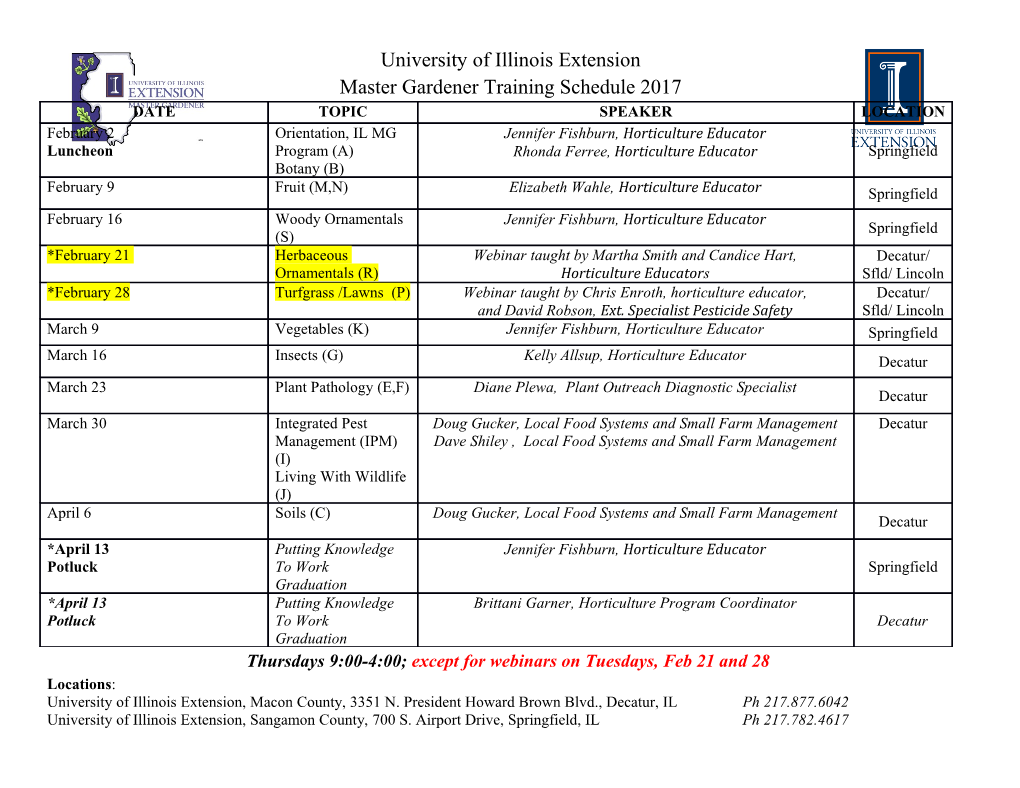
PHYSICAL REVIEW RESEARCH 3, L032049 (2021) Letter Expressivity of quantum neural networks Yadong Wu,1 Juan Yao,2 Pengfei Zhang,3,4,* and Hui Zhai 1,† 1Institute for Advanced Study, Tsinghua University, Beijing, 100084, China 2Guangdong Provincial Key Laboratory of Quantum Science and Engineering, Shenzhen Institute for Quantum Science and Engineering, Southern University of Science and Technology, Shenzhen 518055, Guangdong, China 3Institute for Quantum Information and Matter, California Institute of Technology, Pasadena, California 91125, USA 4Walter Burke Institute for Theoretical Physics, California Institute of Technology, Pasadena, California 91125, USA (Received 24 April 2021; accepted 9 August 2021; published 23 August 2021) In this work, we address the question whether a sufficiently deep quantum neural network can approximate a target function as accurate as possible. We start with typical physical situations that the target functions are physical observables, and then we extend our discussion to situations that the learning targets are not directly physical observables, but can be expressed as physical observables in an enlarged Hilbert space with multiple replicas, such as the Loschmidt echo and the Rényi entropy. The main finding is that an accurate approximation is possible only when all the input wave functions in the dataset do not span the entire Hilbert space that the quantum circuit acts on, and more precisely, the Hilbert space dimension of the former has to be less than half of the Hilbert space dimension of the latter. In some cases, this requirement can be satisfied automatically because of the intrinsic properties of the dataset, for instance, when the input wave function has to be symmetric between different replicas. And if this requirement cannot be satisfied by the dataset, we show that the expressivity capabilities can be restored by adding one ancillary qubit at which the wave function is always fixed at input. Our studies point toward establishing a quantum neural network analogy of the universal approximation theorem that lays the foundation for expressivity of classical neural networks. DOI: 10.1103/PhysRevResearch.3.L032049 I. INTRODUCTION not been fully explored and there are only few works in this direction [13,32]. Neural networks lie at the center of the recent third wave Here we consider the quantum generalizations of fully of artificial intelligence. The universal approximation theorem connected neural networks, which contain quantum wave plays an essential role in the development of neural net- functions of n-qubit states as inputs, parameterized quantum works, which states that sufficiently wide or sufficiently deep circuits made of local quantum gates, and measurements on neural networks can approximate a well-behaved function d readout qubits leading to labels. The parameters in the quan- on d-dimensional Euclidean space R with arbitrary accu- tum circuit will be optimized during training that yields the racy [1]. This theorem lays the foundation of the expressive best approximation of the learning target. Since the Hilbert capability of neural networks and serves as bases for the space dimension of an n-qubit state is 2n, the wave function successes of neural network applications. Quantum neural can encode information of d = 2n complex numbers, up to a networks (QNNs) are quantum generalizations of classical normalization condition and a global phase. In the following, feedforward neural networks on future quantum computers concerning the effects of the depth and the width on the ex- [2–5], which lie at the center of the recent development of the pressive capability of a QNN, we address the question whether quantum machine learning, including quantum unsupervised a sufficiently deep QNN and a sufficiently wide QNN can learning [6–11], quantum generalization of neural networks express any well-behaved function in the Cd space. [12–21], quantum circuit structures developed by classical To be concrete, here we consider a number of typical learn- neural networks [22–29], and information theory in quantum ing tasks in the quantum physics problem, where the learning neural networks [30,31]. However, expressivity of QNNs has targets include (i) physical observables; (ii) the Loschmidt echo, and (iii) the Rényi entropy. We point out that, in contrast to the universal approximation theorem for classical neural networks, a QNN cannot express a general well-behaved func- tion with arbitrary accuracy even though the QNN is made *[email protected] sufficiently deep. However, we show in this work that, by †[email protected] enlarging the Hilbert space dimension of the input state, this Published by the American Physical Society under the terms of the problem can be solved, and the expressivity can be signifi- Creative Commons Attribution 4.0 International license. Further cantly improved or can even be made as accurate as possible. distribution of this work must maintain attribution to the author(s) Enlarging the Hilbert space dimension effectively increases and the published article’s title, journal citation, and DOI. the width of the QNN. This can be achieved either by adding 2643-1564/2021/3(3)/L032049(6) L032049-1 Published by the American Physical Society WU, YAO, ZHANG, AND ZHAI PHYSICAL REVIEW RESEARCH 3, L032049 (2021) an ancillary qubit in the input and (or) by duplicating replicas quantum circuits leads to of the input wave functions. These results point toward an y˜l =ψl |Uˆ †Mˆ Uˆ|ψl . (3) analogy of the universal approximation theorem for QNN. The loss function is taken as L = 1 |y˜l − yl |2, which ND l II. RESULTS enforcesy ˜l to be yl for all |ψl . And we use the Adams method to optimize the coefficient of the generators of each two-qubit We consider a dataset denoted as {(|ψl , yl )}, {l = ,..., } gate during training [31]. 1 ND , where l labels data and ND is the total number Here one thing that should be noticed is whether the input |ψl of data in the dataset. Each input quantum state can wave functions span the entire Hilbert space, which requires |ψl = l | {| } be written as m cm m , where m is a complete N > 2n.HereN denotes the total number of data in the n { l } n D D set of 2 bases of the n-qubit Hilbert space, and cm are 2 training set and 2n is the dimension of the Hilbert space normalized complex numbers with a fixed total phase. Usually n containing n qubits. If ND < 2 , all the input wave functions the information in the label is much more condensed than the in the dataset only occupy a subset of the entire Hilbert space. information of the entire input, therefore, here we consider In some cases, even when N > 2n, if the wave functions have l ∈ − , D that the label is simply a number y [ 1 1]. To motivate this certain structures, for instance, if the wave functions are taken result, let us first start with a simpler situation that the label is as ground states of certain Hamiltonians [30,31], they also l =ψl | ˆ|ψl ˆ a physical observable y O , where O is a hermitian do not span the entire Hilbert space. However, if N > 2n operator on n-qubit quantum state [33]. This is equivalent to D l and the input wave functions are general enough, they span say thaty is a quadratic function of these complex numbers as l ∗ ∗ the entire Hilbert space. In this case, in order for ally ˜ to l = l l = = | ˆ| y mm Omm cm cm , where Omm Omm m O m .The faithfully represent yl , one requires Oˆ = Uˆ †Mˆ Uˆ. However, QNN we considered is shown in Fig. 1(a). this is not possible for a general operator Oˆ. Because Mˆ is A unitary Uˆ is made of a number of two-qubit gates. We a direct product of theσ ˆx operator on the read-out qubit-r and useu ˆij to denote a two-qubit gate acting on qubit-i and - j. the n − 1 identity operators on the rest qubits, the eigenval- Eachu ˆij is parameterized as ues of Mˆ consist 2n−1 number of −1 and equal number of + αk gˆ 1, and any unitary transformation keeps these eigenvalues uˆ = e k ij k , (1) ij invariant. That is to say, even though one can make the QNN k deep enough to present a generic unitary Uˆ in the SU(2n ) whereg ˆk are SU(4) generators and α are parameters. In a ij = Uˆ † Uˆ QNN, these parameters need to be determined by training. We group, it always cannot satisfy Oˆ Mˆ . This argument can be easily generalized to situations that measurements are use the brick wall architecture to arrange theseu ˆij to form Uˆ. To make sure that the QNN can realize any kinds of unitary performed in more than one readout qubit. transformations, we set the circuit depth sufficiently large. The entire quantum circuit Uˆ acts on the input wave function |ψl A. Ancillary qubit σ and then we perform a measurement, say x, on the readout Now we show this problem can be solved by adding one qubit-r. ancillary qubit. Instead of |ψl , we now add one ancillary The measurement operator is therefore denoted by qubit and the input wave function is set as |α⊗|ψl , where |α Mˆ = σˆ 1 ⊗···⊗σˆ r ⊗···⊗σˆ N , (2) the input state at the ancillary qubit is always fixed as . 0 x 0 The unitary Uˆ now acts on the entire 2n+1-dimensional Hilbert where the superscription i = 1,...,N denotes the qubits, space, and the measurement is still performed in the readout σ i and 0 denotes the identity matrix. The measurement of the qubit and now ˜ˆ = σ a ⊗ σ 1 ⊗···⊗σ r ⊗···⊗σ N , M ˆ0 ˆ0 ˆx ˆ0 (4) (a) (b) a where the superscript a denotes the ancillary qubit.
Details
-
File Typepdf
-
Upload Time-
-
Content LanguagesEnglish
-
Upload UserAnonymous/Not logged-in
-
File Pages6 Page
-
File Size-