Coevolution . 36 3.2.1 The Standard Definition of Coevolution . 37 3.2.2 The Coevolutionary Continuum . 38 3.2.3 When is it not Coevolution? . 45 3.2.4 Fitness Propensity and Fitness Realization . 46 3.2.5 A New Definition of Coevolution . 49 3.2.6 Relativism versus Absolutism . 49 3.2.7 Moving Mountains: A Dynamic Landscape . 51 3.2.8 The Nature of Objective Fitness . 53 3.3 The Success Stories . 54 3.4 Critique . 56 3.4.1 Visualization . 56 3.4.2 Overspecialisation . 59 3.4.3 Cycling . 61 3.4.4 Mediocre Stability . 67 3.4.5 Disengagement . 68 3.4.5.1 Classifying Disengagement . 71 3.4.5.2 Coevolutionary Neutrality . 73 3.5 Classroom Analogies . 74 3.6 Summary . 76 4 Research Statement 78 4.1 Objective . 78 4.2 Justification . 79 4.3 Prospects . 80 5 Reduced Parasite Virulence 81 5.1 Rationale . 81 5.1.1 Here Today, Gone Tomorrow . 81 5.1.2 Don't Bite the Hand that Feeds You . 82 vi 5.2 Analogies . 83 5.2.1 Parasite Virulence . 83 5.2.1.1 Myxomatosis: An Illustrative Example . 85 5.2.1.2 A Case of Group Selectionism? . 86 5.2.2 The Laffer Curve . 87 5.3 Implementation . 89 5.3.1 Maintaining Relative Fitness Diversity . 90 5.3.2 Asymmetry . 91 5.4 Summary .
Details
-
File Typepdf
-
Upload Time-
-
Content LanguagesEnglish
-
Upload UserAnonymous/Not logged-in
-
File Pages210 Page
-
File Size-