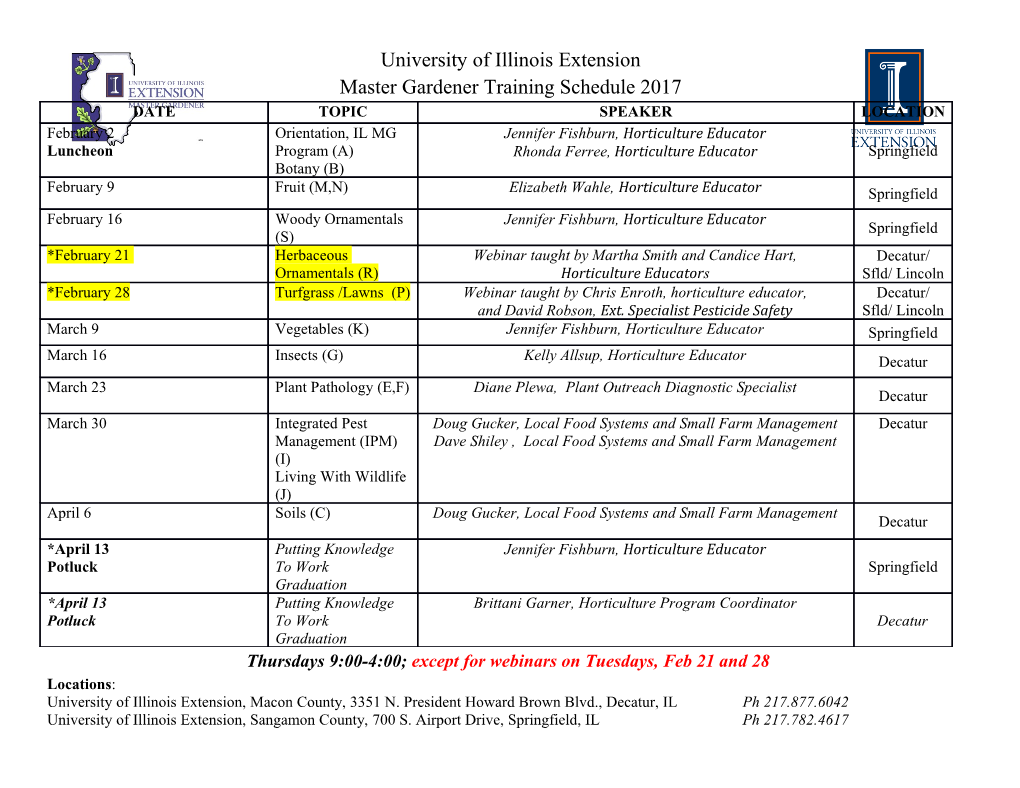
Tutorial 20: Gaussian Measures 1 20. Gaussian Measures Mn(R)isthesetofalln × n-matrices with real entries, n ≥ 1. Definition 141 AmatrixM 2Mn(R) is said to be symmetric, if and only if M = M t. M is orthogonal,ifandonlyifM is non-singular and M −1 = M t.IfM is symmetric, we say that M is non-negative, if and only if: 8u 2 Rn ; hu; Mui≥0 Theorem 131 Let Σ 2Mn(R), n ≥ 1, be a symmetric and non- + negative real matrix. There exist λ1;:::,λn 2 R and P 2Mn(R) orthogonal matrix, such that: 0 1 λ1 0 B . C t Σ=P: @ .. A :P 0 λn t In particular, there exists A 2Mn(R) such that Σ=A:A . www.probability.net Tutorial 20: Gaussian Measures 2 As a rare exception, theorem (131) is given without proof. Exercise 1. Given n ≥ 1andM 2Mn(R), show that we have: 8u; v 2 Rn ; hu; Mvi = hM tu; vi n Exercise 2. Let n ≥ 1andm 2 R .LetΣ2Mn(R) be a symmetric and non-negative matrix. Let µ be the probability measure on R: 1 Z 1 −x2=2 8B 2B(R) ,µ1(B)=p e dx 2π B n Let µ = µ1 ⊗:::⊗µ1 be the product measure on R .LetA 2Mn(R) be such that Σ = A:At.Wedefinethemapφ : Rn ! Rn by: 4 8x 2 Rn ,φ(x) = Ax + m 1. Show that µ is a probability measure on (Rn; B(Rn)). 2. Explain why the image measure P = φ(µ) is well-defined. 3. Show that P is a probability measure on (Rn; B(Rn)). www.probability.net Tutorial 20: Gaussian Measures 3 4. Show that for all u 2 Rn: Z FP (u)= eihu;φ(x)idµ(x) Rn 5. Let v = Atu. Show that for all u 2 Rn: 2 FP (u)=eihu;mi−kvk =2 6. Show the following: n Theorem 132 Let n ≥ 1 and m 2 R .LetΣ 2Mn(R) be a sym- metric and non-negative real matrix. There exists a unique complex n measure on R ,denotedNn(m; Σ), with fourier transform: Z 4 ihu;xi ihu;mi− 1 hu;Σui FNn(m; Σ)(u) = e dNn(m; Σ)(x)=e 2 Rn n for all u 2 R .Furthermore,Nn(m; Σ) is a probability measure. www.probability.net Tutorial 20: Gaussian Measures 4 n Definition 142 Let n ≥ 1 and m 2 R .LetΣ 2Mn(R) be a symmetric and non-negative real matrix. The probability measure n Nn(m; Σ) on R defined in theorem (132) is called the n-dimensional gaussian measure or normal distribution,withmeanm 2 Rn and covariance matrix Σ. n Exercise 3. Let n ≥ 1andm 2 R . Show that Nn(m; 0) = δm. n Exercise 4. Let m 2 R .LetΣ2Mn(R) be a symmetric and t non-negative real matrix. Let A 2Mn(R) be such that Σ = A:A . Amapp : Rn ! C is said to be a polynomial, if and only if, it is a finite linear complex combination of maps x ! xα,1 for α 2 Nn. 1. Show that for all B 2B(R), we have: Z 1 −x2=2 N1(0; 1)(B)=p e dx 2π B 1See definition (140). www.probability.net Tutorial 20: Gaussian Measures 5 2. Show that: Z +1 jxjdN1(0; 1)(x) < +1 −∞ 3. Show that for all integer k ≥ 1: Z Z +1 +1 1 2 k 2 p xk+1e−x =2dx = p xk−1e−x =2dx 2π 0 2π 0 4. Show that for all integer k ≥ 0: Z +1 k jxj dN1(0; 1)(x) < +1 −∞ 5. Show that for all α 2 Nn: Z α jx jdN1(0; 1) ⊗ :::⊗ N1(0; 1)(x) < +1 Rn www.probability.net Tutorial 20: Gaussian Measures 6 6. Let p : Rn ! C be a polynomial. Show that: Z jp(x)jdN1(0; 1) ⊗ :::⊗ N1(0; 1)(x) < +1 Rn 7. Let φ : Rn ! Rn be defined by φ(x)=Ax + m. Explain why the image measure φ(N1(0; 1) ⊗ :::⊗ N1(0; 1)) is well-defined. 8. Show that φ(N1(0; 1) ⊗ :::⊗ N1(0; 1)) = Nn(m; Σ). 9. Show if β 2 Nn and jβj =1,thenx ! φ(x)β is a polynomial. 0 10. Show that if α0 2 Nn and jα0j = k+1, then φ(x)α = φ(x)αφ(x)β for some α, β 2 Nn such that jαj = k and jβj =1. 11. Show that the product of two polynomials is a polynomial. 12. Show that for all α 2 Nn, x ! φ(x)α is a polynomial. 2 n 13. Show thatZ for all α N : α jφ(x) jdN1(0; 1) ⊗ :::⊗ N1(0; 1)(x) < +1 Rn www.probability.net Tutorial 20: Gaussian Measures 7 14. Show the following: n Theorem 133 Let n ≥ 1 and m 2 R .LetΣ 2Mn(R) be a sym- metric and non-negative real matrix. Then, for all α 2 Nn,themap α x ! x is integrable with respect to the gaussian measure Nn(m; Σ): Z α jx jdNn(m; Σ)(x) < +1 Rn n Exercise 5. Let m 2 R .LetΣ=(σij ) 2Mn(R) be a symmetric and non-negative real matrix. Let j; k 2 Nn.Letφ be the fourier transform of the gaussian measure Nn(m; Σ), i.e.: n 4 ihu;mi− 1 hu;Σui 8u 2 R ,φ(u) = e 2 1. Show that: Z −1 ∂φ xj dNn(m; Σ)(x)=i (0) Rn @uj www.probability.net Tutorial 20: Gaussian Measures 8 2. Show that: Z xjdNn(m; Σ)(x)=mj Rn 3. Show that: Z 2 −2 @ φ xj xkdNn(m; Σ)(x)=i (0) Rn @uj@uk 4. Show that: Z xj xkdNn(m; Σ)(x)=σjk + mjmk Rn 5. Show that:Z (xj − mj)(xk − mk)dNn(m; Σ)(x)=σjk Rn www.probability.net Tutorial 20: Gaussian Measures 9 n Theorem 134 Let n ≥ 1 and m 2 R .LetΣ=(σij ) 2Mn(R) be a symmetric and non-negative real matrix. Let Nn(m; Σ) be the gaussian measure with mean m and covariance matrix Σ.Then,for all j; k 2 N , we have: n Z xjdNn(m; Σ)(x)=mj Rn and: Z (xj − mj)(xk − mk)dNn(m; Σ)(x)=σjk Rn Definition 143 Let n ≥ 1.Let(Ω; F;P) be a probability space. Let X :(Ω; F) ! (Rn; B(Rn)) be a measurable map. We say that X is an n-dimensional gaussian or normal vector, if and only if its distribution is a gaussian measure, i.e. X(P )=Nn(m; Σ) for some n m 2 R and Σ 2Mn(R) symmetric and non-negative real matrix. Exercise 6. Show the following: www.probability.net Tutorial 20: Gaussian Measures 10 Theorem 135 Let n ≥ 1.Let(Ω; F;P) be a probability space. Let X :(Ω; F) ! Rn be a measurable map. Then X is a gaussian vector, n if and only if there exist m 2 R and Σ 2Mn(R) symmetric and non-negative real matrix, such that: n ihu;Xi ihu;mi− 1 hu;Σui 8u 2 R ;E[e ]=e 2 where h·; ·i is the usual inner-product on Rn. Definition 144 Let X :(Ω; F) ! R¯ (or C) be a random variable on a probability space (Ω; F;P). We say that X is integrable,ifand only if we have E[jXj] < +1. We say that X is square-integrable, if and only if we have E[jXj2] < +1. Exercise 7. Further to definition (144), suppose X is C-valued. 2 1 F 1. Show X is integrable if and only if X LC(Ω; ;P). 2 2 F 2. Show X is square-integrable, if and only if X LC(Ω; ;P). www.probability.net Tutorial 20: Gaussian Measures 11 Exercise 8. Further to definition (144), suppose X is R¯ -valued. 1. Show that X is integrable, if and only if X is P -almost surely 1 F equal to an element of LR(Ω; ;P). 2. Show that X is square-integrable, if and only if X is P -almost 2 F surely equal to an element of LR(Ω; ;P). Exercise 9. Let X; Y :(Ω; F) ! (R; B(R)) be two square-integrable random variables on a probability space (Ω; F;P). 1. Show that both X and Y are integrable. 2. Show that XY is integrable 3. Show that (X−E[X])(Y −E[Y ]) is a well-defined and integrable. www.probability.net Tutorial 20: Gaussian Measures 12 Definition 145 Let X; Y :(Ω; F) ! (R; B(R)) be two square- integrable random variables on a probability space (Ω; F;P).Wede- fine the covariance between X and Y ,denotedcov(X; Y ),as: 4 cov(X; Y ) = E[(X − E[X])(Y − E[Y ])] We say that X and Y are uncorrelated if and only if cov(X; Y )=0. If X = Y , cov(X; Y ) is called the variance of X,denotedvar(X). Exercise 10. Let X; Y be two square integrable, real random variable on a probability space (Ω; F;P). 1. Show that cov(X; Y )=E[XY] − E[X]E[Y ]. 2. Show that var(X)=E[X2] − E[X]2. 3. Show that var(X + Y )=var(X)+2cov(X; Y )+var(Y ) 4. Show that X and Y are uncorrelated, if and only if: var(X + Y )=var(X)+var(Y ) www.probability.net Tutorial 20: Gaussian Measures 13 Exercise 11.
Details
-
File Typepdf
-
Upload Time-
-
Content LanguagesEnglish
-
Upload UserAnonymous/Not logged-in
-
File Pages23 Page
-
File Size-