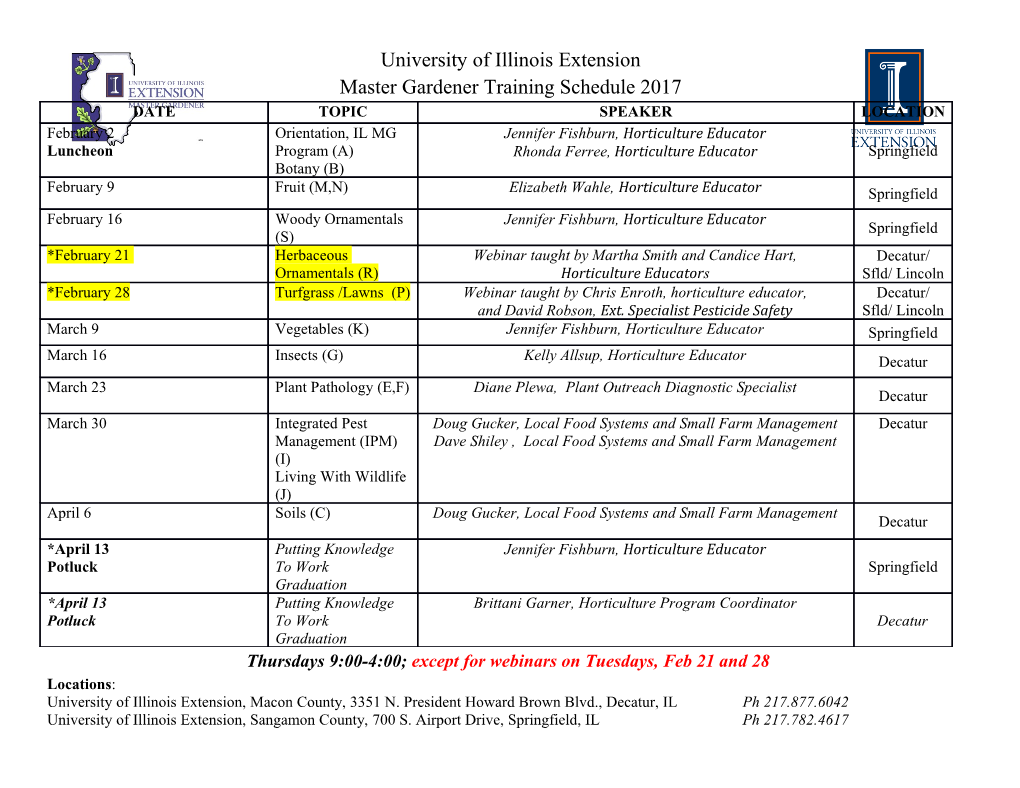
Appl. Math. Inf. Sci. Lett. 6, No. 3, 113-121 (2018) 113 Applied Mathematics & Information Sciences Letters An International Journal http://dx.doi.org/10.18576/amisl/060303 An Advanced Count Data Model with Applications in Genetics Zahoor Ahmad*, Adil Rahsid and T. R. Jan. P.G Department of Statistics, University of Kashmir, Srinagar, India Received: 19 Jan. 2018, Revised: 10 Mar.2018, Accepted: 15 Mar.2018. Published online: 1 Sep. 2018. Abstract:The present paper introduces an advanced count model which is obtained by compounding generalized negative binomial distribution with Kumaraswamy distribution. The proposed model has several properties such as it can be nested to different existing compound distributions on specific parameter setting. Similarity of the proposed model with existing compound distribution has been shown by means of reparameterization. The properties of the new model are discussed and explicit expressions are derived for the factorial moments. Further method of moments and maximum likelihood estimation is used to evaluate the moments. The potentiality of the proposed model has been tested by chi-square goodness of fit test by modeling the real world count data sets from genetics. Keywords: Generalized negative binomial distribution, Kumaraswamy distribution, compound distribution, factorial moment, count data. 1 Introduction distribution. In several research papers it has been found that compound Distributions are very flexible and can be used efficiently to Statistical distributions are commonly applied to describe model different types of data sets. With this in mind many real world phenomenon. Dueto the usefulness of statistical compound probability distributions have been constructed. distributions their theory is widely studied and new Sankaran (1970) obtained a compound of Poisson distributions are developed. There has been an increased distribution with that of Lindley distribution, Zamani and interest in developing generalized or generated families of Ismail (2010) constructed a new compound distribution by new continuous distributions by introducing one or more compounding negative binomial with one parameter additional shape parameters. The popularity and the use of Lindley distribution that provides good fit for count data these new families of distributions have attracted the where the probability at zero has a large value. The attention of statisticians, engineers, economists, researchers like Adil Rashid and Jan obtained several demographers and other applied researchers. The reason compound distributions for instance, (2013) a compound of might be that this additional parameter has proved to be zero truncated generalized negative binomial distribution helpful in improving the goodness-of-fit of the proposed with generalized beta distribution, (2014a) they obtained family of continuous distributions and they have the ability compound of Geeta distribution with generalized beta to fit skewed data better than existing distributions. There distribution and (2014b) compound of Consul distribution exists many generalized family of continuous distributions with generalized beta distribution. Most recently that have received a great deal of attention in recent years. AdilRashid and Jan (2014c) explored a mixture of We discuss below some generalized continuous generalized negative binomial distribution with that of distributions. generalized exponential distribution which contains several From the last few decades researchers are busy to obtain compound distributions as its sub cases and proved that new probability distributions by using different techniques this particular model is better in comparison to others when such as compounding, T-X family, transmutation etc. but it comes to fit observed count data set. compounding of probability distribution has received In this paper we propose a new count data model by maximum attention which is an innovative and sound compounding generalized negative binomial distribution technique to obtain new generalized probability (NBD) with Kumaraswamy distribution (KSD) and some distributions. The compounding of probability distributions similarities of the proposed model will be shown with some enables us to obtain both discrete as well as continuous *Corresponding author e-mail: [email protected] © 2018 NSP Natural Sciences Publishing Cor. 114 Z. Ahmed et al.: An advanced count data model … already existing compound distribution. r ( 1)1 r 2 Material and Methods E(X ) (3) r 1 A discrete random variable is X said to have a generalized negative binomial distribution (GNBD) with parameters Kumaraswamy distribution is a two parameter continuous m, p and if its probability mass function is given by probability distribution that has obtained by Kumaraswamy (1980) but unfortunately this distribution is not very m m x x mxx f1(x;m, p,) (1 p) p , x 0,1,2,... popular among statisticians because researchers have not m x x analyzed and investigated it systematically in much detail. (1) Kumaraswamy distribution is similar to the beta Where distribution but unlike beta distribution it has a closed form of cumulative distribution function which makes it very 0 p 1, m 0, p 1; 1, simple to deal with. For more detailed properties one can 0 p 1, m N , 0. see references Kumaraswamy (1980) and Jones (2009). Usually the parameters m, p,and in GNBD are fixed constants but here we have considered a problem in which For 1, equation (1) reduces to the negative binomial the probability parameter p is itself a random variable distribution (NBD). If m N , for 0, one obtains following KSD with p.d.f (2). from (1) the binomial distribution (BD) and for 1 , the Pascal distribution N.Johnson, S.Kotz and A. Kemp 3 Definition of Proposed Model (1992,p.200). A random variable X is said to have a Kumaraswamy If X | p~ GNBD m, p, where p is itself a random distribution (KSD) if its pdf is given by variable following Kumaraswamy distribution KSD , then determining the distribution that results from 1 1 marginalizing over p will be known as a compound of f 2 X ; , x 1 x , 0 x 1 (2) generalized negative binomial distribution with that of Kumaraswamy distribution which is denoted by GNBKSD Where , 0are shape parameters The raw moments of m, ,, It may be noted that proposed model will be Kumaraswamy distribution are given by a discrete since the parent distribution GNBD is discrete. Theorem 3.1: The probability mass function of a 1 compound of GNBD p, ,m with KSD (,) is given E(X r ) x r f (X ;, )dx 2 by 0 Proof: Using the definition (3), the p.m.f of a compound of GNBD m, p, with KSD can be obtained as 1 f X ;m,, , f x | p f p dp GNBKSD 1 2 0 x 1 m m x x j m jxx1 1 f X ;m,, , 1 p 1 p dp GNBKSD m x x j0 j 0 substituting 1 p y , we get m m x x x 1 m jxx f X ;m,, , 1 j y 1 (1 y) dy GNBKSD m x x j j0 0 © 2018 NSP Natural Sciences Publishing Cor. Appl. Math. Inf. Sci. Lett. 6, No. 3, 113-121 (2018) / http://www.naturalspublishing.com/Journals.asp 115 x m m x x j m j x x f X ;m,, , 1 B , 1 GNBKSD m x x j0 j m j x x x ( 1) 1 m m x x j f X ;m,, , 1 (4) GNBKSD m x x j m j x x j0 1 where . From here a random X variable following a compound of GNBD with KSD f (X ;m,, ,) f x | f , ,d will be symbolized by GNBKSD m, ,, GNBGED 1 3 0 4 ReparameterizationTechniques Proposition 4.1: The probability function of the proposed model gets coincide with the compound of GNBD with There are very few continuous probability distributions in GED obtained through reparameterization. statistics whose support lies between 0 and 1 so in order to ascribe a suitable distribution to a probability parameter p we have a limited choice, to remove this limitation Proof: If X |~GNBDm, p e , where is researchers try to reparameterize the probability parameter itself a random variable following a generalized exponential distribution (GED) with probability density by equating to where is a random variable. e 0 function So instead of ascribing a suitable probability distribution to parameter p researchers ascribe a suitable distribution to 1 f ( ; ,) 1 e e , 0 for the parameter by treating it as a random variable with 3 support 0 and there are numerous probability , 0 (5) distributions in statistics whose support lies 0,.In this then determining the distribution that results from section a similarity will be shown between a proposed marginalizing over will give us a compound of GNBD model and a model which is obtained by compounding generalized negative binomial distribution with generalized with GED exponential distribution through reparameterization technique. m m x x x f (X ;m,, ,) ( 1) j e(m jxx) f ; , d NBGED 3 m x x j0 j 0 m j x x x 1 1 m m x x j f (X ;m,, ,) (1) (6) NBGED m x x j m j x x j0 1 Where x 0,1,2,...,m,, , 0 . Interestingly, this Hence our model can be treated as a simple and easy alternative since it has been obtained without gives rise to the same probability function as the probability reparameterization.function defined in (6) was obtained by function (4) of the proposed model. The probability Adil Rashid and Jan (2014) © 2018 NSP Natural Sciences Publishing Cor. 116 Z. Ahmed et al.: An advanced count data model … j 1 1 1 5 Nested Distributions x x f X ; , 1 j GKSD j j 1 In this particular section we show that the proposed model j0 1 can be nested to different models under specific parameter setting. Setting 1we get a compound of negative binomial Proposition 5.1: If X ~ GNBKSD m, , , then by distribution with Kummarswamy distribution.
Details
-
File Typepdf
-
Upload Time-
-
Content LanguagesEnglish
-
Upload UserAnonymous/Not logged-in
-
File Pages9 Page
-
File Size-