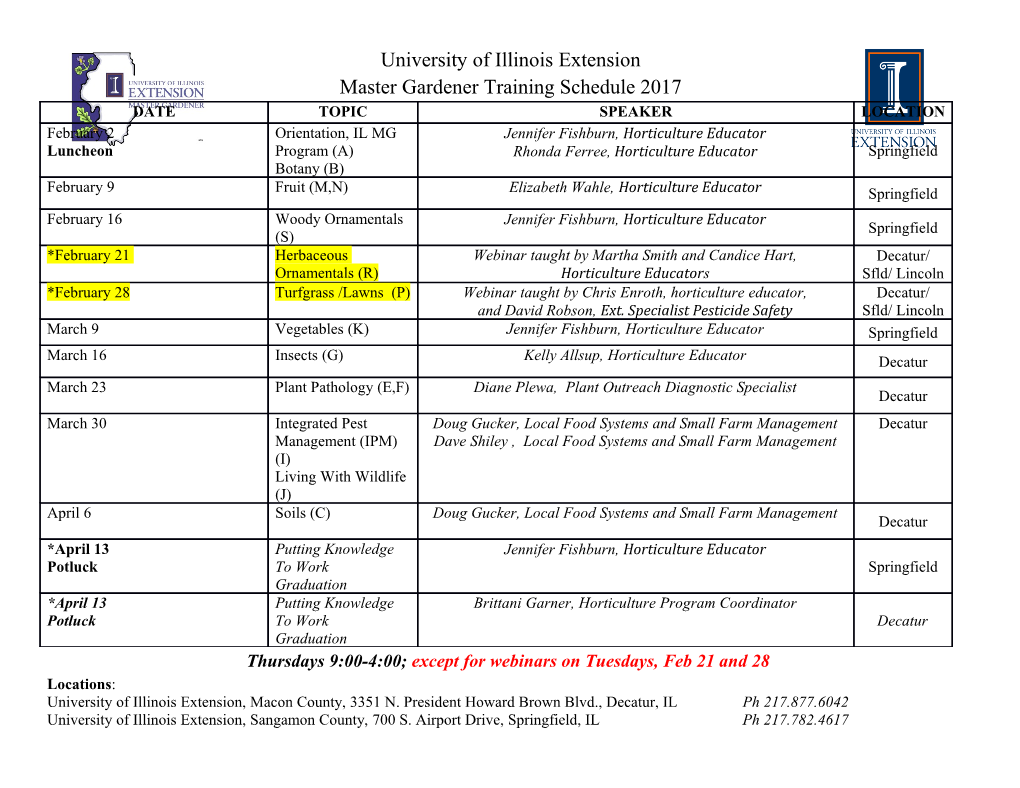
An Unsupervised Query Rewriting Approach Using N-gram Co-occurrence Statistics to Find Similar Phrases in Large Text Corpora Hans Moen1, Laura-Maria Peltonen2, Henry Suhonen2;3, Hanna-Maria Matinolli2, Riitta Mieronkoski2, Kirsi Telen2, Kirsi Terho2;3, Tapio Salakoski1 and Sanna Salantera¨2;3 1Turku NLP Group, Department of Future Technologies, University of Turku, Finland 2Department of Nursing Science, University of Turku, Finland 3Turku University Hospital, Finland fhanmoe,lmemur,hajsuh,hmkmat, ritemi,kikrte,kmterh,sala,[email protected] Abstract multi-word phrases and expressions, possible vari- ations in word use, word count and word order We present our work towards developing a complicate things further (e.g. “consume food” vs system that should find, in a large text cor- “food and eating” or “DM II” vs “type 2 diabetes pus, contiguous phrases expressing sim- mellitus”). ilar meaning as a query phrase of arbi- An important task for a search engine is to trary length. Depending on the use case, try to bridge the gap between user queries and this task can be seen as a form of (phrase- how associated phrases of similar meaning (se- level) query rewriting. The suggested ap- mantics) are written in the targeted text. In this pa- proach works in a generative manner, is per we present our work towards enabling phrase- unsupervised and uses a combination of level query rewriting in an unsupervised manner. a semantic word n-gram model, a statisti- Here we explore a relatively simple generative ap- cal language model and a document search proach, implemented as a prototype (search) sys- engine. A central component is a distri- tem. The task of the system is, given a query butional semantic model containing word phrase as input, generate and suggest as output n-grams vectors (or embeddings) which contiguous candidate phrases from the targeted models semantic similarities between n- corpus that each express similar meaning as the grams of different order. As data we use query. These phrases, input and output, may be a large corpus of PubMed abstracts. The of any length (word count), and not necessarily presented experiment is based on man- known as such before the system is presented with ual evaluation of extracted phrases for ar- the query. Ideally, all unique phrases with similar bitrary queries provided by a group of meaning as the query should be identified. For ex- evaluators. The results indicate that the ample, the query might be: “organizational char- proposed approach is promising and that acteristics of older people care”. This exact query the use of distributional semantic models phrase may or may not occur in the target corpus. trained with uni-, bi- and trigrams seems Regardless, a phrase candidate of related mean- to work better than a more traditional uni- ing that we want our system to identify in the tar- gram model. geted corpus could then be: “community care of 1 Introduction elderly”. In this example, the main challenges that we are faced with are: 1) how can we identify When searching to see if some information is these four words as a relevant phrase, and 2) de- found in a text corpus, it may be difficult to for- cide that its meaning is similar to that of the query. mulate search queries that precisely match all rel- Depending on the use case, the task can be seen as evant formulations expressing the same informa- a form of query rewriting/substitution, paraphras- tion. This becomes particularly difficult when the ing or a restricted type of query expansion. Rel- information is expressed using multiple words, as evant use cases include information retrieval, in- a phrase, due to the expressibility and complex- formation extraction, question–answering and text ity of natural language. Single words may have summarization. We also aim to use this functional- several synonyms, or near synonyms, which re- ity to support manual annotation. For that purpose fer to the same or similar underlying concept (e.g. the system will be tasked with finding phrases “school” vs “gymnasium”). When it comes to that have similar meaning as exemplar phrases and queries provided by the user, and/or as previously nington et al., 2014) are nowadays commonly used annotated text spans. An unsupervised approach to (pre-)train word embeddings for further use like we are aiming for would be particularly valu- in various NLP tasks, including supervised text able for corpora and in domains that lack relevant classification with neural networks. However, re- labeled training data, e.g. in the form of search cent methods such as ELMo (Peters et al., 2017) history logs, needed for supervised paraphrasing and BERT (Devlin et al., 2018) use deep neural and query rewriting approaches. networks to represent context sensitive word em- The presented system relies on a combination beddings, which achieves state-of-the-art perfor- of primarily three components: A distributional mance when used in supervised text classification semantic model of word n-gram vectors (or em- and similar. beddings), containing unigrams, bigrams and tri- Further, there are several relatively recent works grams; A statistical language model; And a doc- focusing on using and/or representing n-gram in- ument search engine. Briefly explained, the way formation as semantic vectors (see e.g. Bo- the system works is by first generating a set of janowski et al. (2016); Zhao et al. (2017); Po- plausible phrase (rewrite) candidates for a given liak et al. (2017); Gupta et al. (2019)), possibly query. This is done by first composing vector rep- to further represent clauses, sentences and/or doc- resentation(s) of the query, and then searching for uments (see e.g. Le and Mikolov (2014); Pagliar- and retrieving n-grams that are close by in the se- dini et al. (2018)) in semantic vector spaces. mantic vector space. These n-grams are then con- A relatively straight forward approach to iden- catenated to form the phrase candidates. In this tify and represent common phrases as vectors in process, the statistical language model helps to a semantic space is to first use some type of col- quickly discard phrases that are likely nonsensi- location detection. Here the aim is to identify se- cal. Next the phrases are ranked according to their quences of words that co-occur more often than similarity to the query, and finally the search en- what is expected by chance in a large corpus. One gine checks which phrase candidates actually exist can then train a semantic model where identified in the targeted corpus, and where. phrases are treated as individual tokens, on the Similar to Zhao et al. (2017) and Gupta et al. same level as words, like it is done in Mikolov (2019) we explore the inclusion of word n- et al. (2013). grams of different sizes in the same semantic In the works mentioned so far, the focus is on space/model. One motivation for this is that they distributional semantics for representing and cal- both found this to produce improved unigram rep- culating semantic similarity and relatedness be- resentations compared to only training with uni- tween predefined lexical units and/or of predefined gram co-occurrence statistics. Another motivation length (words/n-grams, collocations, clauses, sen- is that we want to use the model to not only re- tences, etc.). Dinu and Baroni (2014) and Tur- trieve unigrams that are semantically close to each ney (2014) take things a step further and approach other, but also bigrams and trigrams. the more complex and challenging task of us- ing semantic models to enable phrase generation. 2 Related Work Their aim is similar to ours: given an input query Unsupervised methods for capturing and modeling (phrase) consisting of k words, generate as output word-level semantics as vectors, or embeddings, t phrases consisting of l words that each expresses have been popular since the introduction of La- its meaning. Their approaches rely on applying tent Semantic Analysis (LSA) (Deerwester et al., a set of separately trained vector composition and 1990) around the beginning of the 1990s. Such decomposition functions able to compose a single word vector representations, where the underlying vector from a vector pair, or decompose a vector training heuristic is typically based on the distri- back into estimates of its constituent vectors, pos- butional hypothesis (Harris, 1954), usually with sibly in the semantic space of another domain or some form of dimension reduction, have shown language. to capture word similarity (synonymy and relat- Dinu and Baroni (2014) also apply vector com- edness) and analogy (see e.g. Agirre et al. (2009); position and decomposition in a recursive man- Mikolov et al. (2013)). Methods and toolkits like ner for longer phrases (t ≤ 3). Their focus is Word2Vec (Mikolov et al., 2013) and GloVe (Pen- on mapping between unigrams, bigrams and tri- grams. As output their system produce one vec- the customized training file, we put the source n- tor per word which represent the (to be) generated gram and one of its neighboring n-grams as target phrase. Here the evaluation primarily assumes context. The size of the sliding window is decided that t = 1, i.e. the nearest neighbouring word by how many neighboring (context) n-grams we in the semantic model, belonging to the expected include for each source n-gram. Overlap between word class, is extracted per vector to form the out- the source n-gram and target n-grams is allowed. put phrase. However, no solution is presented for However, we found that Word2Vecf only allows when t > 1 other than independent ranked lists of training using negative sampling. As an alternative semantically similar words to each vector.
Details
-
File Typepdf
-
Upload Time-
-
Content LanguagesEnglish
-
Upload UserAnonymous/Not logged-in
-
File Pages9 Page
-
File Size-