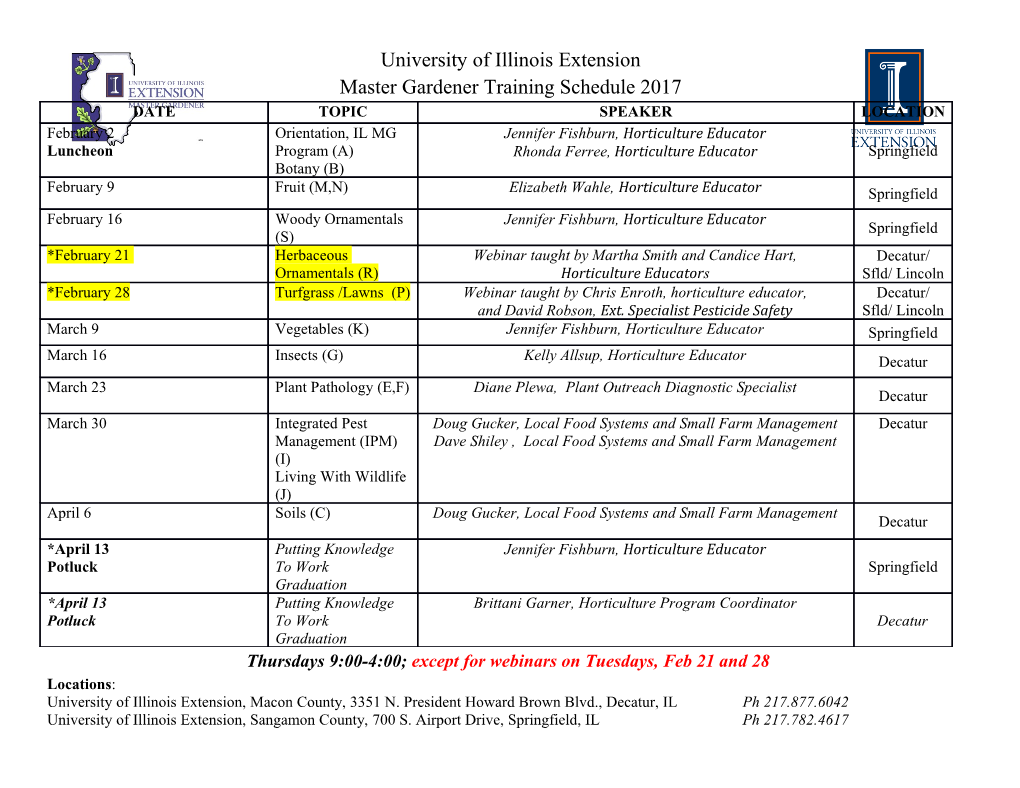
Undoing Software Engineering: Demodularization of a SGLR Parser for Performance Gains Nik Kapitonenko TU Delft Abstract avoided by removing unnecessary architecture supporting the modularization. This ‘inlining’ may simplify the way com- JSGLR2 is a java implementation of the Scanner- ponents interact on a lower abstraction level, which may im- less Generalized LR-parsing (SGLR) algorithm. It prove parsing throughput. employs a modular architecture. This architec- This paper presents four inlined versions of one the JS- ture comes with a performance overhead for letting GLR2 variants, called “recovery”. A copy of the “recovery multiple components interact with each other. This variant” is created, and refactors it be be less modular. The paper looks into the size of the performance over- four inlined versions share a common base, but have slight head penalty for the recovery parser variant. It does differences in how they inline certain components. Next, us- so by creating an ‘inlined’ version of the recovery ing a dedicated evaluation suite, this paper presents the per- parser variant. The inlined recovery variant is a formance results of the original and the inlined variants. The JSGLR2 implementation that ignores the modular inlined variants are compared to the base case and each other. architecture, and hard-codes the components. The From the results, conclusions are drawn on which inlined performance of the inlined variant is measured with variants are the most successful. a pre-existing evaluation suite. The results show that there is a performance increase between the 1.3 Organization original, and the inlined variant. The paper is organized as follows into several sections. Sec- tion 2 provides a more in-depth background on the JSGLR2 1 Introduction recovery parser. It gives an overview of SLGR parsing. Sec- tion 3 describes the methodology, and what the inlining pro- 1.1 Context cess consisted of. It also discusses the differences between Parsing is a fundamental component of processing most pro- the four proposed variants, and why four different variants gramming and markup languages. As various projects can get were created in the first place. Section 4 describes the eval- a large amount of source code, it is important that parsing is uation suite and discusses the results. Section 5 touches on as fast as possible to reduce build times. At the same time, reproducibility of the results. Section 6 summarizes the pa- it is convenient if a single parser implementation can han- per, and suggests future work. Finally, the appendix contains dle a large variety of languages. JSGLR2 [Denkers, 2018] most figures, including the results. is a Scannerless Generalized LR-parser implementation that can parse any context free language at practical speeds. It 2 Background manages the algorithm’s complexity by splitting the algo- rithm into multiple components of a manageable size, each 2.1 LR Parsing of which receive optimizations sourced from various scien- JSGLR2 is a Scannerless Generalized LR-parser. Visser tific papers and other sources. The components are then com- [Visser, 1997] describes it as “the integration and improve- posed together using dependency injection. A factory design ment of scannerless parsing, generalized-LR parsing and pattern provides several different compositions, each provid- grammar normalization”. LR-parsing forms the base of the ing a different feature set and optimizations. JSGLR2 shows SLGR algorithm. LR-parsing is based on Knuth’s LR pars- a 3x speedup for parsing Java source code, compared to JS- ing algorithm [Knuth, 1965]. It parses a subset of context free GLR1 [Denkers, 2018]. languages, noted as “LR(k)” grammars. It is based on con- suming input tokens one by one, and updating a state machine 1.2 The work with a stack. The state then tells which of the three functions However, to achieve the modularity, the JSGLR2 implemen- to perform: shifting, reducing, or accepting [Denkers, 2018]. tation makes use of bridging architecture unrelated to the ac- Before the parsing can begin, the state machine needs to tual paring algorithm itself. It is possible that the modular be created. In JSGLR2, it is called the parse table [Denkers, architecture provides an performance overhead, that can be 2018]. It represents the grammar of the language to be parsed. As the parse table is not part of parsing itself, further discus- know whether to reduce the "x + x" into "(x + x)", or to sion on its creation is outside the scope of this work. What shift until the last x, and then reduce the multiplication first. is important to know, is that it is a map between a tuple of a The solution for this would be to designate some kind of oper- state with a token, and an action. ‘Shift’ and ‘Reduce’ action ator precedence, where one action is preferred over the other. state to which state to transition to next, while the ‘Accept’ However, in this example, if "x + (x * x)" is the action successfully ends parsing. proffered outcome, another issue appears. When deciding Parsing itself starts with a pre-processing step called scan- whether to shift or reduce the middle x, the parser so far has ning. It reads the input text, and turns it into a stream of only consumed "x + x". It does not know what the next tokens. A token represents a language primitive, such as an character is. It might be *, in which case the parser should number, an operator, or a keyword. Scanning strips out white- shift, or it might be actually End of Input, in which case the space, layout, comments, and other features not needed for parser should reduce. Knuth solved this with lookahead: in- the parser. The tokens are then fed into the parser. stead of using the currently parsed token to look up the next Parsing starts with the freshly generated stream of tokens, state in the parse table, The parser reads, but not consumes, and a stack containing one element: the start state of the parse the next k tokens and uses them all together to look the next table. The parser then iteratively reads the next token, and state [Knuth, 1965]. uses it together with the topmost state in the stack to retrieve While this works for most practical languages, in theory, the next action. it is possible to devise a grammar that requires more than k The shift action pushes a tuple on top of the stack. The tokens to resolve an ambiguity [Denkers, 2018]. Generalized tuple consists of a new state, and the token that triggered this LR-Parsing is an alternative solution to the ambiguity prob- action. The shift action lets the parser consume the input until lem [Visser, 1997]. It allows a parse table to return multiple a reduce action can be applied. states from a lookup. Then, for each return action, the parser The reduce action is where the ‘comprehension’ of the in- creates a new parallel stack, and applies a different action on put happens. It pops off one or more states from the stack, each action. The parser then continues updating all stacks in and combines their associated tokens into a composite token parallel. Effectively, this means that the parser tries all ac- called a parse node. The node represents what the tokens tions at the same time. If a single stack ends up being wrong mean together. For example, if the three top-most tokens were in some way, it can be discarded, while other stacks continue “Constant 1”, “Operation +”, “Variable x”, then a reduction working. The parser fails only when all stacks fail. that takes these three tokens could produce a parse node rep- To save space, the parallel stacks are stored in a Graph resenting “Value (1 + x)”. After reducing, the next state is Structured Stack (GSS) [Denkers, 2018]. The states in the looked up in the parse table. The input tuple is then the new stacks are stored as nodes. Directed edges point to the next topmost state, which was directly below the states that were element below the top state. This lets parallel stacks share removed for reducing, and the parse node as token. The found common states. state is then put on top with the stack, together with the parse node. Effectively, reducing replaces simpler tokens with a 2.3 Scannerless parsing single composite token. The action state signifies that parsing is done. Usually, it is Scannerless parsing, as the name implies, is a modification only accessible when the next token is End Of Input, which to the LR-parsing algorithm that removes the scanner. It is appended at the end of a token stream. It tells the parser to changes the parsing algorithm to work on characters directly. inspect the stack, and see if is represents a successful parse. One advantage of this is that now white-space and layout This is the case when the stack only contains two elements: can be part of the language grammar. However, the main is- the start state, and another state with an associated token. This sue that scannerless parsing solves are context sensitive key- int int = 1; C token then represents the full syntax tree that the parser is words. For example, take the string in a - supposed to generate. If there more than two states in the like language. Normally, the scanner would mark the second int stack, then parser signals a failure. as a keyword token, and then parsing would fail, as as- signing a value to a keyword does not make any sense. How- 2.2 Generalized parsing ever, with scannerless parsing, a grammar could be devised that would consider any alphanumeric characters between the While LR parsing is relatively fast and simple, it has one ma- first int and the = as a valid identifier.
Details
-
File Typepdf
-
Upload Time-
-
Content LanguagesEnglish
-
Upload UserAnonymous/Not logged-in
-
File Pages13 Page
-
File Size-