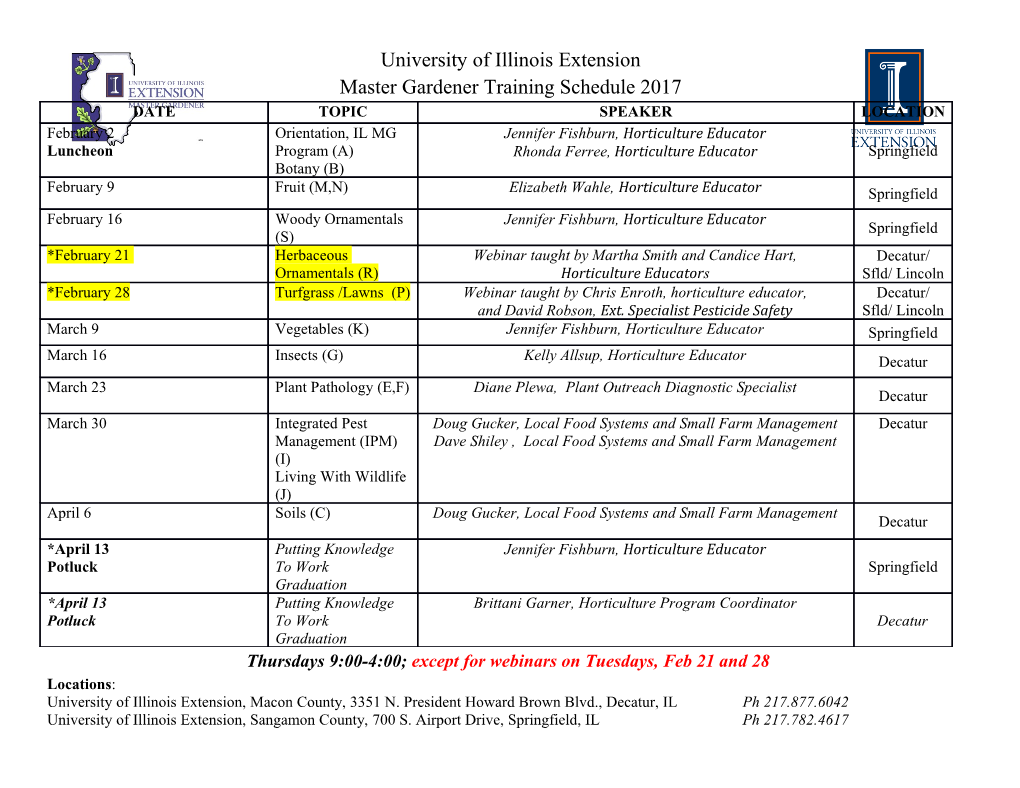
remote sensing Article An Improved Segmentation Method for Automatic Mapping of Cone Karst from Remote Sensing Data Based on DeepLab V3+ Model Han Fu 1,2, Bihong Fu 1,* and Pilong Shi 1 1 Key Laboratory of Digital Earth Science, Aerospace Information Research Institute, Chinese Academy of Sciences, Beijing 100094, China; [email protected] (H.F.); [email protected] (P.S.) 2 University of Chinese Academy of Sciences, Beijing 100049, China * Correspondence: [email protected]; Tel.: +86-10-8217-8096 Abstract: The South China Karst, a United Nations Educational, Scientific and Cultural Organization (UNESCO) natural heritage site, is one of the world’s most spectacular examples of humid tropical to subtropical karst landscapes. The Libo cone karst in the southern Guizhou Province is considered as the world reference site for these types of karst, forming a distinctive and beautiful landscape. Geomorphic information and spatial distribution of cone karst is essential for conservation and management for Libo heritage site. In this study, a deep learning (DL) method based on DeepLab V3+ network was proposed to document the cone karst landscape in Libo by multi-source data, including optical remote sensing images and digital elevation model (DEM) data. The training samples were generated by using Landsat remote sensing images and their combination with satellite derived DEM data. Each group of training dataset contains 898 samples. The input module of DeepLab V3+ network was improved to accept four-channel input data, i.e., combination of Landsat RGB images and DEM data. Our results suggest that the mean intersection over union (MIoU) using the four-channel data as training samples by a new DL-based pixel-level image segmentation approach Citation: Fu, H.; Fu, B.; Shi, P. is the highest, which can reach 95.5%. The proposed method can accomplish automatic extraction An Improved Segmentation Method of cone karst landscape by self-learning of deep neural network, and therefore it can also provide a for Automatic Mapping of Cone Karst from Remote Sensing Data Based on powerful and automatic tool for documenting other type of geological landscapes worldwide. DeepLab V3+ Model. Remote Sens. 2021, 13, 441. https://doi.org/ Keywords: UNESCO natural heritage site; cone karst landscape; segmentation; deep learning; 10.3390/rs13030441 multi-source remote sensing data Academic Editor: Devrim Akca Received: 29 December 2020 Accepted: 25 January 2021 1. Introduction Published: 27 January 2021 Karst landscapes are general term for the surface and underground landforms formed by the dissolution of water on soluble rocks. They are widely distributed in the world and Publisher’s Note: MDPI stays neutral have a total area of 1.25 million km2 in China [1]. Mapping of a karst landscape generally with regard to jurisdictional claims in aims to record its location, distribution, type and development stage in an area [2]. The for- published maps and institutional affil- mation of karst landscape is influenced by various factors, acting together or alone, such as iations. local lithology, geological structure, climate, precipitation and vegetation. Most of karst areas have complex topography and geomorphic conditions, fragile ecological environment and poor accessibility, which significantly restrict the development of regional land use, urban planning, mineral survey, geological disaster prevention and control, ecological Copyright: © 2021 by the authors. environment protection and tourism resources management [3]. Therefore, extracting the Licensee MDPI, Basel, Switzerland. geomorphic information and spatial distribution of karst landscapes effectively is essential This article is an open access article and significant for land use planning, ecological environment protection, and management distributed under the terms and for these heritage sites. conditions of the Creative Commons Currently, extraction of karst landscapes is traditionally conducted through field Attribution (CC BY) license (https:// investigations or interpretation using remote sensing images and digital elevation model creativecommons.org/licenses/by/ 4.0/). (DEM) data. Based on methods of visual interpretation [4,5], supervised classification [6], Remote Sens. 2021, 13, 441. https://doi.org/10.3390/rs13030441 https://www.mdpi.com/journal/remotesensing Remote Sens. 2021, 13, 441 2 of 16 ratio calculation [7], decision tree and maximum likelihood estimation (MLE) [8] applied to satellite remote sensing images, one can extract information of rocky desertification in karst areas. By using DEM data, researchers can extract various terrain factors, such as rivers [9,10], elevation [9,10], slope [4,11], aspect [4,11], for karst landform recognition. These traditional methods not only consume a lot of time, cost and human resources, but also their classification results of karst landscapes depend on experts’ knowledge and the accuracy is approximately 80% [12]. Both optical remote sensing images and DEM data were not jointly used for mapping karst landscapes [13,14]. Recently, deep learning (DL) has emerged as the state-of-the-art machine learning tech- nique with a great capability of remote sensing image classification. Instead of depending on manually-engineered shallow features, deep learning techniques automatically learn hi- erarchical features (from low-level to high-level) from input data directly [15,16]. However, due to the lack of training data, semantic segmentation based on deep learning is usually used to recognize features with regular shape or distinct boundaries, such as buildings [17], urban green space [18] and shorelines [19]. It is a big challenge for recognition of complex landscapes. The karst landscape, as one typical complex landscape, the geometry and texture information contained in remote sensing data and elevation information contained in DEM data are all important factors for segmentation [13]. DeepLab model is a convolutional network for image semantic segmentation proposed by Google in 2015 based on fully convolutional networks (FCNs) [20]. So far, it has four versions, i.e., DeepLab V1 [21], DeepLab V2 [22], DeepLab V3 [23] and DeepLab V3+ [24]. The three main advantages of the DeepLab system are efficiency, accuracy and simplicity, by mixing various new algorithms, such as conditional random fields (CRF) [25–27], atrous convolution [28], atrous spatial pyramid pooling (ASPP) [29,30], spatial pyramid pooling (SPP) [31] and encode-decoder structure, to the deep convolutional neural networks (DCNNs). The latest version of DeepLab V3+ released in 2018 has a mean intersection over union (MIoU) of 89% in the PASCAL visual object classes (VOC) [32] dataset. In this study, we selected the DeepLab V3+ as the basic network model of training. However, a limitation of applying the DeepLab model is it only allows to input one-channel or three-channel data as training samples. If we need to use optical remote sensing images containing red, green, and blue channels information and additionally the DEM data, there will be four channels information, which is not accepted by the currently used DeepLab V3+ model. In order to extract information of karst landscapes using jointly remote sensing and DEM data for higher accuracy, the input layer of DeepLab V3+ model needs to be improved so that the network could accept at least four-channel data. Therefore, in this study we attempt to develop an automatic method to extract spatial distribution of cone karst landscape by combining satellite remote sensing images and DEM data based on the deep learning model DeepLab V3+ in order to improve landscape classification accuracy and efficiency. The South China Karst, with an area of approximately 550,000 km2, as the world’s most spectacular example of humid tropical to sub-tropical karst landscape, was named as a United Nations Educational, Scientific and Cultural Organization (UNESCO) world natural heritage property in 2007 and 2014, respectively. It includes seven karst clusters, namely, Shilin, Libo, Wulong, Guilin, Shibing, Jinfoshan, and Huanjiang Karsts, which contain the most typical types of karst landforms, including tower karst, pinnacle karst, cone karst and so on. We applied the developed method to classify cone karst in Libo, which is a representative of tropical cone karst landscape in the world. Following the Introduction, detailed description of the Libo study area is presented in Section2. In Section3, the developed methodology to extract cone karst landscape using both satellite remote sensing images and DEM data based on the DeepLab V3+ model is described. Then, the segmentation results of cone karst landscape in Libo using the developed method are evaluated. To further highlight the advantage of using the DL method to classify cone karst landscape, we also conducted the same experiment but using the classical classification method, i.e., Support Vector Machine (SVM) and compared with Remote Sens. 2021, 13, 441 3 of 16 the results achieved using the DeepLab V3+ model. In Section5, we briefly discussed the reason that combing both satellite remote sensing images with DEM data can significantly improve accuracy of segmentation of cone karst landscape. The conclusions are drawn in the last section. 2. Study Area The Libo cone karst landscape, as one of South China Karst UNESCO heritage sites in 2007, is located in the southernmost part of Guizhou Province, with an area of 2431.8 km2 (107◦37’~108◦39’E, 25◦06’~25◦39’N). Due to influence of tectonic deformation during the Cenozoic era,
Details
-
File Typepdf
-
Upload Time-
-
Content LanguagesEnglish
-
Upload UserAnonymous/Not logged-in
-
File Pages16 Page
-
File Size-