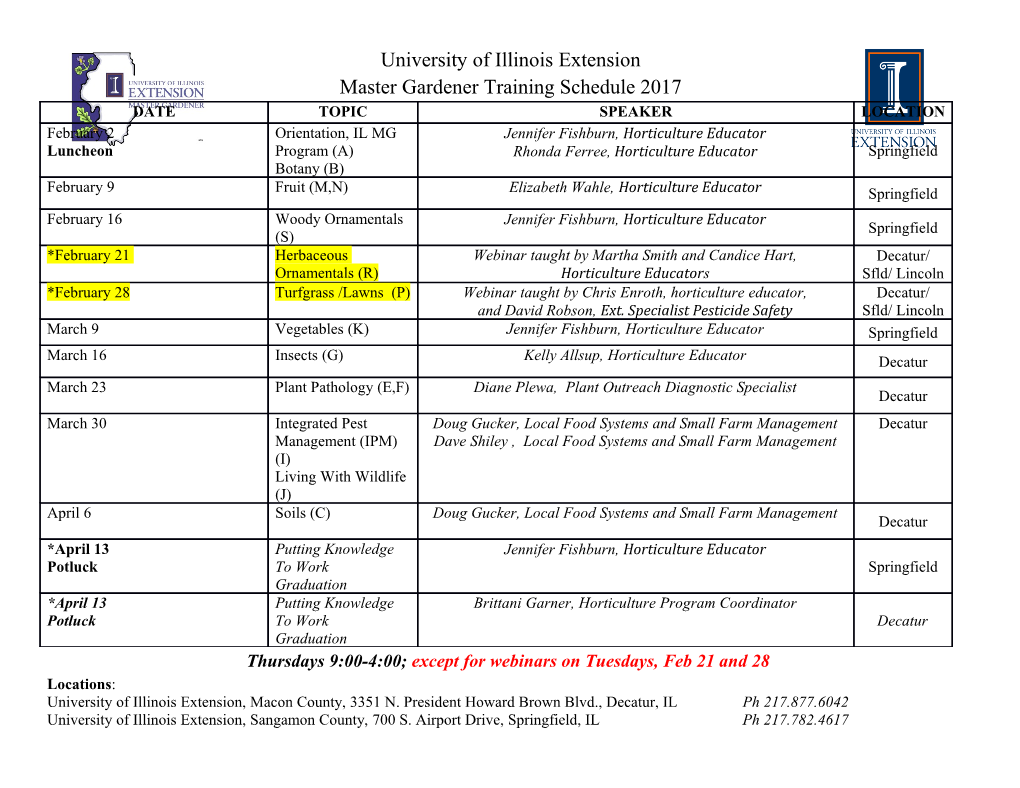
Cheat Sheet: Mathematical Foundations for Finance Steven Battilana, Autumn Semester 2017 1 1 b b 2 b 2 Thm. (dominated convergence theorem for stochastic integrals) • R u(τ)w(τ)dτ ≤ R u2(τ)dτ R w2(τ)dτ a a a Suppose that X is a semimartingale with decomposition X = M +A 1 1 n • ja(u; v)j ≤ (a(u; v)) 2 (a(u; v)) 2 as above, and let G ; n 2 N, and G be predictable processes. If Def. (Taylor) n j j Gt (!) ! Gt(!) for anyt ≥ 0, almost surely, • f(x ± h) = P1 (±h) d f 1 Appendix: Some Basic Concepts and Results j=0 j! dxj and if there exists a process H that is integrable w.r.t. X such that (n) P1 f (a) n jGnj ≤ H for any n 2 N, then • T f(x; a) = n=0 n! (x − a) Rem. (uniform convergence on compacts in probability 1) GnX ! G X u.c.p., as n ! 1. 1.1 Very Basic Things A mode of convergence on the space of processes which occurs often · · 2 in the study of stochastic calculus, is that of uniform convergence on If, in addition to the assumptions above, X is in H and kHkX < 1 then even Def. compacts in probability or ucp convergence for short. First, a sequence of (non-random) functions fn : R+ ! R converges n Q0 kG· X − G·XkH2 ! 0 as n ! 1. (i) An empty product equals 1, i.e. j=1 sj = 1. uniformly on compacts to a limit f if it converges uniformly on each P0 bounded interval [0; t]. That is, "Def." (Wiki: null set) (ii) An empty sum equals 0, i.e. j=1 sj = 0. In set theory, a null set N ⊂ R is a set that can be covered by a Def. (geometric series) sup jfn(s) − f(s)j ! 0 s≤t countable union of intervals of arbitrarily small total length. The no- n n+1 n+1 tion of null set in set theory anticipates the development of Lebesgue P k q6=1 q −1 1−q as n ! 1. (i) sn = a0 q = a0 = a0 measure since a null set necessarily has measure zero. More generally, q−1 1−q If stochastic processes are used rather than deterministic functions, k=0 on a given measure space M = (X; Σ; µ) a null set is a set S ⊂ X then convergence in probability can be used to arrive at the following 1 jqj<1 s.t. µ(S) = 0. (ii) s = P a qk = a0 definition. 0 q−1 Def. (power set) k=0 Def. (uniform convergence on compacts in probability) We denote by 2Ω the power set of Ω; this is the family of all subsets A sequence of jointly measurable stochastic processes Xn converges Def. (conditional probabilities) of Ω. to the limit X uniformly on compacts in probability if Q[C \ D] = Q[C]Q[DjC] Def. (σ-fiel or σ-algebra) " # A σ-field or σ-algebra on Ω is a family F of subsets of Ω which con- Def. (compact) n P sup jXs − Xsj > K ! 0 tains Ω and which is closed under taking complements and countable Compactness is a property that generalises the notion of a subset of s≤t unions, i.e. Euclidean space being closed (that is, containing all its limit points) c and bounded (that is, having all its points lie within some fixed di- as n ! 1; 8t; K > 0. A 2 F ) A 2 F N S stance of each other). Examples include a closed interval, a rectangle, Rem. (uniform convergence on compacts in probability 2) Ai 2 F; i 2 ) i2N Ai is in F ucp or a finite set of points (e.g. [0; 1]). The notation Xn −−! X is sometimes used, and Xn is said to Rem. Def. (P -trivial) converge ucp to X. Note that this definition does not make sense for F is then also closed under countable intersections. F0 is P -trivial iff P [A] 2 f0; 1g; 8A 2 F0. arbitrary stochastic processes, as the supremum is over the uncoun- Def. (finite σ-field) Useful rules table index set [0; t] and need not be measurable. However, for right A σ-field is called finite if it contains only finitely many sets. 1 2 Z µ+ 2 σ 2 or left continuous processes, the supremum can be restricted to the Def. (measurable space) E[e ] = e , for Z ∼ N (µ, σ ). countable set of rational times, which will be measurable. A pair (Ω; F) with Ω 6= ; and F a σ-algebra on Ω is called a mea- Let W be a BM, then hW it = t, hence dhW is = ds. Def. surable space. Def. (Hilbert space) 2 2 Rem. M ([0; a]) := fM 2 M ([0; a]) j t 7! Mt(!) RCLL for any ! 2 Ωg A Hilbert space is a vector space H with an inner product hf; gisuch d X is measurable (or more precisely Borel-measurable) if for every that the norm defined by Def. B 2 B(R), we have fX 2 Bg 2 F. 2 Def. (indicator function) jfj = phf; gi (i) We denote by H the vector space of all semimartingales va- 2 For any subset A of Ω, the indicator function IA is the function de- nishing at 0 of the form X = M + A with M 2 Md(0; 1) ( turns H into a complete metric space. If the metric defined by the and A 2 FV (finite variation) predictable with total variation 1;! 2 A fined by IA(!) := norm is not complete, then H is instead known as an inner product (1) R 1 2 0; !2 = A V1 (A) = 0 jdAsj 2 L (P ). space. Def. Def. (cauchy-schwarz) (ii) R 0 2 Let Ω 6= ; and a function X :Ω ! (or more generally to Ω ). • jhu; wi j ≤ hu; ui · hw; wi Then σ(X) is the smallest σ-field, say, on Ω s.t. X is measurable 1 1 " Z 1 2# PN PN 2 2 PN 2 2 2 2 (1) 2 with respect to G and B(R) (or G and F 0, respectively). We call • j=1 uj wj ≤ j=1 uj j=1 wj kXkH2 = kMkM2 +kV1 (A)kL2 = E [M]1 + jdAj 0 σ(X) the σ-field generated by X. Steven Battilana p 1 / 23 Mathematical Foundations for Finance Rem. Intuitively, one can compute the conditional expectation We also consider a σ-field generated by a whole familiy of mappings; E[F (U; V )jG] by ’fixing the known value U and taking the ex- this is then the smallest σ-field that makes all the mappings in that pectation over the independent quantity V . Thm. 2.3 family measurable. Suppose (Un)n2N is a sequence of random variables. Def. (probability measure, probability space) 1.2 Conditional expectations: A survival kit (i) If U ≥ XP -a.s. for all n and some integrable random variable If (Ω; F) is a masurable space, a probability measure on F is a map- n X, then ping P : F! [0; 1] s.t. P [Ω] = 1 and P is σ-additive, i.e. Rem. 2 3 E[lim inf UnjG] ≤ lim inf E[UnjG];P -a.s. Let (Ω; F;P ) be a probability space and U a real-valued random n!1 n!1 [ X N P 4 Ai5 = P [Ai];Ai 2 F; i 2 ;Ai \ Aj = ;; i 6= j: variable, i.e. an F-measurable mapping U :Ω ! R. Let G ⊆ F be (ii) If (Un) converges to some random variable UP -a.s. and if N N i2 i2 a fixed sub-σ-field of F; the intuitive interpretation is that G gives jUnj ≤ XP -a.s. for all n and some integrable random variable us some partial information. The goal is then to find a prediction for The triple (Ω; F;P ) is then called a probability space. X, then U on the basis of the information conveyed by G, or a best estimate Def. (P -almost surely) E[ lim U jG] = E[UjG] = lim E[U jG];P -a.s. for U that uses only information from G. n n A statement holds P -almost surely or P -a.s. if the set n!1 n!1 Def. (conditional expectation of U given G) A := f! j the statement does not holdg A conditional expectation of U given G is a real-valued random va- riable Y with the following two properties: is a P -nullset, i.e. has P [A] = 0. We sometimes use instead the for- mulation that a statement holds for P -almost all !. (i) Y is G-measurable Notation (ii) E[UIA] = E[YIA]; 8A 2 G 1.3 Stochastic processes and functions E.g., X ≥ YP -a.s. means that P [X < Y ] = 0, or, equivalently Y is then called a version of the conditional expectation and is de- P [X ≥ Y ] = 1. Notation: noted by Y = E[UjG]. Def. (stochastic process) P [X ≥ Y ] := P [fX ≥ Y g] := P [f! 2 Ω j X(!) ≥ Y (!)g]: Thm. 2.1 A (real-valued) stochastic process with index set T is a family of Let U be an integrable random variable, i.e. U 2 L1(P ). Then: random variables Xt; t 2 T , which are all defined on the same pro- Def. (random variable) bability space (Ω; F;P ). We often write X = (Xt)t2T . Let (Ω; F;P ) be a probability space and X :Ω ! R a measurable (i) There exists a conditional expectation E[UjG], and E[UjG] is Def.
Details
-
File Typepdf
-
Upload Time-
-
Content LanguagesEnglish
-
Upload UserAnonymous/Not logged-in
-
File Pages23 Page
-
File Size-