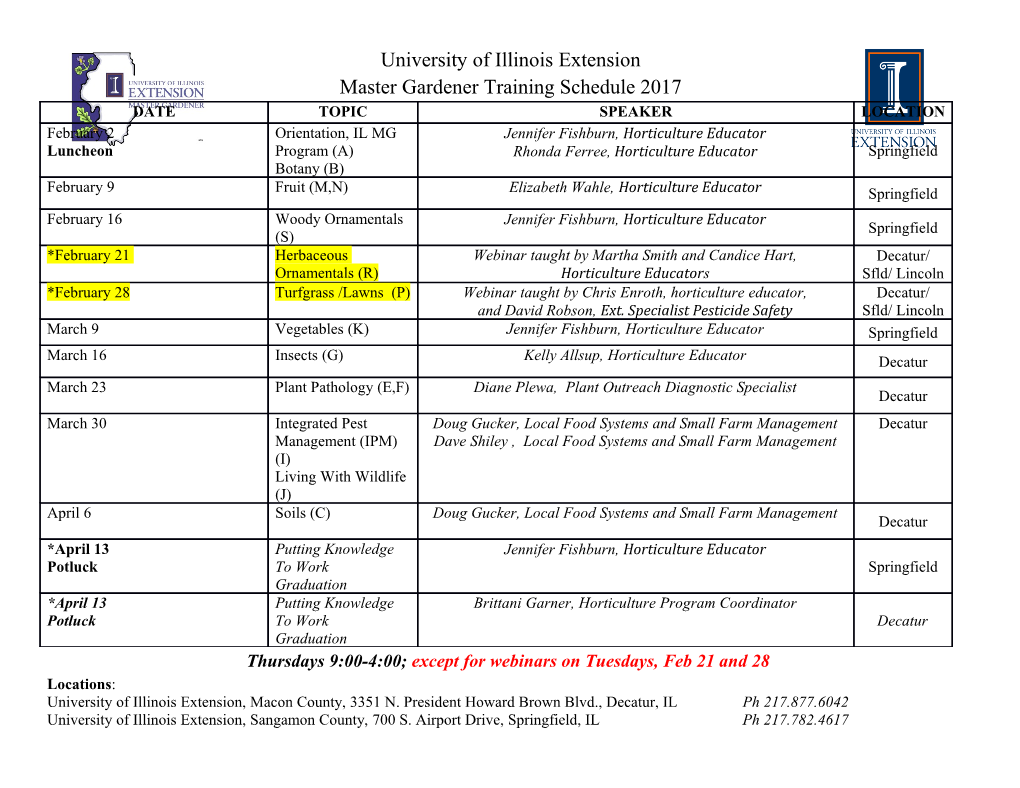
An introduction to convex and discrete geometry Lecture Notes Tomasz Tkocz∗ These lecture notes were prepared and written for the undergraduate topics course 21-366 An introduction to convex and discrete geometry that I taught at Carnegie Mellon University in Fall 2019. ∗Carnegie Mellon University; [email protected] 1 Contents 1 Introduction 5 1.1 Euclidean space . .5 1.2 Linear and affine hulls . .7 1.3 Exercises . 10 2 Basic convexity 12 2.1 Convex hulls . 12 2.2 Carath´eodory's theorem . 14 2.3 Exercises . 16 3 Separation 17 3.1 Supporting hyperplanes . 17 3.2 Separation theorems . 21 3.3 Application in linear optimisation { Farkas' lemma . 24 3.4 Exercises . 25 4 Further aspects of convexity 26 4.1 Extreme points and Minkowski's theorem . 26 4.2 Extreme points of polytopes . 27 4.3 Exercises . 29 5 Polytopes I 30 5.1 Duality . 31 5.2 Bounded polyhedra are polytopes . 36 5.3 Exercises . 38 6 Polytopes II 39 6.1 Faces . 39 6.2 Cyclic polytopes have many faces . 40 6.3 Exercises . 45 7 Combinatorial convexity 46 7.1 Radon's theorem . 46 7.2 Helly's theorem . 47 7.3 Centrepoint . 49 7.4 Exercises . 51 8 Arrangements and incidences 52 8.1 Arrangements . 52 8.2 Incidences . 54 2 8.3 The crossing number of a graph . 56 8.4 Proof of the Szemer´edi-Trotter theorem . 58 8.5 Application in additive combinatorics . 59 8.6 Exercises . 61 9 Volume 62 9.1 The Brunn-Minkowski inequality . 62 9.2 Isoperimetric and isodiametric inequality . 66 9.3 Epsilon nets . 68 9.4 Concentration of measure on the sphere . 69 9.5 Exercises . 71 10 Equilateral and equiangular sets 72 10.1 Upper bound for equilateral sets for arbitrary norms . 73 10.2 Upper bound for equilateral sets for Euclidean norms . 73 10.3 Upper bound for equilateral sets for the `1 norm . 75 10.4 Upper bound for equiangular sets . 76 10.5 Exercises . 80 11 Diameter reduction { Borsuk's question 81 11.1 A result from extremal set theory . 81 11.2 Construction via tensor product . 83 11.3 A positive answer in dimension 2 . 84 11.4 Exercises . 86 12 Zonotopes and projections of the cube 87 12.1 Dissections and volume of zonotopes . 88 12.2 Volume of projections of the cube on orthogonal subspaces . 89 12.3 Exercises . 92 13 Minkowski's theorem in geometry of numbers 93 13.1 Minkowski's theorem . 93 13.2 Application: approximations by rationals . 96 13.3 Application: The sum of two squares theorem . 97 13.4 Exercises . 99 14 The plank problem 100 14.1 Archimedes' Hat-Box Theorem and a solution on the plane . 100 14.2 Bang's solution . 102 14.3 Exercises . 106 3 A Appendix: Euler's formula 107 4 1 Introduction 1.1 Euclidean space d We shall work in d-dimensional Euclidean space R = (x1; : : : ; xd); xi R; i d , f 2 ≤ g the space of all length d real sequences x = (x1; : : : ; xd). Its elements are called points or vectors, the origin is 0 = (0;:::; 0). It is a real vector space. We add vectors coordiante-wise, d x + y = (x1 + y1; : : : ; xd + yd); x; y R ; 2 and the same for scalar multiplication d λx = (λx1; : : : ; λxd); λ R; x R : 2 2 The standard basis consits of the vectors e1 = (1; 0; 0;:::; 0; 0), e2 = (0; 1; 0;:::; 0; 0), Pd and so on, ed = (0; 0; 0;:::; 0; 1) and plainly x = j=1 xjej. Addition of vectors suggests a natural way of adding nonempty subsets A, B of Rd, which is defined by A + B = a + b; a A; b B f 2 2 g and called the Minkowski sum of A and B. Similarly for multiplication by a scalar λ, λA = λa, a A ; f 2 g called the dilation of A. In particular, ( 1)A, denoted A is the symmetric image of − − A. The set A is called symmetric if A = A. − A + v v A 0 Figure 1.1: A + v is A translated by v. 1.1 Example. The Minkowski sum involving a singleton, say A+ v is the translation f g of A by v, denoted A + v, or v + A (see Figure 1.1). 1.2 Example. The Minkowski sum of a square and a disk is a rounded square (see Figure 1.2). 5 A + B A B b b b Figure 1.2: The Minkowski sum of a square and a disk. 1.3 Example. The Minkowski sum of two intervals is a a parallelogram. The Minkowski sum of several intervals is a polygon (see Figure 1.3). A + B + C C B A Figure 1.3: The Minkowski sum of several intervals. The Euclidean structure of Rd is given by the standard scalar product d X d x; y = xjyj; x; y R : h i 2 j=1 Recall the defining properties of a scalar product: ; : Rd Rd R is symmetric and h· ·i × ! bilinear and satisfies x; x 0, for every x Rd, with equality if and only if x = 0. The h i≥ 2 Euclidean norm of x is p sX x = x; x = x2 j j h i j j which is its distance to the origin. Recall that a function : Rd R is called a norm k · k ! if: 1) x 0, for every x Rd with equality if and only if x = 0, 2) λx = λ x , for k k ≥ 2 k k j jk k every λ R and x Rd, 3) x + y x + y , for every x; y Rd. The (Euclidean) 2 2 k k ≤ k k k k 2 distance between x and y in Rd is d(x; y) = x y j − j 6 which defines the Euclidean metric on Rd. In general, every scalar product gives rise p to a norm by x = x; x . The triangle inequality is a consequence of the Cauchy- k k h i Schwarz inequality. 1.4 Theorem. If x; y Rd, then x; y x y . Equality holds if and only if x = λy 2 jh ij ≤ j j · j j or y = λx for some λ R. 2 Proof. If y = 0, the statement is clear. Assume y = 0 and consider the following 6 polynomial of degree 2 P (t) = x ty; x ty = x 2 + t2 y 2 2t x; y : h − − i j j j j − h i Since P is nonnegative and the leading coefficient y 2 is positive, the discriminant of P j j is nonpositive, hence 4 x; y 2 4 x 2 y 2 0. If we have equality, P has a zero at say h i − j j j j ≤ λ, that is 0 = P (λ) = x λy; x λy which by the axioms of a scalar product implies h − − i that x λy = 0. − 1.2 Linear and affine hulls d Pk A linear combination of points x1; : : : ; xk in R is λjxj, where λj R. We j=1 2 call it an affine combination if additionally the scalars (weights) λj add up to 1, Pk j=1 λj = 1. We define the linear hull (or simply the span) of points x1; : : : ; xk as the set of all linear combinations of x1; : : : ; xk. Similarly for the affine hull. 1.5 Example. The linear hull of two points a; b is a line if they are colinear with the origin and otherwise, it is a plane. The affine hull of two different points a; b is the line 3 3 passing through a and b. The linear hull of e1; e2; e3 in R is R and their affine hull is 3 the plane x R ; x1 + x2 + x3 = 1 . f 2 g 0 b span{a,b} a aff{a,b} Figure 1.4: The affine and linear hull of two points. The definitions of linear and affine hulls extend from finite sets to arbitrary ones. That is, given a subset A of Rd, its affine hull is defined as 7 aff(A) = all affine combinations of points from A f g ( k k ) X X = λixi; k 1; ai A; λi R; λi = 1 : ≥ 2 2 i=1 i=1 Similarly for the linear hull of A, denoted lin(A) or span(A). A set A in Rd is called a flat (or an affine subspace) if it is a translate of a subspace d F , that is A = F + x0 for some x0 R . The dimension of A is the dimension of F . 2 We have a basic result relating flats and affine hulls. d 1.6 Theorem. If x1; : : : ; xk are points in R , then their affine hull is (a) a flat, (b) the smallest flat containing them, that is if x ; : : : ; x F for some flat F , then 1 k 2 aff(x ; : : : ; x ) F , 1 k ⊂ (c) aff(x ; : : : ; x ) = T F : F is a flat containing x ; : : : ; x . 1 k f 1 kg Proof. Exercise. Some affine subspaces have spacial names: 1-dimensional ones are called lines, 1- codimensional ones are called hyperplanes and in general k-dimensional ones are called d k-flats. A hyperplane H in R can be specified by a single linear equation a1x1 + + ··· d adxd = b, that is H = x R ; a; x = b and H is perpendicular to a. It gives rise to f 2 h i g d + d two closed half-spaces: H− = x R ; a; x b and H = x R ; a; x b .
Details
-
File Typepdf
-
Upload Time-
-
Content LanguagesEnglish
-
Upload UserAnonymous/Not logged-in
-
File Pages111 Page
-
File Size-