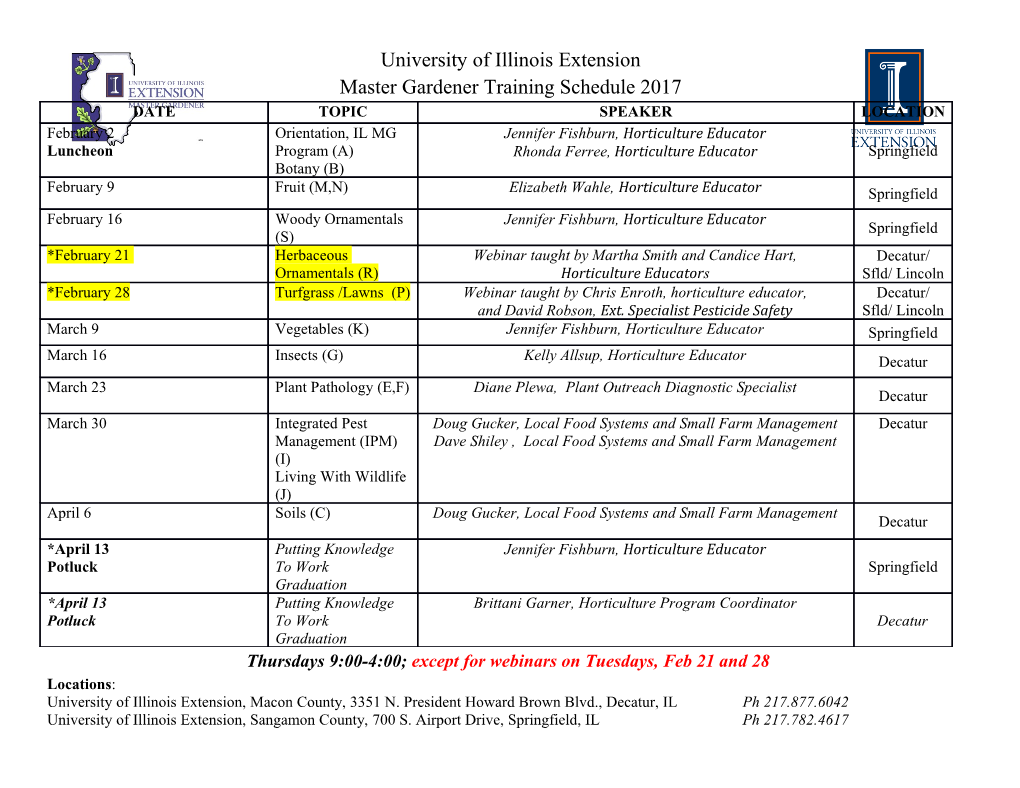
VIII. Approximation Algorithms: MAX-CUT Problem (Outlook) Thomas Sauerwald Easter 2017 Outline Simple Algorithms for MAX-CUT A Solution based on Semidefinite Programming Summary VIII. MAX-CUT Problem Simple Algorithms for MAX-CUT 2 cluster analysis VLSI design 2 b Weighted MAX-CUT: Every edge e 2 E has a non-negative weight w(e) a 1 3 5 4 c Given: Undirected graph G = (V ; E) 2 1 Goal: Find a subset S ⊆ V such that jE(S; V n S)j 2 h e 1 is maximized. g 3 Weighted MAX-CUT: Maximize the weights of edges crossing d P the cut, i.e., maximize w(S) := fu;vg2E(S;V nS) w(fu; vg) S = fa; b; gg w(S) = 18 Applications: Max-Cut MAX-CUT Problem VIII. MAX-CUT Problem Simple Algorithms for MAX-CUT 3 cluster analysis VLSI design 2 b Weighted MAX-CUT: Every edge e 2 E has a non-negative weight w(e) a 1 3 5 4 c 2 1 Goal: Find a subset S ⊆ V such that jE(S; V n S)j 2 h e 1 is maximized. g 3 Weighted MAX-CUT: Maximize the weights of edges crossing d P the cut, i.e., maximize w(S) := fu;vg2E(S;V nS) w(fu; vg) S = fa; b; gg w(S) = 18 Applications: Max-Cut MAX-CUT Problem Given: Undirected graph G = (V ; E) VIII. MAX-CUT Problem Simple Algorithms for MAX-CUT 3 cluster analysis VLSI design 2 b Weighted MAX-CUT: Every edge e 2 E has a non-negative weight w(e) a 1 3 5 4 c 2 1 2 h e 1 g 3 Weighted MAX-CUT: Maximize the weights of edges crossing d P the cut, i.e., maximize w(S) := fu;vg2E(S;V nS) w(fu; vg) S = fa; b; gg w(S) = 18 Applications: Max-Cut MAX-CUT Problem Given: Undirected graph G = (V ; E) Goal: Find a subset S ⊆ V such that jE(S; V n S)j is maximized. VIII. MAX-CUT Problem Simple Algorithms for MAX-CUT 3 cluster analysis VLSI design 2 b a 1 3 5 4 c 2 1 2 h e 1 g 3 Weighted MAX-CUT: Maximize the weights of edges crossing d P the cut, i.e., maximize w(S) := fu;vg2E(S;V nS) w(fu; vg) S = fa; b; gg w(S) = 18 Applications: Max-Cut Weighted MAX-CUT: Every edge e 2 E has a non-negative weight w(e) MAX-CUT Problem Given: Undirected graph G = (V ; E) Goal: Find a subset S ⊆ V such that jE(S; V n S)j is maximized. VIII. MAX-CUT Problem Simple Algorithms for MAX-CUT 3 cluster analysis VLSI design 2 b a 1 3 5 4 c 2 1 2 h e 1 g 3 d S = fa; b; gg w(S) = 18 Applications: Max-Cut Weighted MAX-CUT: Every edge e 2 E has a non-negative weight w(e) MAX-CUT Problem Given: Undirected graph G = (V ; E) Goal: Find a subset S ⊆ V such that jE(S; V n S)j is maximized. Weighted MAX-CUT: Maximize the weights of edges crossing P the cut, i.e., maximize w(S) := fu;vg2E(S;V nS) w(fu; vg) VIII. MAX-CUT Problem Simple Algorithms for MAX-CUT 3 cluster analysis VLSI design b a g S = fa; b; gg w(S) = 18 Applications: Max-Cut 2 b Weighted MAX-CUT: Every edge e 2 E has a non-negative weight w(e) a 1 3 MAX-CUT Problem 5 4 c Given: Undirected graph G = (V ; E) 2 1 Goal: Find a subset S ⊆ V such that jE(S; V n S)j 2 h e 1 is maximized. g 3 Weighted MAX-CUT: Maximize the weights of edges crossing d P the cut, i.e., maximize w(S) := fu;vg2E(S;V nS) w(fu; vg) VIII. MAX-CUT Problem Simple Algorithms for MAX-CUT 3 cluster analysis VLSI design w(S) = 18 Applications: Max-Cut 2 b Weighted MAX-CUT: Every edge e 2 E has a non-negative weight w(e) a 1 3 MAX-CUT Problem 5 4 c Given: Undirected graph G = (V ; E) 2 1 Goal: Find a subset S ⊆ V such that jE(S; V n S)j 2 h e 1 is maximized. g 3 Weighted MAX-CUT: Maximize the weights of edges crossing d P the cut, i.e., maximize w(S) := fu;vg2E(S;V nS) w(fu; vg) S = fa; b; gg VIII. MAX-CUT Problem Simple Algorithms for MAX-CUT 3 cluster analysis VLSI design Applications: Max-Cut 2 b Weighted MAX-CUT: Every edge e 2 E has a non-negative weight w(e) a 1 3 MAX-CUT Problem 5 4 c Given: Undirected graph G = (V ; E) 2 1 Goal: Find a subset S ⊆ V such that jE(S; V n S)j 2 h e 1 is maximized. g 3 Weighted MAX-CUT: Maximize the weights of edges crossing d P the cut, i.e., maximize w(S) := fu;vg2E(S;V nS) w(fu; vg) S = fa; b; gg w(S) = 18 VIII. MAX-CUT Problem Simple Algorithms for MAX-CUT 3 cluster analysis VLSI design Max-Cut 2 b Weighted MAX-CUT: Every edge e 2 E has a non-negative weight w(e) a 1 3 MAX-CUT Problem 5 4 c Given: Undirected graph G = (V ; E) 2 1 Goal: Find a subset S ⊆ V such that jE(S; V n S)j 2 h e 1 is maximized. g 3 Weighted MAX-CUT: Maximize the weights of edges crossing d P the cut, i.e., maximize w(S) := fu;vg2E(S;V nS) w(fu; vg) S = fa; b; gg w(S) = 18 Applications: VIII. MAX-CUT Problem Simple Algorithms for MAX-CUT 3 VLSI design Max-Cut 2 b Weighted MAX-CUT: Every edge e 2 E has a non-negative weight w(e) a 1 3 MAX-CUT Problem 5 4 c Given: Undirected graph G = (V ; E) 2 1 Goal: Find a subset S ⊆ V such that jE(S; V n S)j 2 h e 1 is maximized. g 3 Weighted MAX-CUT: Maximize the weights of edges crossing d P the cut, i.e., maximize w(S) := fu;vg2E(S;V nS) w(fu; vg) S = fa; b; gg w(S) = 18 Applications: cluster analysis VIII. MAX-CUT Problem Simple Algorithms for MAX-CUT 3 Max-Cut 2 b Weighted MAX-CUT: Every edge e 2 E has a non-negative weight w(e) a 1 3 MAX-CUT Problem 5 4 c Given: Undirected graph G = (V ; E) 2 1 Goal: Find a subset S ⊆ V such that jE(S; V n S)j 2 h e 1 is maximized. g 3 Weighted MAX-CUT: Maximize the weights of edges crossing d P the cut, i.e., maximize w(S) := fu;vg2E(S;V nS) w(fu; vg) S = fa; b; gg w(S) = 18 Applications: cluster analysis VLSI design VIII. MAX-CUT Problem Simple Algorithms for MAX-CUT 3 E [ w(S; V n S)] 2 3 X = E 4 w(fu; vg) 5 fu;vg2E(S;V nS) X = Pr [ fu 2 S \ v 2 (V n S)g [ fu 2 (V n S) \ v 2 Sg ] · w(fu; vg) fu;vg2E X 1 1 = + · w(fu; vg) 4 4 fu;vg2E 1 X 1 ∗ = w(fu; vg) ≥ w : 2 2 fu;vg2E We could employ the same derandomisation used for MAX-3-CNF. Proof: We express the expected weight of the random cut (S; V n S) as: Random Sampling Ex 35.4-3 Suppose that for each vertex v, we randomly and independently place v in S with probability 1=2 and in V n S with probability 1=2. Then this algorithm is a randomized 2-approximation algorithm. VIII. MAX-CUT Problem Simple Algorithms for MAX-CUT 4 We could employ the same derandomisation used for MAX-3-CNF. E [ w(S; V n S)] 2 3 X = E 4 w(fu; vg) 5 fu;vg2E(S;V nS) X = Pr [ fu 2 S \ v 2 (V n S)g [ fu 2 (V n S) \ v 2 Sg ] · w(fu; vg) fu;vg2E X 1 1 = + · w(fu; vg) 4 4 fu;vg2E 1 X 1 ∗ = w(fu; vg) ≥ w : 2 2 fu;vg2E Random Sampling Ex 35.4-3 Suppose that for each vertex v, we randomly and independently place v in S with probability 1=2 and in V n S with probability 1=2. Then this algorithm is a randomized 2-approximation algorithm. Proof: We express the expected weight of the random cut (S; V n S) as: VIII. MAX-CUT Problem Simple Algorithms for MAX-CUT 4 We could employ the same derandomisation used for MAX-3-CNF. 2 3 X = E 4 w(fu; vg) 5 fu;vg2E(S;V nS) X = Pr [ fu 2 S \ v 2 (V n S)g [ fu 2 (V n S) \ v 2 Sg ] · w(fu; vg) fu;vg2E X 1 1 = + · w(fu; vg) 4 4 fu;vg2E 1 X 1 ∗ = w(fu; vg) ≥ w : 2 2 fu;vg2E Random Sampling Ex 35.4-3 Suppose that for each vertex v, we randomly and independently place v in S with probability 1=2 and in V n S with probability 1=2. Then this algorithm is a randomized 2-approximation algorithm.
Details
-
File Typepdf
-
Upload Time-
-
Content LanguagesEnglish
-
Upload UserAnonymous/Not logged-in
-
File Pages137 Page
-
File Size-