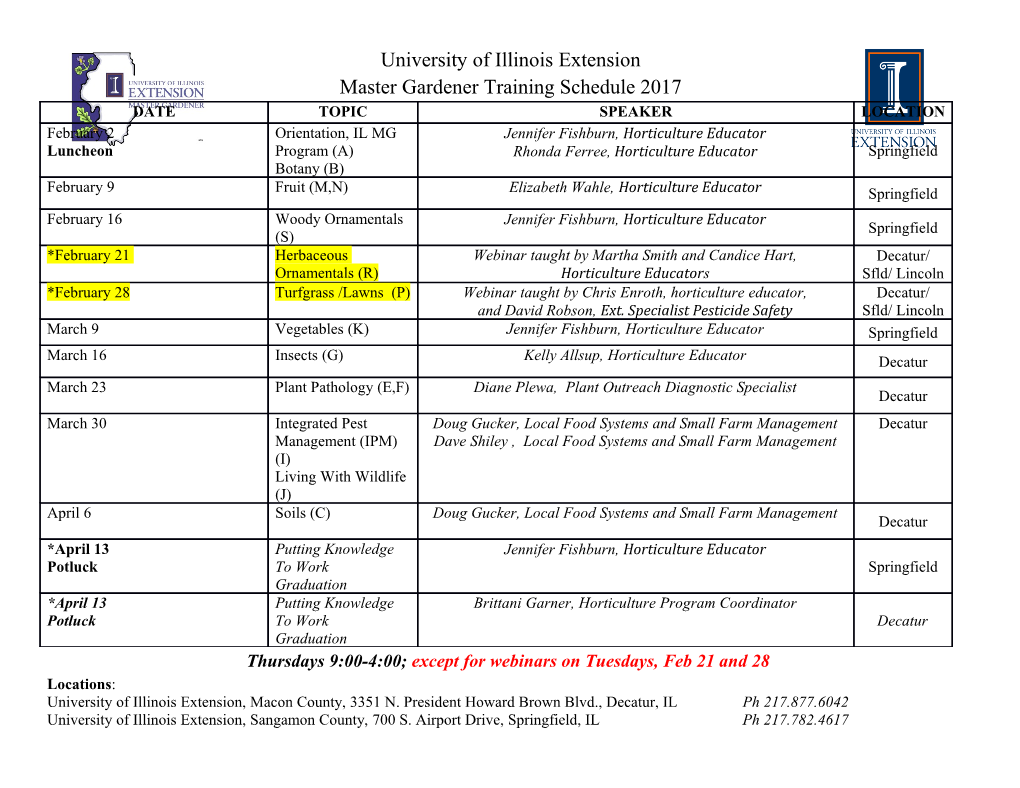
Query & Exploration SQL, Search, Cypher, … Stream Processing Platforms Data Storm, Spark, .. Data Ingestion Serving ETL, Distcp, Batch Processing Platforms BI, Cubes, Kafka, MapReduce, SparkSQL, BigQuery, Hive, Cypher, ... RDBMS, Key- OpenRefine, value Stores, … Data Definition Tableau, … SQL DDL, Avro, Protobuf, CSV Storage Systems HDFS, RDBMS, Column Stores, Graph Databases Computing Platforms Distributed Commodity, Clustered High-Performance, Single Node Query & Exploration SQL, Search, Cypher, … Stream Processing Platforms Data Storm, Spark, .. Data Ingestion Serving ETL, Distcp, Batch Processing Platforms BI, Cubes, Kafka, MapReduce, SparkSQL, BigQuery, Hive, Cypher, ... RDBMS, Key- OpenRefine, value Stores, … Data Definition Tableau, … SQL DDL, Avro, Protobuf, CSV Storage Systems HDFS, RDBMS, Column Stores, Graph Databases Computing Platforms Distributed Commodity, Clustered High-Performance, Single Node Computing Single Node Parallel Distributed Computing Computing Computing CPU GPU Grid Cluster Computing Computing A single node (usually multiple cores) Attached to a data store (Disc, SSD, …) One process with potentially multiple threads R: All processing is done on one computer BidMat: All processing is done on one computer with specialized HW Single Node In memory Retrieve/Stores from Disc Pros Simple to program and debug Cons Can only scale-up Does not deal with large data sets Single Node solution for large scale exploratory analysis Specialized HW and SW for efficient Matrix operations Elements: Data engine software for optimized operations HW design pattern for balancing Storage, CPU and GPU computing Optimized machine learning package Advanced communication patterns Common Data Store Many Processors Parallel execution of tasks Processor communication R is a single thread computing application R Snow enable multi threading/distribution Pros Distributed/parallel Commonly known tool and model Cons Each node requires access to all data Connected processors collaborate to achieve a common goal Requires: Message passing Coordination Scheduling Tolerate failures on individual nodes Uniform nodes Data shards in a distributed storage Hadoop BigQuery Pregel Spark … Each node does computation Each node can be distributed Information is passed between nodes Execution is coordinated amongst nodes Distributed Nodes Heterogeneous and Physically Separate Nodes SETI@home (SETI Institute) Large Hadron Collider Computing Grid (CERN) NFCR Centre for Comp. Drug Discovery (Oxford Univ) Globus Toolkit (Globus Alliance) … Data Warehouse Data Lake Data Transformed to Many data sources defined schema Retain all data Loaded when usage Allows for exploration identified Apply transform as Allows for quick needed response of defined Apply schema as queries needed Key points: ● Extract needed data ● Map to schema ● Prepare for defined use cases Key points: Query ● Store all data Processing ● Transform as needed ● Apply schema as needed Cleaning, Transformation Schema (Avro, Thrift, Protobuf) Data Ingest Data Storage Understanding of data models and schemas in traditional BW Understanding of data models and schemas proposed for big data systems Transaction data and Fact based data model New transactions Analytics Data and facts Online Transaction Online Analytics Processing (OLTP) Processing (OLAP) systems and approach that systems and approach to facilitate and manage answering multi- transaction-oriented dimensional analytical applications, typically for queries quickly data entry and retrieval transaction processing OLTP OLAP CRUD Transactions (Create, Aggregations Read, Update, and Delete) Drill-downs, Frequent Updates Roll-ups Example query: Example query: UPDATE Employees select o.customerid, o.orderid, SET Salary='100,000' o.orderdate, p.price, sum WHERE EmployeeName='Alfred Nobel'; (p.price) over (partition by SELECT Salary FROM Employees o.customerid order by o.orderdate) WHERE Salary ='Alfred Nobel'; running_total Master Data Transaction Data Analytics Data Master Data: The source of truth in your system, cannot withstand corruption Transaction Data: Keeps relationships (e.g., who bought what) Analytics Data: Aggregated/processed transaction data Master Data (Dimension Tables) Transaction Data Analytics Data (Fact table) (Cuboid) Captures concepts and relationships that participate in transactions object, relationship, object attributes Each Record Captures a Transaction (id, object, amount, time, ...) Roll-ups, drill downs, summaries summary, average, grouping Master Data (fact based, Immutable, Dimensions) Transaction Data Analytics Data (Log items) (Aggregates, Roll-ups) In the fact-based model, you deconstruct the data into fundamental units called facts Supports queries about any time in history Enables complex queries (that a predefined schema might have have ignored) More easily correctable (remove erroneous facts) Two kinds of database management systems Relational Databases ▪ Presents via Declarative Query Languages ▪ Organize underlying storage row-wise ▪ Sometimes column-wise Columnar Databases ▪ Presents via API and Declarative Query Languages ▪ Organize underlying storage column-wise ● Records stored as tables ○ Schema validated on- write Logical Schema ○ Typically indexed <RowID> EmployeeID FirstName LastName Dept Salary ● Records may be persisted 001 12 John Smith Marketing 90 ○ Row-wise 002 24 Sue Richards Engine 130 ○ Column-wise ● Additional structures can 003 35 Maggie Smith DataSci 120 be applied to enhance 004 44 Bobby Jones DataSci 120 access ○ Secondary Indices ○ Materialized Views ○ Stored Procedures Physical Schema Row-Oriented 001:12,John,Smith,Marketing,90\n ● Very fast to insert rows 002:24,Sue,Richards,Engine,130\n ● Very fast to retrieve whole rows 003:35,Maggie,Smith,DataSci,120\n ● 004:44,Bobby,Jones,DataSci,120\n Slower to retrieve whole columns ● Records stored as tables ● Largely for analytical workloads Logical Schema ○ Read-mostly <RowID> EmployeeID FirstName LastName Dept Salary ○ Bulk-insertion 001 12 John Smith Marketing 90 ● Additional access structures ● Presumption 002 24 Sue Richards Engine 130 ○ More likely to read all 003 35 Maggie Smith DataSci 120 values of a column 004 44 Bobby Jones DataSci 120 than all values of a row ○ Optimize storage for fast column retrieval Physical Schema Column-Oriented 12:001;24:002;35:003;44:004\n ● Very fast to retrieve columns John:001;Sue:002;Maggie:003;Bobby:004\n ● May save space for sparse Smith:001,003;Richards:002;Jones:004\m data Marketing:001;Engine:002;DataSci:003,004\n ● Slow to insert, slow for row 90:001;130:002;120:003,004\n reads Relational Columnar Most common data storage Optimized for analytical and retrieval system workloads Many drivers, declarative Maintains relational data language (SQL) model Good for fast inserts Good for analytical Good for (some) fast reads operations Good for sharing data among Good for horizontal scaling applications Bad for fast writes Limited schema-on-read Bad for fast row-wise support reads Can be costly or difficult to scale Two distributed approaches to data storage HDFS (Hadoop Distributed File System) ▪ Presents like a local filesystem ▪ Distribution mechanics handled automatically NoSQL Databases (Key/Value Stores) ▪ Typically store records as “key-value pairs” ▪ Distribution mechanics tied to record keys File divided into blocks (Typically between 64MB and 256MB) ● Blocks replicated k times ○ Typically: k >= 3 ● Block placement tries to A Large File B1 B2 B3 ○ Ensure resiliency ○ Minimize network hops ● On read ○ Request 1 copy of each block Bn ○ Retries on other copies Server 1 Server 2 Server 3 ... Server n ● Records stored by key ○ Provides a simple index Key Value ● Record placement ○ Keys are used to shard 1234 Some text like this ○ All keys in a certain range go to a certain (set of) servers 2345 {name: “A JSON ● On read Document”, number: ○ Driver process reads from 7} servers based on key-ranges 3456 <image data> Server 1 Server 2 Server 3 ... Server n HDFS Key-Value Stores Popular bulk data store Many popular choices Many, large files ▪ Redis, Berkeley DB ▪ File size >= Block size ▪ MongoDB Agnostic to file content ▪ Cassandra (column-families Good as an immutable imposed on value) data store Good for fast, key-based Good for parallel reads of access lots of blocks Good for fast writes Bad for small, specific Bad for off-key access reads Complicated for merging Bad for fast writes datasets Two Concepts Object Storage (OS): as a new abstraction for storing data Software Defined Storage (SDS): An architecture that enables cost effective, scalable, highly available (HA) storage systems Combining OS and SDS provides an efficient solution for certain data applications Manage data as one (large) logical object Object Storage Consists of meta data and data Unique identifier across system Data is an uninterpreted set of bytes Software Defined Storage Distributed Storage Manager/Files System Network Node Node Node Data Replication for resilience and HA Fil Store anything Disc Disc Disce Disc Disc Disc Disc Fil Fil Fil Fil Fil Runs on commodity hardware e e e e e Manages data automatically, blocks hidden Simplified model for managing data growth Lowers management cost Lowers hardware cost Meet Resiliency and HA needs and lower cost Simple API with an Object Abstraction Data is partitioned, partitions are replicated for resiliency and HA Software layer ▪ manages replication and data placement ▪ automatically re-distributes
Details
-
File Typepdf
-
Upload Time-
-
Content LanguagesEnglish
-
Upload UserAnonymous/Not logged-in
-
File Pages84 Page
-
File Size-