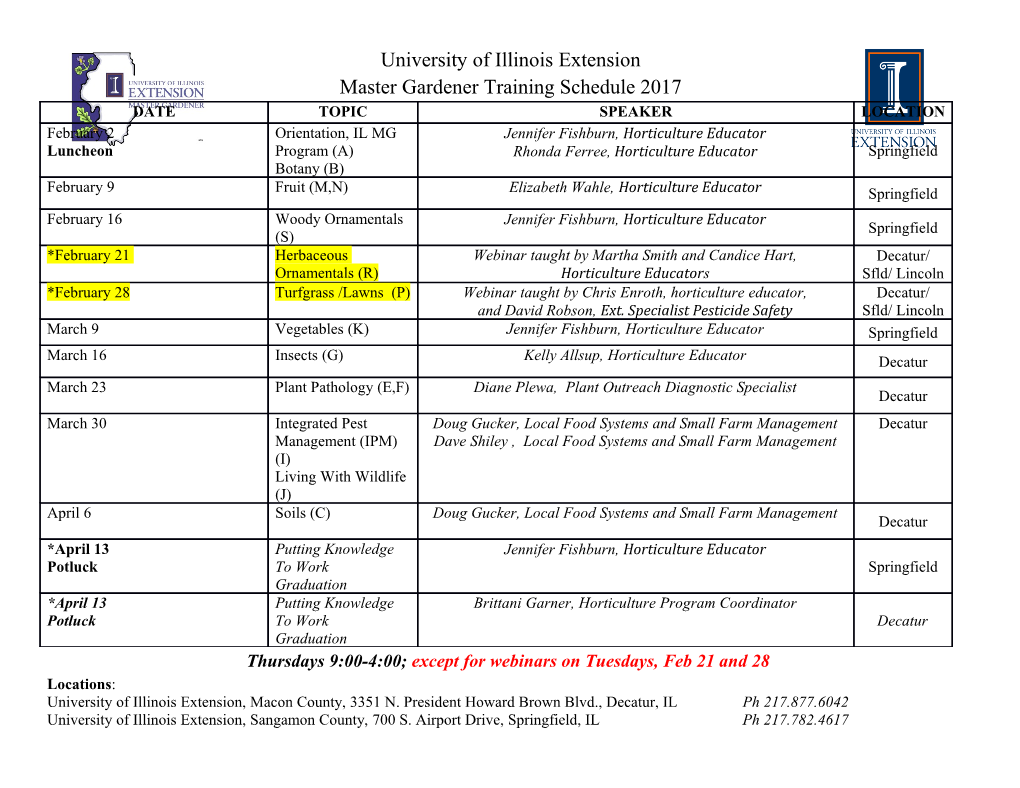
koan¯ : A Corrected CBOW Implementation Ozan Irsoy˙ Adrian Benton Bloomberg LP Bloomberg LP [email protected] [email protected] Karl Stratos Rutgers University [email protected] Abstract It is a common belief in the NLP community that continuous bag-of-words (CBOW) word embeddings tend to underperform skip-gram (SG) embeddings. We find that this belief is founded less on theoretical differences in their training objectives but more on faulty CBOW implementations in standard software li- braries such as the official implementation word2vec.c [Mikolov et al., 2013b] and Gensim [Rehˇ u˚rekˇ and Sojka, 2010]. We show that our correct implementa- tion of CBOW yields word embeddings that are fully competitive with SG on various intrinsic and extrinsic tasks while being more than three times as fast to train. We release our implementation, koan¯ 1, at https://github.com/ bloomberg/koan. 1 Introduction Pre-trained word embeddings are a standard way to boost performance on many tasks of interest such as named-entity recognition (NER), where contextual word embeddings such as BERT [Devlin et al., 2019] are computationally expensive and may only yield marginal gains. Word2vec [Mikolov et al., 2013a] is a popular method for learning word embeddings because of its scalability and robust performance. Word2vec offers two training objectives: (1) continuous bag-of-words (CBOW) that predicts the center word by averaging context embeddings, and (2) skip-gram (SG) that predicts context words from the center word.2 A common belief in the NLP community is that while CBOW is fast to train, it lags behind SG in performance.3 This observation is made by the inventors of Word2vec themselves [Mikolov, 2013] and also independently by other works such as Stratos et al. [2015]. This is strange since the two arXiv:2012.15332v1 [cs.CL] 30 Dec 2020 objectives lead to very similar weight updates. This is also strange given the enormous success of contextual word embeddings based on masked language modeling (MLM), as CBOW follows a rudimentary form of MLM (i.e., predicting a masked target word from a context window without any perturbation of the masked word). In fact, direct extensions of CBOW such as fastText [Bojanowski et al., 2017] and Sent2vec [Pagliardini et al., 2018] have been quite successful. In this work, we find that the performance discrepancy between CBOW and SG embeddings is founded less on theoretical differences in their training objectives but more on faulty CBOW im- plementations in standard software libraries such as the official implementation word2vec.c [Mikolov et al., 2013b] and Gensim [Rehˇ u˚rekˇ and Sojka, 2010]. Specifically, we find that in these 1A story which is used in Zen practice to provoke “great doubt”. 2In this work, we always use the negative sampling formulations of Word2vec objectives which are consis- tently more efficient and effective than the hierarchical softmax formulations. 3SG requires sampling negative examples from every word in context, while CBOW requires sampling negative examples only for the target word. implementations, the gradient for source embeddings is incorrectly multiplied by the context win- dow size, resulting in incorrect weight updates and inferior performance. We make the following contributions. First, we show that our correct implementation of CBOW indeed yields word embeddings that are fully competitive with SG while being trained in less than a third of the time (e.g., it takes less than 73 minutes to train strong CBOW embeddings on the entire Wikipedia corpus). We present experiments on intrinsic word similarity and anal- ogy tasks, as well as extrinsic evaluations on the SST-2, QNLI, and MNLI tasks from the GLUE benchmark [Wang et al., 2018], and the CoNLL03 English named entity recognition task [Sang and De Meulder, 2003]. Second, we make our implementation, koan¯ , publicly available at https://github.com/bloomberg/koan. In addition to including a correct CBOW im- plementation, koan¯ features other software improvements such as a constant time, linear space negative sampling routine based on the alias method [Walker, 1974] along with multi-threaded sup- port. 2 Implementation The parameters of CBOW are two sets of word embeddings: “source-side” and “target-side” vectors 0 d vw; vw 2 R for every word type w 2 V in the vocabulary. A window of text in a corpus consists of a center word wO and context words w1 : : : wC . For instance, in the window the dog laughed, we have wO = dog and w1 = the and w2 = laughed. Given a window of text, the CBOW loss is defined as: C 1 X v = v c C wj j=1 k > X > L = − log σ(v0 v ) − log σ(−v0 v ) wO c ni c i=1 where n1 : : : nk 2 V are negative examples drawn iid from some noise distribution Pn over V . The L v0 v0 v gradients of with respect to the target ( wO ), negative target ( ni ), and average context source ( c) embeddings are: @L 0 > =(σ(v vc) − 1)vc @v0 wO wO @L 0 > =σ(v vc)vc @v0 ni ni k @L 0 > 0 X 0 > 0 =(σ(v vc) − 1)v + σ(v vc)v (1) @v wO wO ni ni c i=1 and by the chain rule with respect to a source context embedding: k @L 1 0 > 0 X 0 > 0 = [(σ(v vc) − 1)v + σ(v vc)v ] @v C wO wO ni ni wj i=1 However, the CBOW negative sampling implementations in word2vec.c4 and Gensim5 incor- rectly update each context vector by Eq. (1), without normalizing by the number of context words. In fact, this error has been pointed out in several Gensim issues.6 Why does this matter? Aside from being incorrect, this update matters because: 4https://github.com/tmikolov/word2vec/blob/20c129af10659f7c50e86e3be406df663beff438/ word2vec.c#L483 5In fact, it appears that normalization by number of context words is only done for the “sum variant” of CBOW: https://github.com/RaRe-Technologies/gensim/blob/master/ gensim/models/word2vec_inner.pyx#L455-L456. 6https://github.com/RaRe-Technologies/gensim/issues/1873, https: //github.com/RaRe-Technologies/gensim/issues/697. 2 CBOW, 5 epochs Skipgram, 1 epoch 10 10 Implementation Implementation word2vec.c word2vec.c 8 gensim 8 gensim k an k an 6 6 4 4 Time (hours) Time (hours) 2 2 0 0 4 8 16 32 48 4 8 16 32 48 Workers Workers Figure 1: Hours to train CBOW (left) and SG (right) embeddings as a function of number of worker threads for different Word2vec implementations. 1. In both Gensim and word2vec.c, the width of the context window is randomly sampled from 1;:::;Cmax for every target word. This means that context embeddings which were averaged over wider context windows will experience a larger update than their contribu- tion, relative to context embeddings averaged over narrower windows. As a consequence, the norm of the source embeddings increases with the context size (Appendix B). 2. One might think that scaling of the source vector updates will have little effect on the re- sulting embeddings, as this stochastic scaling can be folded into the learning rate. However, recall that the set of parameters for the CBOW model is θ = [v; v0]. Now it is clear that by scaling the update for source and not target embeddings, we are no longer taking a step in the direction of the negative stochastic gradient, and our computation of the stochastic gradient is no longer an unbiased estimate of the true gradient. See Appendix C for an ana- lysis of how the incorrect gradient update relates to the correct one as a function of CBOW hyperparameters. In addition to implementing the correct gradient for CBOW training, we also use the alias method for constant time, linear memory negative sampling (Appendix D). 3 Experiments We evaluated CBOW and SG embeddings as well as recorded the time it took to train them under Gensim and our implementations. Unless otherwise noted, we learn word embeddings on the entire Wikipedia corpus. The corpus was tokenized using the PTB tokenizer with default settings, and words occurring fewer than ten times were dropped. Training hyperparameters were held fixed for each set of embeddings: negative sampling rate 5, maximum context window of 5 words, number of training epochs 5, and embedding dimensionality 300. We found that the default initial learning rate in Gensim, 0.025, learned strong SG embeddings. However, we swept separately for CBOW initial learning rate for Gensim and our implementa- tion. We swept over initial learning rate in f0:025; 0:05; 0:075; 0:1g selecting 0.025 to learn Gensim CBOW embeddings and 0.075 for koan¯ . We selected learning rate to maximize average perfor- mance on the intrinsic evaluation tasks. We believe that Gensim requires a smaller learning rate for CBOW, because a smaller learning rate is more likely to reduce the stochastic loss, since the incorrect update is still a descent direction, although not following the negative stochastic gradient (Appendix C). 3.1 Training time Figure 1 shows the time to train each set of embeddings as a function of number of worker threads on the tokenized Wikipedia corpus. All implementations were benchmarked on an Amazon EC2 c5.metal instance (96 logical processors, 196GB memory) using time, and embeddings were trained with a minimum token frequency of 10 and downsampling frequency threshold of 10−3. We benchmark koan¯ with a buffer of 100,000 sentences. We trained CBOW for five epochs and SG for a single epoch, as it is much slower. Gensim seems to have trouble exploiting more worker 3 threads when training CBOW, and both word2vec.c and koan¯ learn embeddings faster.
Details
-
File Typepdf
-
Upload Time-
-
Content LanguagesEnglish
-
Upload UserAnonymous/Not logged-in
-
File Pages10 Page
-
File Size-