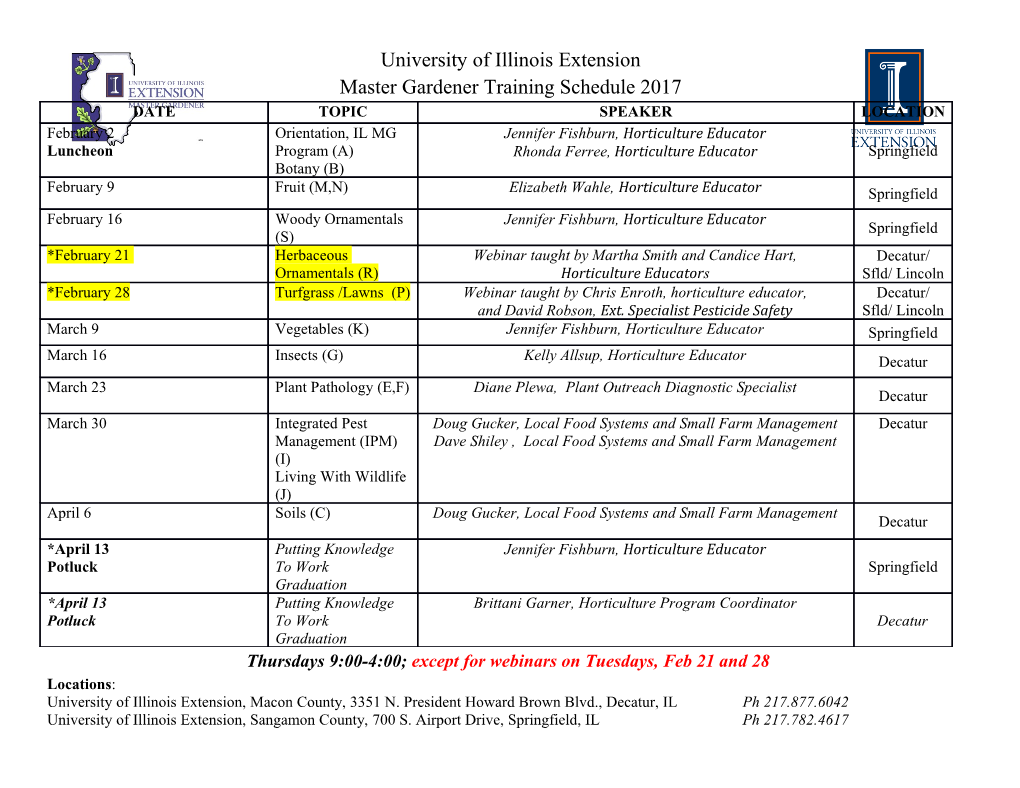
ROB 537: Learning Based Control Week 6, Lecture 2 Recurrent Neural Networks Artificial Neural Networks • • • Your Name | Event Name 1 Assumptions • • Your Name | Event Name 2 Example: XOR Hidden layer • – Output layer Input 1 Input 2 Your Name | Event Name 3 Example: XOR with single input channel Hidden layer • – Output layer • Input – – Your Name | Event Name 4 Example: XOR with single input channel; Hidden • Intermediate output – Input 1 t1 Hidden • Output – Input 2 t2 – Your Name | Event Name 5 Example: XOR • – Your Name | Event Name 6 Example: XOR • – • Your Name | Event Name 7 Example: XOR • – • • – – – Your Name | Event Name 8 Some approaches • 9 Some approaches • – – 10 Some approaches • – – • Recurrent Neural Networks (RNNs) – Use ‘recurrent connections’ to bridge information flow across time 11 XOR problem with one input channel Hidden Intermediate output Input 1 t1 Hidden Output Input 2 t2 12 XOR problem with one input channel Hidden • Intermediate output Input 1 • t1 Hidden Output Input 2 t2 Recurrent Connection 13 XOR problem with one input channel Hidden • Intermediate output Input 1 • t1 Hidden Output Input 2 • t2 Recurrent Connection 14 Recurrent Connections Hidden • Intermediate output Input 1 t1 Hidden Output Input 2 t2 15 Recurrent Connections Hidden • Intermediate output Input 1 t1 Hidden Output Input 2 t2 16 Recurrent Connections Hidden • Intermediate output Input 1 t1 Hidden Output Input 2 t2 17 Recurrent Neural Networks Ian Goodfellow, Yoshua Bengio, and Aaron Courville. Deep Learning. MIT Press, 2016. • 18 Recurrent Neural Networks Ian Goodfellow, Yoshua Bengio, and Aaron Courville. Deep Learning. MIT Press, 2016. • • 19 Recurrent Neural Networks Ian Goodfellow, Yoshua Bengio, and Aaron Courville. Deep Learning. MIT Press, 2016. • • 20 Overview of RNNs • • 21 Overview of RNNs • • • • 22 Training a RNN • • • 23 Solution • – – 24 Solution • – – Ian Goodfellow, Yoshua Bengio, and Aaron Courville. Deep Learning. MIT Press, 2016. 25 Gating mechanisms • • 26 Gating mechanisms • • Input Hidden Output Input gate 27 Standard RNN (SRN) Source: Deeplearning4J Source: Deeplearning4J 28 Long short term memory (LSTM) Source: Deeplearning4J 29 Long short term memory (LSTM) • • • • LSTM (Chung et al.) 30 Gated Recurrent Unit (GRU) • • • GRU (Chung et al.) 31 GRU and LSTM • • GRU (Chung et al.) LSTM (Chung et al.) 32 Topology Limitation • • GRU (Chung et al.) LSTM (Chung et al.) 33 Gated Recurrent Unit with Memory Block (GRU-MB) • • Khadka, Chung and Tumer. GECCO 2017 34 Feedforward NN 35 Read from external memory 36 Write to memory 37 Gate Input 38 Gate what’s read from memory 39 Gate what’s written to memory 40 Topology comparison GRU-MB GRU (Chung et al.) LSTM (Chung et al.) 41 Topology comparison GRU-MB GRU (Chung et al.) LSTM (Chung et al.) 42 Topology comparison GRU-MB GRU (Chung et al.) LSTM (Chung et al.) 43 RNNs with Attention Mechanisms • • • NTM (Graves 2016) 44.
Details
-
File Typepdf
-
Upload Time-
-
Content LanguagesEnglish
-
Upload UserAnonymous/Not logged-in
-
File Pages23 Page
-
File Size-