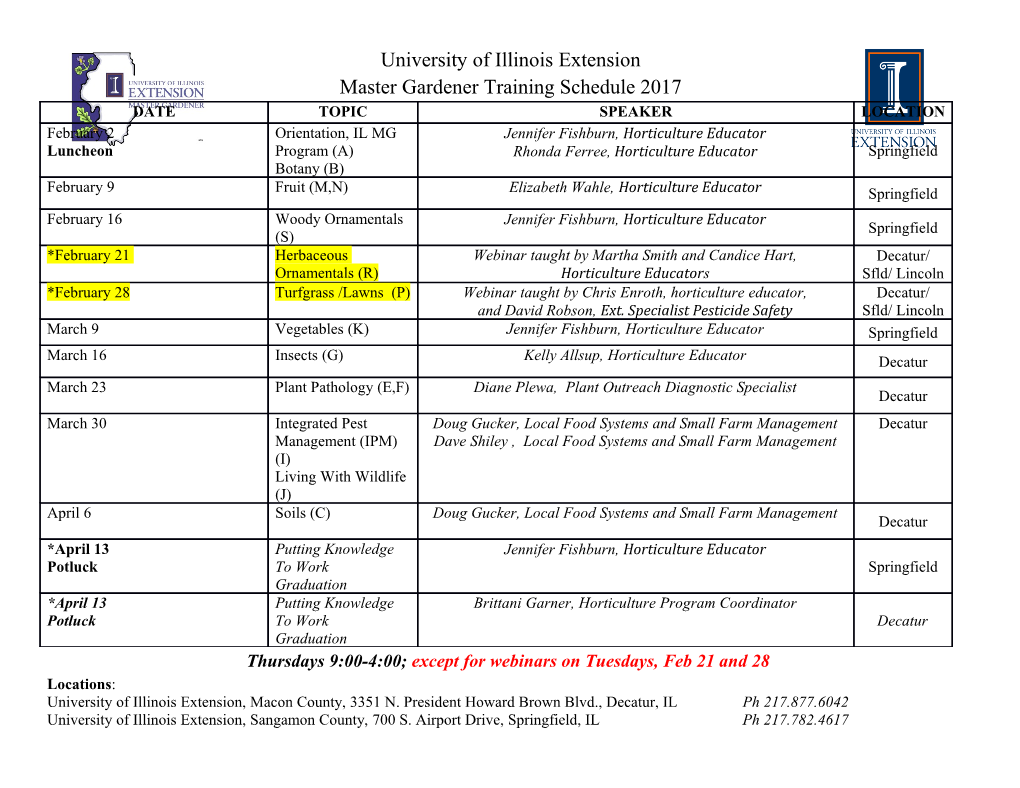
ORIGINAL ARTICLE Robustness and Reproducibility of Radiomics in Magnetic Resonance Imaging A Phantom Study Bettina Baeßler, MD,*† Kilian Weiss, PhD,‡ and Daniel Pinto dos Santos, MD* finally aims at supporting clinical decision making and leads the Objectives: The aim of this study was to investigate the robustness and reproduc- way toward precision medicine, while overcoming the limitations ibility of radiomic features in different magnetic resonance imaging sequences. of a purely visual image interpretation. Materials and Methods: A phantom was scanned on a clinical 3 T system using The extensive and encouraging preliminary clinical research in fluid-attenuated inversion recovery (FLAIR), T1-weighted (T1w), and T2-weighted the field has led to an increasing desire and need for translating (T2w) sequences with low and high matrix size. For retest data, scans were repeated radiomic image analysis to clinical practice. The establishment of novel after repositioning of the phantom. Test and retest datasets were segmented using quantitative imaging biomarkers, however, has to be preceded by an a semiautomated approach. Intraobserver and interobserver comparison was per- extensive knowledge of the robustness and reproducibility of the un- formed. Radiomic features were extracted after standardized preprocessing of derlying quantitative imaging features. Recently, an Image biomarker images. Test-retest robustness was assessed using concordance correlation coef- standardization initiative has been formed by Zwanenburg and col- ficients, dynamic range, and Bland-Altman analyses. Reproducibility was assessed leagues,7 addressing the need for standardization of the radiomic by intraclass correlation coefficients. feature extraction process. In addition, many studies have been Results: The number of robust features (concordance correlation coefficient and ≥ published mostly in the environment of CT and positron emission dynamic range 0.90) was higher for features calculated from FLAIR than from tomography imaging highlighting the challenges of reproducibility of T1w and T2w images. High-resolution FLAIR images provided the highest per- radiomic features when using different vendors, scanners or acquisi- centage of robust features (n = 37/45, 81%). No considerable difference in the tion, and reconstruction settings.7–15 number of robust features was observed between low- and high-resolution T1w Conversely, only very few studies have analyzed the robustness and T2w images (T1w low: n = 26/45, 56%; T1w high: n = 25/45, 54%; T2 low: of radiomic features in MRI.16–19 Given the qualitative nature of most n = 21/45, 46%; T2 high: n = 24/45, 52%). A total of 15 (33%) of 45 features showed MRI techniques and the known variations of the resulting absolute excellent robustness across all sequences and demonstrated excellent intraobserver 20 ≥ signal intensities, we hypothesized that the robustness of radiomic and interobserver reproducibility (intraclass correlation coefficient 0.75). features extracted from MRI scans largely depends on the MRI se- Conclusions: FLAIR delivers the most robust substrate for radiomic analyses. quence used for image acquisition as well as on acquisition and Only 15 of 45 features showed excellent robustness and reproducibility across reconstruction settings. all sequences. Care must be taken in the interpretation of clinical studies using Thus, the presented phantom study aims at evaluating the ro- nonrobust features. bustness and reproducibility of radiomic imaging features for the most Key Words: radiomics, texture analysis, magnetic resonance imaging, MRI, commonly used MRI sequences in a phantom at 3 Tand proposing a set robustness, reproducibility, phantom study of robust features, which can be reliably used in future clinical studies. (Invest Radiol 2019;54: 221–228) MATERIALS AND METHODS he recent rise of machine learning techniques and the exponential T growth of computational power enable researchers to exploit large Study Design numbers of quantitative features derived from medical images,1 a field A total of 4 onions, 4 limes, 4 kiwifruits, and 4 apples placed on called radiomics. The term radiomics refers to the characterization of a a box made out of styrofoam served as our radiomics phantom (Fig. 1). tissue (eg, a tumor2–4 or the myocardium5,6) by extraction of high- The different vegetables/fruits were supposed to reflect different signal dimensional, mineable data from various sources of medical images, intensities, shapes, and tissue textures. Image acquisition was repeated including computed tomography (CT) and magnetic resonance im- immediately after repositioning of the phantom and replanning of all aging (MRI). Various classes of radiomic features can be extracted, sequences to obtain test and retest data. including morphological (shape), intensity-based (histogram), and various textural features. The subsequent analysis of these features Magnetic Resonance Imaging The phantom was placed in a clinical 3 T scanner (Ingenia; Philips Healthcare, Best, the Netherlands) and imaged using the stan- Received for publication August 16, 2018; and accepted for publication, after revision, dard body-matrix coil and built-in spine matrix coil. Six different MRI October 6, 2018. From the *Department of Radiology, University Hospital of Cologne, Cologne; †Institute sequences were acquired (Fig. 1): (1) low-resolution fluid-attenuated in- of Clinical Radiology and Nuclear Medicine, University Medical Centre Mannheim, version recovery (FLAIR), (2) high-resolution FLAIR, (3) low-resolution Medical Faculty Mannheim, Heidelberg University, Mannheim; and ‡Philips T1-weighted (T1w), (4) high-resolution T1w (T1w), (5) low-resolution Healthcare Hamburg, Hamburg, Germany. T2-weighted (T2w), and (6) high-resolution T2w. Imaging parameters Conflicts of interest and sources of funding: none decalred. Supplemental digital contents are available for this article. Direct URL citations appear are shown in Table 1. The resolution was changed by alteration of the in the printed text and are provided in the HTML and PDF versions of this article matrix size while keeping all other imaging parameters constant. The on the journal’s Web site (www.investigativeradiology.com). high-resolution sequences were adopted from the standard clinical Correspondence to: Bettina Baeßler, MD, Institute of Clinical Radiology and Nu- brain imaging sequences used in our department. clear Medicine, University Medical Centre Mannheim, Theodor-Kutzer-Ufer 1-3, D-68167 Mannheim, Germany. E-mail: [email protected]. Copyright © 2018 Wolters Kluwer Health, Inc. All rights reserved. Image Segmentation ISSN: 0020-9996/19/5404–0221 Image segmentation was performed semiautomatically using the DOI: 10.1097/RLI.0000000000000530 3-dimensional (3D) Slicer open-source software platform (version 4.8; Investigative Radiology • Volume 54, Number 4, April 2019 www.investigativeradiology.com 221 Copyright © 2019 Wolters Kluwer Health, Inc. All rights reserved. Baeßler et al Investigative Radiology • Volume 54, Number 4, April 2019 FIGURE 1. Phantom images acquired with different MRI sequences. Images of the vegetable/fruit radiomics phantom (upper left), an exemplarily 2-dimensional slice after segmentation with colored segmentation label maps (upper mid), and the 3D segmentation label (upper right). In the middle row from left to right, exemplarily images of the phantom acquired with FLAIR, T1w, and T2 low-resolution imaging; in the bottom row, images acquired with FLAIR, T1w, and T2w high-resolution imaging. T1w indicates T1-weighted; T2w, T2-weighted. www.slicer.org21) as follows (Fig. 1): (1) after loading the Digital Imag- 3D volumes of interest (VOIs) from the surrounding volume; (4) the ing and Communications in Medicine (DICOM) files, a region of interest semiautomatically generated VOIs were corrected manually to exclude, (ROI) was placed separately in each vegetable/fruit using the Segment for example, partial volume artifacts by manually excluding the border Editor; (2) an additional ROI was placed in the volume outside the zone between fruit/vegetable and surrounding air as well as the most vegetables/fruits; (3) the grow from seeds algorithm was used to seg- apical and basal slice of each fruit/vegetable using a brush-erase tool; ment all 16 vegetables/fruits semiautomatically and to separate their and (5) the corresponding label map was exported and saved as a .nii 222 www.investigativeradiology.com © 2018 Wolters Kluwer Health, Inc. All rights reserved. Copyright © 2019 Wolters Kluwer Health, Inc. All rights reserved. Investigative Radiology • Volume 54, Number 4, April 2019 Robustness of Radiomics in MRI TABLE 1. Imaging Sequence Parameters FLAIR Low FLAIR High T1 Low T1 High T2 Low T2 High Parameter Resolution Resolution Resolution Resolution Resolution Resolution Voxel size (acquired), mm 1.2 Â 1.5 Â 5.5 0.8 Â 1.1 Â 5.5 1.4 Â 1.4 Â 5.5 0.9 Â 0.9 Â 5.5 0.8 Â 1.0 Â 5.5 0.56 Â 0.70 Â 5.5 Voxel size (reconstructed), mm 0.45 Â 0.45 Â 5.5 0.45 Â 0.45 Â 5.5 0.45 Â 0.45 Â 5.5 0.45 Â 0.45 Â 5.5 0.30 Â 0.30 Â 5.5 0.30 Â 0.30 Â 5.5 Field of view 300 Â 300 Â 77 300 Â 300 Â 77 300 Â 300 Â 77 300 Â 300 Â 77 300 Â 300 Â 77 300 Â 300 Â 77 TR, ms 12000 1200 366 366 2500 2500 TE, ms 140 140 13.4 13.4 80 80 Flip angle, degrees 90 90 90 90 90 90 FLAIR indicates fluid-attenuated inversion recovery. file. Image segmentation with manual correction took approximately the lack of a fully automated segmentation approach), calculated CCCs 10 minutes per image stack. After segmentation of the test and retest were corrected for intraobserver variability as follows: CCCcorr =CCC+ dataset, one observer repeated the segmentation of all images in the test (1 − intraobserver ICC). dataset after a pause of 2 weeks and in random order to allow for Statistical analysis was performed in R (version 3.4.0; R Founda- intraobserver comparison. A second observer analyzed all images of tion for Statistical Computing, Vienna, Austria26) with RStudio (version the test dataset to allow for assessment of interobserver reproducibility.
Details
-
File Typepdf
-
Upload Time-
-
Content LanguagesEnglish
-
Upload UserAnonymous/Not logged-in
-
File Pages8 Page
-
File Size-