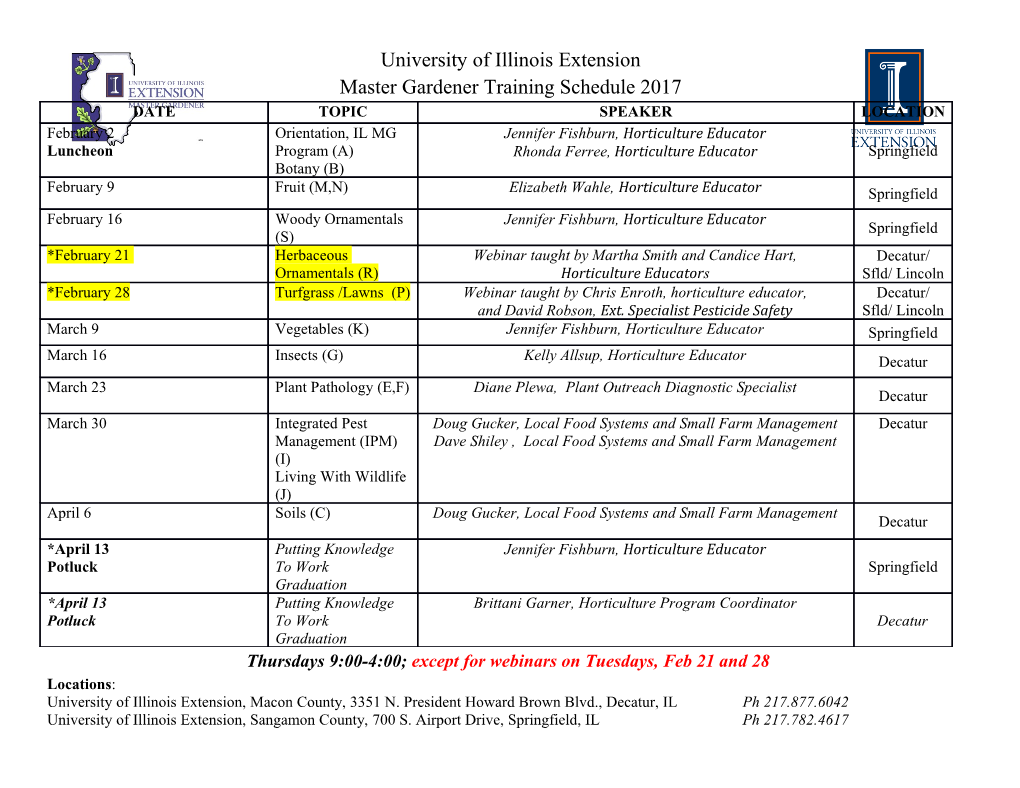
Florida State University Libraries Electronic Theses, Treatises and Dissertations The Graduate School 2017 Predicting Child Welfare Future Placements for Foster Youth: An Application of Statistical Learning to Child Welfare Andrew S. (Andrew Scott) Benesh Follow this and additional works at the DigiNole: FSU's Digital Repository. For more information, please contact [email protected] FLORIDA STATE UNIVERSITY COLLEGE OF HUMAN SCIENCES PREDICTING CHILD WELFARE FUTURE PLACEMENTS FOR FOSTER YOUTH: AN APPLICATION OF STATISTICAL LEARNING TO CHILD WELFARE By ANDREW S. BENESH A Dissertation submitted to the Department of Family and Child Sciences in partial fulfillment of the requirements for the degree of Doctor of Philosophy 2017 Copyright c 2017 Andrew S. Benesh. All Rights Reserved. Andrew S. Benesh defended this dissertation on March 23, 2017. The members of the supervisory committee were: Ming Cui Professor Directing Dissertation Carter Hay University Representative Lenore McWey Committee Member Francis Fincham Committee Member The Graduate School has verified and approved the above-named committee members, and certifies that the dissertation has been approved in accordance with university requirements. ii TABLE OF CONTENTS ListofTables.......................................... ... vi ListofFigures ......................................... viii Abstract............................................. ... x 1 INTRODUCTION 1 1.1 Background......................................... 1 1.1.1 The Importance of Placement Type . 1 1.1.2 The Importance of Placement Stability and Placement Changes . 2 1.1.3 Gaps in Understanding Placement Changes . 3 1.2 ResearchQuestions.................................. 4 1.2.1 Question 1. How Well do Child Factors, Caseworker Factors, and Foster Family Factors Predict Future Placements for Foster Youth? . 4 1.2.2 Question 2. How Well do Child Factors, Caseworker Factors, and Foster Family Factors Predict Different Types of Placement Changes? . 4 1.2.3 Methodological Innovations . 5 2 LITERATURE REVIEW 7 2.1 PlacementDisruption ................................ 7 2.1.1 EffectsofDisruption................................ 7 2.1.2 Causes of Disruption . 8 2.1.3 Limitations of Current Knowledge . 13 2.2 RandomForestModeling ................................ 15 2.2.1 Overview ...................................... 15 2.2.2 Classification Trees . 15 2.2.3 Random Forests . 16 3 METHOD 18 3.1 Sample............................................ 18 3.1.1 Sample Design . 18 3.1.2 Procedures ..................................... 19 3.2 Measures .......................................... 21 3.2.1 Outcomes...................................... 21 3.2.2 Child Factors . 21 3.2.3 Caseworker Factors . 24 3.2.4 Caregiver Factors . 25 3.3 Analytic Strategy . 26 3.3.1 General Approach . 26 3.3.2 Questions ...................................... 27 iii 4 RESULTS 29 4.1 PreliminaryAnalyses................................. 29 4.1.1 Youth Demographics . 29 4.1.2 Caregiver Demographics . 30 4.1.3 Caseworker Demographics . 30 4.2 Main Analyses . 30 4.2.1 QuestionOne.................................... 30 4.2.2 QuestionTwo.................................... 56 4.3 Post Hoc Analyses . 81 5 DISCUSSION 88 5.1 Question 1. How Well do Child Factors, Caseworker Factors, and Foster Family Factors Predict Future Placements for Foster Youth? . 88 5.1.1 Child Factors . 88 5.1.2 Caseworker Factors . 89 5.1.3 Caregiver Factors . 90 5.1.4 Combined Factors . 90 5.2 Question 2. How Well do Child Factors, Caseworker Factors, and Foster Family Factors Predict Different Types of Placement Changes? . 91 5.2.1 Child Factors . 91 5.2.2 Caseworker Factors . 92 5.2.3 Caregiver Factors . 92 5.2.4 Combined Factors . 92 5.3 ClinicalImplications ................................ 93 5.4 Limitations ......................................... 95 5.4.1 Utility........................................ 95 5.4.2 Racial Bias . 96 5.4.3 Data Quality . 97 5.4.4 Modeling Algorithms . 98 5.4.5 Outcome Constructs . 98 5.5 FutureResearch .................................... 99 5.5.1 Further Investigation of Identified Predictors . 99 5.5.2 New Variables . 99 5.5.3 Local Data . 99 5.5.4 Novel Targets . 100 5.6 Conclusion ......................................... 100 Appendices A Random Forest Demonstration 101 A.1 Data Overview and Partitioning . 101 A.2 LinearModeling ..................................... 103 A.3 ClassificationTree ................................... 104 A.4 RandomForestModel ................................... 109 iv B IRB Approval Letter 113 References......................................... 115 BiographicalSketch ..................................... 123 v LIST OF TABLES 1.1 Common Placement Types by Level of Care . 2 3.1 Hierarchy of Parent-Child Relationships . 20 3.2 Placement Types and Relative Level of Care . 21 4.1 Youth Demographics . 29 4.2 Caregiver Demographics . 31 4.3 Caseworker Demographics . 32 4.4 Predicted vs Observed Level of Care for the Model 1 Training Data Initial Fit . 33 4.5 Predicted vs Observed Level of Care for the Tuned Model 1 Testing Data . 39 4.6 Predicted vs Observed Level of Care for the Model 2 Training Data Initial Fit . 39 4.7 Predicted vs Observed Level of Care for the Tuned Model 2 Testing Data . 43 4.8 Predicted vs Observed Level of Care for the Model 3 Training Data Initial Fit . 48 4.9 Predicted vs Observed Level of Care for the Tuned Model 3 Testing Data . 52 4.10 Predicted vs Observed Level of Care for the Model 4 Training Data Initial Fit . 52 4.11 Predicted vs Observed Level of Care for the Tuned Model 4 Testing Data . 56 4.12 Predicted vs Observed Level of Care Change for the Model 5 Training Data Initial Fit 59 4.13 Predicted vs Observed Level of Care Change for the Tuned Model 5 Testing Data . 65 4.14 Predicted vs Observed Level of Care Direction Change for the Model 6 Training Data InitialFit.......................................... 65 4.15 Predicted vs Observed Level of Care Change for the Tuned Model 6 Testing Data . 71 4.16 Predicted vs Observed Level of Care Direction Change for the Model 7 Training Data InitialFit.......................................... 71 4.17 Predicted vs Observed Level of Care Change for the Tuned Model 7 Testing Data . 75 4.18 Predicted vs Observed Level of Care Direction Change for the Model 8 Training Data InitialFit.......................................... 75 4.19 Predicted vs Observed Level of Care Change for the Tuned Model 8 Testing Data . 81 vi 4.20 Predicted vs Observed Level of Care Change for the Tuned Model 9 Testing Data . 84 4.21 Summary of Fit for All Models . 87 vii LIST OF FIGURES 4.1 OOB Error by Number of Trees for Model 1 . 34 4.2 OOB Error by Number of Variables at Each Node for Model 1 . 35 4.3 OOBErrorbyNodeSizeforModel1 ......................... 36 4.4 Mean Accuracy Influence by Variable for Model 1 . 37 4.5 Mean Importance by Variable for Model 1 . 38 4.6 OOB Error by Number of Trees for Model 2 . 40 4.7 OOB Error by Number of Variables at Each Node for Model 2 . 41 4.8 OOBErrorbyNodeSizeforModel2 ......................... 42 4.9 Mean Accuracy Influence by Variable for Model 2 . 44 4.10 Mean Importance by Variable for Model 2 . 45 4.11 OOB Error by Number of Trees for Model 3 . 46 4.12 OOB Error by Number of Variables at Each Node for Model 3 . 47 4.13 OOB Error by Node Size for Model 3 . 49 4.14 Mean Accuracy Influence by Variable for Model 3 . 50 4.15 Mean Importance by Variable for Model 3 . 51 4.16 OOB Error by Number of Trees for Model 4 . 53 4.17 OOB Error by Number of Variables at Each Node for Model 4 . 54 4.18 OOB Error by Node Size for Model 4 . 55 4.19 Mean Accuracy Influence by Variable for Model 4 . 57 4.20 Mean Importance by Variable for Model 4 . 58 4.21 OOB Error by Number of Trees for Model 5 . 60 4.22 OOB Error by Number of Variables at Each Node for Model 5 . 61 4.23 OOB Error by Node Size for Model 5 . 62 4.24 Mean Accuracy Influence by Variable for Model 5 . 63 viii 4.25 Mean Importance by Variable for Model 5 . 64 4.26 OOB Error by Number of Trees for Model 6 . 66 4.27 OOB Error by Number of Variables at Each Node for Model 6 . 67 4.28 OOB Error by Node Size for Model 6 . 68 4.29 Mean Accuracy Influence by Variable for Model 6 . 69 4.30 Mean Importance by Variable for Model 6 . 70 4.31 OOB Error by Number of Trees for Model 7 . 72 4.32 OOB Error by Number of Variables at Each Node for Model 7 . 73 4.33 OOB Error by Node Size for Model 7 . 74 4.34 Mean Accuracy Influence by Variable for Model 7 . 76 4.35 Mean Importance by Variable for Model 7 . 77 4.36 OOB Error by Number of Trees for Model 8 . 78 4.37 OOB Error by Number of Variables at Each Node for Model 8 . 79 4.38 OOB Error by Node Size for Model 8 . 80 4.39 Mean Accuracy Influence by Variable for Model 8 . 82 4.40 Mean Importance by Variable for Model 8 . 83 4.41 Mean Accuracy Influence.
Details
-
File Typepdf
-
Upload Time-
-
Content LanguagesEnglish
-
Upload UserAnonymous/Not logged-in
-
File Pages134 Page
-
File Size-