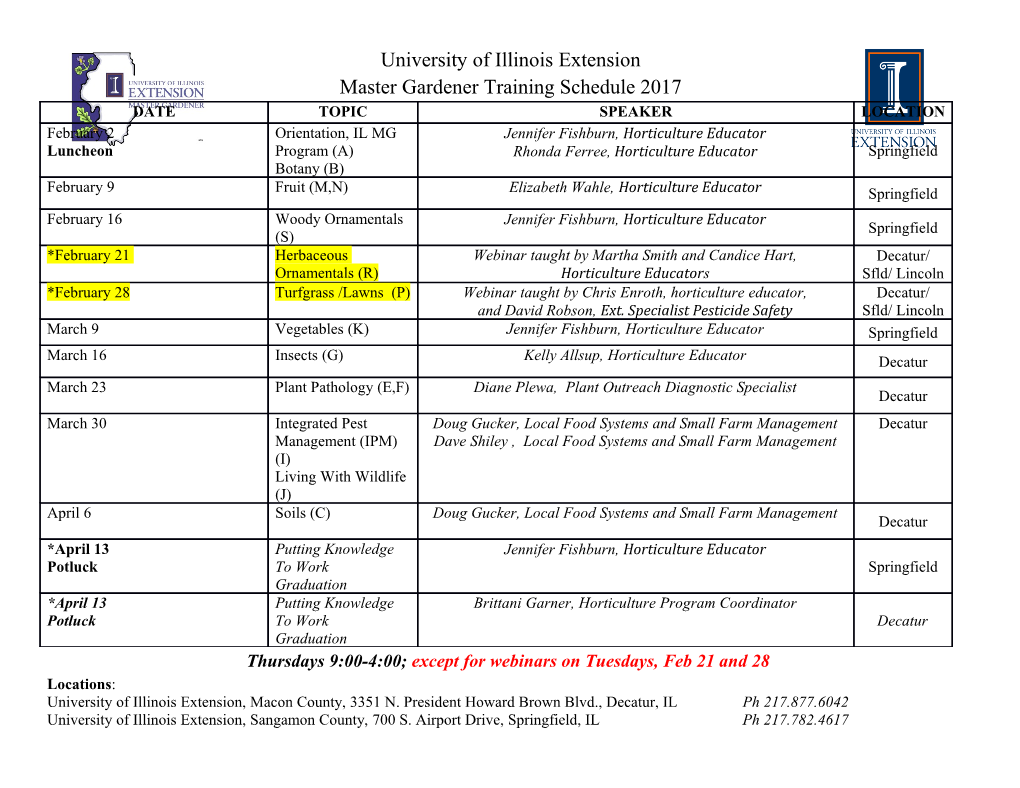
Topic 4: Multivariate random variables Joint, marginal, and conditional pmf ² Joint, marginal, and conditional pdf and cdf ² Independence ² Expectation, covariance, correlation ² Conditional expectation ² Two jointly Gaussian random variables ² ES150 { Harvard SEAS 1 Multiple random variables In many problems, we are interested in more than one random ² variables representing di®erent quantities of interest from the same experiment and the same sample space. { Examples: The tra±c loads at di®erent routers in a network, the received quality at di®erent HDTVs, the shuttle arrival time at di®erent stations. These random variables can be represented by a random vector X that ² assign a vector of real number to each outcome s in the sample space ­. X = (X1; X2; : : : ; Xn) It prompts us to investigate the mutual coupling among these random ² variables. { We will study 2 random variables ¯rst, before generalizing to a vector of n elements. ES150 { Harvard SEAS 2 Joint cdf The joint cdf of two random variables X and Y speci¯es the ² probability of the event X x Y y f · g \ f · g F (x; y) = P [X x; Y y] X;Y · · The joint cdf is de¯ned for all pairs of random variables. Properties of the joint cdf: ² { Non-negativity: F (x; y) 0 X;Y ¸ { Non-decreasing: If x x and y y , 1 · 2 1 · 2 then F (x ; y ) F (x ; y ) X;Y 1 1 · X;Y 2 2 { Boundedness: lim FX;Y (x; y) = 1 x;y!1 lim FX;Y (x; y) = lim FX;Y (x; y) = 0 x!¡1 y!¡1 ES150 { Harvard SEAS 3 { Marginal cdf's: which are the individual cdf's FX (x) = lim FX;Y (x; y) y!1 The marginal cdf's can be obtained from the joint cdf, but usually not the reverse. In general, knowledge of all marginal cdf's is insu±cient to specify the joint cdf. { Rectangle formula: P [a < X b; c < Y d] · · = F (b; d) F (b; c) F (a; d) + F (a; c) X;Y ¡ X;Y ¡ X;Y X;Y ES150 { Harvard SEAS 4 Discrete random variables { Joint pmf Consider two discrete random variables X and Y . ² They are completely speci¯ed by their joint pmf, which speci¯es the ² probability of the event X = x; Y = y f g pX;Y (xk; yj) = P (X = xk; Y = yj) The probability of any event A is given as ² P ((X; Y ) A) = p (x ; y ) 2 X;Y k j (xkX;yj )2A By the axioms of probability ² p (x ; y ) 0 X;Y k j ¸ 1 1 pX;Y (xk; yj) = 1 kX=1 Xj=1 ES150 { Harvard SEAS 5 Marginal and conditional pmf's From the joint pmf P (x; y), we can calculate the individual pmf ² X;Y pX (x) and pY (y), which are now referred to as the marginal pmf 1 pX (xi) = pX;Y (xi; yj) Xj=1 1 Similarly pY (yk) = i=1 pX;Y (xi; yk). { The marginal pmfPis an one-dimensional pmf. { In general, knowledge of all marginal pmf's is insu±cient to specify the joint pmf. Sometimes we know one of the two random variables, and we are ² interested in the probability of the other one. This is captured in the conditional pmf pX;Y (xi; yk) pXjY (X = xi Y = yk) = j pY (yk) provided p (y ) = 0. Otherwise de¯ne p (x y ) = 0. Y k 6 XjY j k ES150 { Harvard SEAS 6 Example: The binary symmetric channel Consider the following binary communication channel Z 0; 1 2 f g X 0; 1 Y 0; 1 2 f g 2 f g The bit sent is X Bern(p); 0 p 1 » · · The noise is Z Bern(²); 0 ² 0:5 » · · The bit received is Y = (X + Z) mod 2 = X Z © where X and Z are independent. Find the following probabilities: 1. pY (y); 2. p (x y); XjY j 3. P [X = Y ], the probability of error. 6 ES150 { Harvard SEAS 7 Jointly continuous random variables { Joint, marginal, and condition pdf Two random variables X and Y are jointly continuous if the ² probability of any event involving (X; Y ) can be expressed as an integral of a probability density function P [(X; Y ) A] = fX;Y (x; y) dx dy 2 Z ZA { fX;Y (x; y) is called the joint probability density function { It is possible to have two continuous random variables that are not jointly continuous. For jointly continuous random variables ² x y FX;Y (x; y) = fX;Y (u; v) dv du Z¡1 Z¡1 @2F (x; y) f (u; v) = X;Y X;Y @x@y Since FX;Y (x; y) is non-decreasing, the joint density is always ES150²{ Harvard SEAS 8 non-negative f (x; y) 0 X;Y ¸ But fX;Y (x; y) is NOT a probability measure (it can be > 1). By the axioms of probability, ² 1 1 fX;Y (x; y) dx dy = 1 Z¡1 Z¡1 The marginal pdf can be obtained from the joint pdf as ² 1 fX (x) = fX;Y (x; y)dy Z¡1 The conditional pdf is given as ² fX;Y (x; y) fXjY (x y) = j fY (y) Example: Consider two jointly Gaussian random variables with the ² joint pdf 1 x2 2½xy + y2 fX;Y (x; y) = exp ¡ 2¼ 1 ½2 ½¡ 2(1 ½2) ¾ ¡ ¡ ES150 { Harvard SEAS p 9 { The marginal pdf of X is Gaussian 2 1 ¡x =2 fX (x) = e p2¼ { The conditional pdf of X given Y is also Gaussian 1 (x ½y)2 fX (x y) = exp ¡ j 2¼(1 ½2) ½¡ 2(1 ½2) ¾ ¡ ¡ p ES150 { Harvard SEAS 10 Independence Independence between 2 random variables X and Y means ² P (X B; Y C) = P (X B) P (Y C) 2 2 2 2 Two random variables X and Y are independent if and only if ² FX;Y (x; y) = FX (x)FY (y) Equivalently, in terms of the joint and conditional densities ² { For discrete r.v.'s: pX;Y (xi; yk) = pX (xi)pY (yk) p (x y ) = p (x ) XjY ij k X i { For jointly continuous r.v.'s: fX;Y (x; y) = fX (x)fY (y) f (x y) = f (x) XjY j X ES150 { Harvard SEAS 11 X and Y independent implies ² E[XY ] = E[X]E[Y ] The reverse is not always true. That is, E[XY ] = E[X]E[Y ] does not necessarily imply X and Y are independent. Example: Consider discrete RVs X and Y such that ² 1 P [X = 1] = P [X = 2] = and Y = X2 § § 4 Then E[X] = 0 and E[XY ] = 0, but X and Y are not independent. Find pY (1), pY (4), pXY (1; 4). ES150 { Harvard SEAS 12 Expectation, covariance, correlation Let g(x; y) be a function of two random variables X and Y . The ² expectation of g(X; Y ) is given by g(xi; yk)pX;Y (xi; yk) discrete X; Y 8 E[g(X; Y )] = Xk Xi > 1 1 < g(x; y)fX;Y (x; y) dx dy jointly continuous X; Y Z¡1 Z¡1 > The covarianc:e between 2 random variables is de¯ned as ² cov(X; Y ) = E X E[X] Y E[Y ] ¡ ¡ = E[£XY ] E[X¤£]E[Y ] ¤ ¡ { If E[X] = 0 or E[Y ] = 0 then cov(X; Y ) = E[XY ]. { Covariance is analogous to the variance of a single random variable: cov(X; X) = var(X). E[XY ] is the correlation between the two random variables. ² { By the Cauchy-Schwatz inequality E[XY ] E[X2]E[Y 2] j j · ES150 { Harvard SEAS p 13 X and Y are said to be (note the di®erence in terminology here) ² { orthogonal if E[XY ] = 0 { uncorrelated if cov(X; Y ) = 0 The correlation coe±cient is de¯ned as ² cov(X; Y ) ½X;Y = X Y where X = var(X) and Y = var(Y ) are the standard deviations. Properties: p p { Bounded: 1 ½ 1 ¡ · X;Y · { If ½X;Y = 0, X and Y are uncorrelated { If X and Y are independent, then ½X;Y = 0. Independence implies uncorrelation, but not the reverse. For Gaussian random variables X and Y , however, if they are ² uncorrelated, then they are also independent. ES150 { Harvard SEAS 14 Conditional expectation The conditional expectation of X given Y is de¯ned as ² { For discrete r.v.'s: E X Y = y = x p (x y ) j k i XjY ij k £ ¤ Xi { For jointly continuous r.v.'s: 1 E X Y = y = x f (x y)dx j Z XjY j £ ¤ ¡1 Similarly for the conditional expectation of a function of X, given Y ² g(x )p (x y) for discrete X; Y i XjY ij 8 i E [g(X) Y = y] = X1 j > < g(x) fXjY (x y)dx for jointly continuous X; Y Z¡1 j > :> The law of conditional expectation ² E[g(X)] = E E[g(X) Y ] Y j ES150 { Harvard SEAS £ ¤ 15 For discrete r.v.'s E[g(X)] = E g(X) y p (y ) j k Y k Xk £ ¤ For continuous r.v.'s 1 E[g(X)] = E g(X) y fY (y)dy Z j ¡1 £ ¤ This law is very useful in calculating the expectation. Example: Defects in a chip. ² { The total number of defects on a chip is X Poisson(®). » { The probability of ¯nding an defect in the memory is p. Find the expected number of defects in the memory. ES150 { Harvard SEAS 16 Two jointly Gaussian random variables The joint pdf of two jointly Gaussian r.v.'s X and Y is 2 2 1 (x¡¹X ) (x¡¹X )(y¡¹Y ) (y¡¹Y ) exp 2 2 2½ + 2 2(1¡½ ) XY X Y ¡ XY X ¡ Y fX;Y (x; y) = n h io 2¼ 1 ½2 X Y ¡ XY p The pdf is a function only of ¹ , ¹ , , , and ½ .
Details
-
File Typepdf
-
Upload Time-
-
Content LanguagesEnglish
-
Upload UserAnonymous/Not logged-in
-
File Pages9 Page
-
File Size-