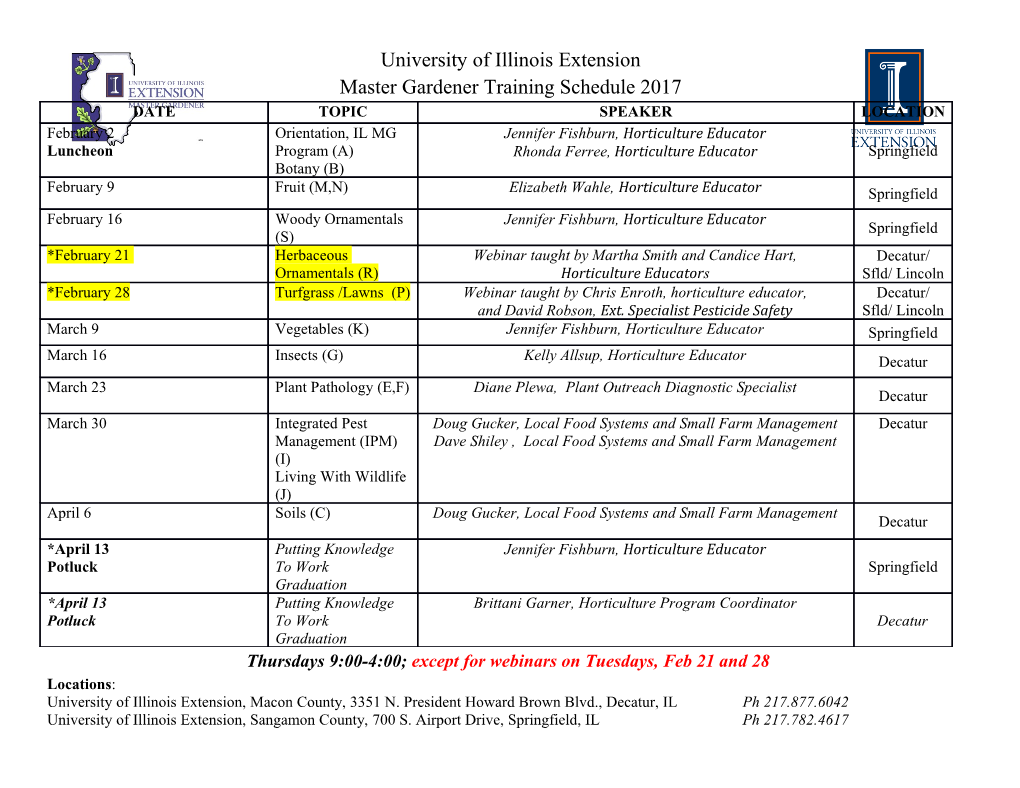
Data-driven discovery Case studies from patient records and spontaneous reports Niklas Norén, PhD Uppsala Monitoring Centre WHO Collaborating Centre for International Drug Monitoring ISPE 2011 Mid-Year Meeting. April 9, 2011. Florence, Italy. Disclosure • Uppsala Monitoring Centre research primarily self- funded • The results on patient records in this presentation came out of a, now finished, pilot study co-financed by IMS Health • Government support throught grants – IMI PROTECT – Monitoring Medicines (FP7) – OMOP (FNIH) Presentation outline • Data-driven discovery • Three case studies • Lessons learned RTWLAAQZDDYTFGFQXYSSSTFGLOIRQQXY DDAFAFEKRRQTNGDIRGEEWGWCFSSJWQYS JKAOFVHVVIKQXYWZSCYGRRWOYSAOVDVA DWUACVVSVKKIHLZJWDOVEYIQXYAQVVVQ KMRDPVWVFERUQTESQWMIERFPSYDVDAVQ JKKOLTHSNMKQXYYFNGHDDLYOCSAOLDZA VKKIHLZKRRQTNGDIRGEEWGWCFSSJWQXY QXYMYWZACIYRGEFQXYSWOYSAWLAAQZSE What is data-driven discovery? • The application of analytics to detect patterns in data • Let data lead the way! – No pre-specified hypothesis – Parallel perspectives on data – Many covariates, many pattern types • Simple diagnostic test: Can you enumerate the possible findings prior to your analysis? Why is it important? • Identify the unexpected • Obtain a more complete perspective of data • Highlight issues that may alter the interpretation of your primary analysis When is it relevant? • Fundamental to broad surveillance • A core component in signal refinement and refutation • Useful for data management and quality assurance • A safeguard in hypothesis-driven research Hand and Bolton J Appl Statist, 2004 Pattern discovery • A pattern can be defined as a local deviation from a global baseline model • Affects a limited number of covariates and / or data points RTWLAAQZDDYTFGFQXYSSSTFGLOIRQQXY DDAFAFEKRRQTNGDIRGEEWGWCFSSJWQYS JKAOFVHVVIKQXYWZSCYGRRWOYSAOVDVA DWUACVVSVKKIHLZJWDOVEYIQXYAQVVVQ KMRDPVWVFERUQTESQWMIERFPSYDVDAVQ JKKOLTHSNMKQXYYFNGHDDLYOCSAOLDZA VKKIHLZKRRQTNGDIRGEEWGWCFSSJWQXY QXYMYWZACIYRGEFQXYSWOYSAWLAAQZSE Hand and Bolton J Appl Statist, 2004 Pattern discovery • A pattern can be defined as a local deviation from a global baseline model • Affects a limited number of covariates and / or data points RTWLAAQZDDYTFGFQXYSSSTFGLOIRQQXY DDAFAFEKRRQTNGDIRGEEWGWCFSSJWQYS JKAOFVHVVIKQXYWZSCYGRRWOYSAOVDVAJKAOF V H VV IKQXYWZSCYGRRWOYSAO V D V A DWUACDWUACVVSVKKIHLZJWDOVEYIQXYAQVVVQVV S V KKIHLZJWDO V EYIQXYAQ VVV Q KMRDPKMRDPVWVFERUQTESQWMIERFPSYDVDAVQV W V FERUQTESQWMIERFPSYD V DA V Q JKKOLTHSNMKQXYYFNGHDDLYOCSAOLDZA VKKIHLZKRRQTNGDIRGEEWGWCFSSJWQXY QXYMYWZACIYRGEFQXYSWOYSAWLAAQZSE How do you do it? • No such thing as completely open-ended analysis! • Need to define: – Type of patterns (examples on following slides) – Baseline model – Covariates – Data subset(s) – How to follow up • The challenge is to maintain power to detect the unexpected Success factors • Data preparation – Effective data management and cleaning • Robustness to data quality issues – ... or relevant patterns may be drowned in noise • Control of false alerts – Some false positives ok but positive predictive value must be acceptable – Rate of spurious associations can often be evaluated with Monte Carlo simulation or permutation tests – Biases are more difficult! Norén et al. Data Min Knowl Discov, 2007 Record matching • Duplicate detection in six million VigiBase reports • Screen for pairs of suspiciously similar records • Baseline model: independent reports • Score each covariate with log-likelihood ratio (matches rewarded, mismatches penalized) RTWLAAQZDDYTFGFQXYSSSTFGLOIRQQXY DDAFAFEKRRQTNGDIRGEEWGWCFSSJWQYS JKAOFVHVVIKQXYWZSCYGRRWOYSAOVDVA DWUACVVSVKKIHLZJWDOVEYIQXYAQVVVQ KMRDPVWVFERUQTESQWMIERFPSYDVDAVQ JKKOLTHSNMKQXYYFNGHDDLYOCSAOLDZA VKKIHLZKRRQTNGDIRGEEWGWCFSSJWQXY QXYMYWZACIYRGEFQXYSWOYSAWLAAQZSE Norén et al. Data Min Knowl Discov, 2007 Record matching • Duplicate detection in six million VigiBase reports • Screen for pairs of suspiciously similar records • Baseline model: independent reports • Score each covariate with log-likelihood ratio (matches rewarded, mismatches penalized) RTWLAAQZDDYTFGFQXYSSSTFGLOIRQQXY DDAFAFE KRRQTNGDIRGEEWGWCFSSJWQ YS JKAOFVHVVIKQXYWZSCYGRRWOYSAOVDVA DWUACVVSVKKIHLZJWDOVEYIQXYAQVVVQ KMRDPVWVFERUQTESQWMIERFPSYDVDAVQ JKKOLTHSNMKQXYYFNGHDDLYOCSAOLDZA VKKIHLZ KRRQTNGDIRGEEWGWCFSSJWQ XY QXYMYWZACIYRGEFQXYSWOYSAWLAAQZSE Norén et al. Data Min Knowl Discov, 2007 Record matching method • Covariates: country of origin, patient gender, patient age, date of onset, outcome, drugs, suspected ADRs • Suspected duplicates reviewed by national centre RTWLAAQZDDYTFGFQXYSSSTFGLOIRQQXY DDAFAFE KRRQTNGDIRGEEWGWCFSSJWQ YS JKAOFVHVVIKQXYWZSCYGRRWOYSAOVDVA DWUACVVSVKKIHLZJWDOVEYIQXYAQVVVQ KMRDPVWVFERUQTESQWMIERFPSYDVDAVQ JKKOLTHSNMKQXYYFNGHDDLYOCSAOLDZA VKKIHLZ KRRQTNGDIRGEEWGWCFSSJWQ XY QXYMYWZACIYRGEFQXYSWOYSAWLAAQZSE Norén et al. Data Min Knowl Discov, 2007 Record matching results • 78,000 suspected duplicates (2011) • ~65% recall, ~80% precision (rel. manual review, small study!) • Highlighted non-duplicates typically otherwise related Country Patient Patient Drugs ADRs Date of of origin age gender onset Norway 8 F Epinephrine/Lidocaine Facial pain 2003-12-16 Norway 18 F Epinephrine/Lidocaine Facial pain 2003-12-16 Norway 29 F Epinephrine/Lidocaine Facial pain 2003-12-16 • Three reports from the same dentist! Norén et al. Stat Med, 2008. Interaction detection • Drug interaction detection in VigiBase • Covariates: drugs and ADRs • Identify excess reporting of ADR with two drugs RTWLAAQZDDYTFGFQXYSSSTFGLOIRQQXY DDAFAFEKRRQTNGDIRGEEWGWCFSSJWQYS JKAOFVHVVIKQXYWZSCYGRRWOYSAOVDVA DWUACVVSVKKIHLZJWDOVEYIQXYAQVVVQ KMRDPVWVFERUQTESQWMIERFPSYDVDAVQ JKKOLTHSNMKQXYYFNGHDDLYOCSAOLDZA VKKIHLZKRRQTNGDIRGEEWGWCFSSJWQXY QXYMYWZACIYRGEFQXYSWOYSAWLAAQZSE Norén et al. Stat Med, 2008. Interaction detection • Drug interaction detection in VigiBase • Covariates: drugs and ADRs • Identify excess reporting of ADR with two drugs RTWLAAQZDDYTFGF QX Y SSSTFGLOIRQ QX Y DDAFAFEKRRQTNGDIRGEEWGWCFSSJWQYS JKAOFVHVVIK QX Y WZSCYGRRWOYSAOVDVA DWUACVVSVKKIHLZJWDOVEYI QX Y AQVVVQ KMRDPVWVFERUQXESQWMIERFPSYDVDAVQ JKKOLTHSNMK QX Y YFNGHDDLYOCSAOLDZA VKKIHLZKRRQTNGDIRGEEWGWCFSSJW QX Y QX Y MYWZACIYRGEF QX Y SWOYSAWLAAQZSE Norén et al. Stat Med, 2008. Interaction detection method • Baseline model: additive attributable risks • Shrinkage observed-to-expected ratio to protect against spurious associations • Suspected interactions assessed by clinical experts RTWLAAQZDDYTFGF QX Y SSSTFGLOIRQ QX Y DDAFAFEKRRQTNGDIRGEEWGWCFSSJWQYS JKAOFVHVVIK QX Y WZSCYGRRWOYSAOVDVA DWUACVVSVKKIHLZJWDOVEYI QX Y AQVVVQ KMRDPVWVFERUQXESQWMIERFPSYDVDAVQ JKKOLTHSNMK QX Y YFNGHDDLYOCSAOLDZA VKKIHLZKRRQTNGDIRGEEWGWCFSSJW QX Y QX Y MYWZACIYRGEF QX Y SWOYSAWLAAQZSE Norén et al. Stat Med, 2008. Interaction detection results • 15,000 triplets with excess reporting rates • Among those are cases of known interactions such as cerivastatin/gemfibrozil, digoxin/clarithromycin etc. • Also report clusters and a patient safety issue: Drugs ADR(s) # Reports Expected Comment Bupivacain Strabismus 25 <1 25 reports listing the same Hyaluronidase five drugs and ADR Cefazolin submitted by the same Gentamicin reporter in 1985 Lidocaine Celecoxib Drug maladministration 51 <1 Confusion of brand names Citalopram (Celebrex & Celexa) Norén et al. Data Min Knowl Discov, 2010 Temporal pattern discovery • Pattern discovery in IMS UK collection of two million longitudinal patient records • Covariates: drugs and medical events • Screen for medical events that occur more often than expected soon after start of treatment Norén et al. Data Min Knowl Discov, 2010 Temporal pattern discovery method • Baseline model: Relative frequency of medical event constant over time in exposed patients • Self-controlled cohort with external control group to adjust for age gradients, variations in use of healthcare and clustering of doctor’s visits • Follow-up: – Visualisation of temporal patterns – Computerized highlighting of potential confounders – Clinical assessment of patient details – Secondary analysis (related drugs or events, stratification...) Norén et al. Data Min Knowl Discov, 2010 Temporal pattern discovery results • 42,000 associations between drugs and events • A variety of temporal patterns Lessons learned • Many different patterns can be highlighted as deviations from a single simple baseline model • A substantial proportion of findings relate to data quality issues or highlight aspects of data that are important for interpretation of the primary analysis • Major intellectual input required after initial discovery! More lessons learned • 10,000+ patterns -> additional triages required – Emerging patterns – Focus areas – Predictive models • Careful communication! • Many times, biases dominate! • Multiple comparisons are a real issue in some applications Hopstadius and Norén Submitted, 2011 – Naive sub-group analyses in spontaneous reports can lead to ~50% false positive rates RTWLAAQZD D YTFGF QXY SSSTFGLOIRQ QXY D D AFAFE K R RQTNG DI RGEEWGWCFSSJWQ YS JKAOF V H VV I K QXY WZ SC YGRRWOYSAO V D V A DWU A C VV S V KKIHLZJWD OV EYI QXY AQ VVV Q KMRDP V W V F E RUQTESQWMI ER FPSYD V DA V Q JKKOL T HS N MK QXY YFNGHDDL Y OCSAOLDZA VKKIHLZ KRRQTNGDIRGEEWGWCFSSJWQXY QXY MYWZ A CIYRGEF QXY SWOYSAWLAAQZSE References 1. Hand DJ, Bolton R. Pattern discovery and detection: a unified statistical methodology . Journal of Applied Statistics , 2004. 31 (8):885-924. 2. Norén GN, Orre R, Bate A, Edwards IR. Duplicate detection in adverse drug reaction surveillance . Data Mining and
Details
-
File Typepdf
-
Upload Time-
-
Content LanguagesEnglish
-
Upload UserAnonymous/Not logged-in
-
File Pages26 Page
-
File Size-