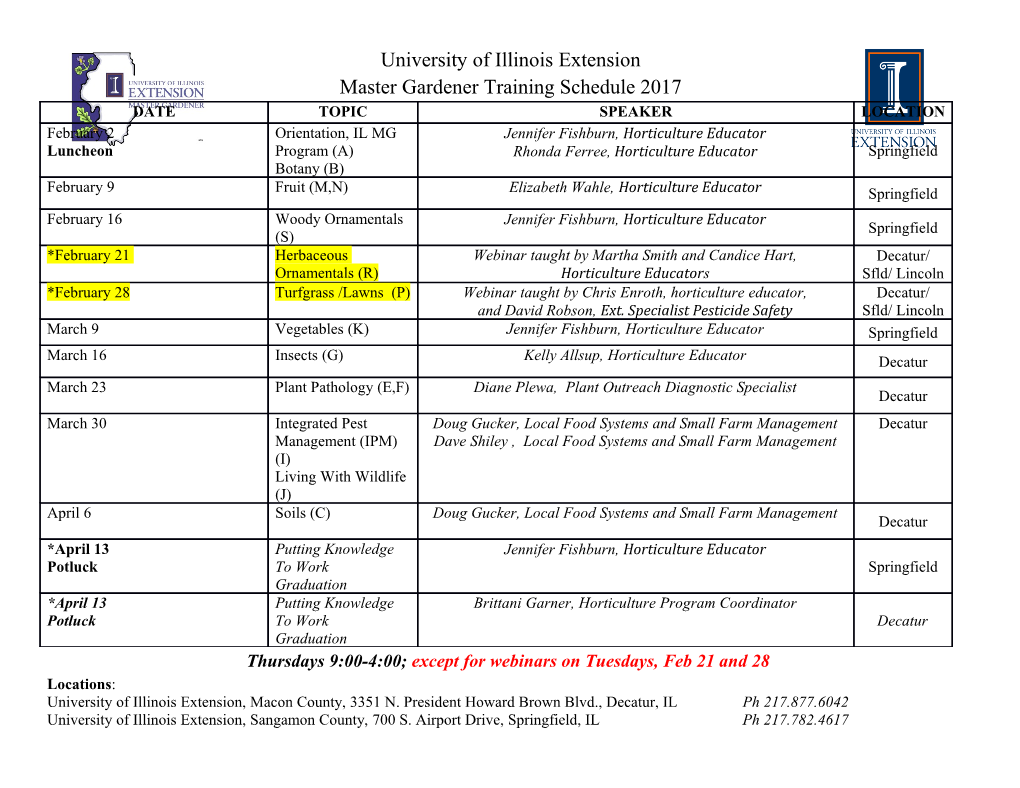
Simulated Annealing A Basic Introduction Prof. Olivier de Weck Massachusetts Institute of Technology Dr. Cyrus Jilla Outline • Heuristics • Background in Statistical Mechanics – Atom Configuration Problem – Metropolis Algorithm • Simulated Annealing Algorithm • Sample Problems and Applications • Summary Learning Objectives • Review background in Statistical Mechanics: configuration, ensemble, entropy, heat capacity • Understand the basic assumptions and steps in Simulated Annealing • Be able to transform design problems into a combinatorial optimization problem suitable to SA • Understand strengths and weaknesses of SA Heuristics What is a Heuristic? • A Heuristic is simply a rule of thumb that hopefully will find a good answer. • Why use a Heuristic? – Heuristics are typically used to solve complex (large, nonlinear, nonconvex (ie. contain many local minima)) multivariate combinatorial optimization problems that are difficult to solve to optimality. • Unlike gradient-based methods in a convex design space, heuristics are NOT guaranteed to find the true global optimal solution in a single objective problem, but should find many good solutions (the mathematician's answer vs. the engineer’s answer) • Heuristics are good at dealing with local optima without getting stuck in them while searching for the global optimum. Types of Heuristics • Heuristics Often Incorporate Randomization • 2 Special Cases of Heuristics – Construction Methods • Must first find a feasible solution and then improve it. – Improvement Methods • Start with a feasible solution and just try to improve it. • 3 Most Common Heuristic Techniques – Genetic Algorithms – Simulated Annealing – Tabu Search – New Methods: Particle Swarm Optimization, etc… Origin of Simulated Annealing (SA) • Definition: A heuristic technique that mathematically mirrors the cooling of a set of atoms to a state of minimum energy. • Origin: Applying the field of Statistical Mechanics to the field of Combinatorial Optimization (1983) • Draws an analogy between the cooling of a material (search for minimum energy state) and the solving of an optimization problem. • Original Paper Introducing the Concept – Kirkpatrick, S., Gelatt, C.D., and Vecchi, M.P., “Optimization by Simulated Annealing,” Science, Volume 220, Number 4598, 13 May 1983, pp. 671­ 680. Statistical Mechanics The Analogy • Statistical Mechanics: The behavior of systems with many degrees of freedom in thermal equilibrium at a finite temperature. • Combinatorial Optimization: Finding the minimum of a given function depending on many variables. • Analogy: If a liquid material cools and anneals too quickly, then the material will solidify into a sub-optimal configuration. If the liquid material cools slowly, the crystals within the material will solidify optimally into a state of minimum energy (i.e. ground state). – This ground state corresponds to the minimum of the cost function in an optimization problem. Sample Atom Configuration Original Configuration Perturbed Configuration Atom Configuration - Sample Problem Atom Configuration - Sample Problem 7 7 6 6 5 5 4 3 4 3 4 3 4 2 2 2 3 2 1 1 1 1 0 0 -1 -1 -1 0 1 2 3 4 5 6 7 -1 0 1 2 3 4 5 6 7 E=133.67 E=109.04 Perturbing = move a random atom Energy of original (configuration) to a new random (unoccupied) slot Configurations • Mathematically describe a configuration – Specify coordinates of each “atom” Ø1ø Ø 3 øØ 4 ø Øø 5 {ri } with i=1,..,4 r1 = Œœ, r2 = Œ œ, r3 = Œ œ, r4 = Œœ º1ß º2 ß º4 ß ºß3 – Specify a slot for each atom {ri} with i=1,..,4 R = [1 12 19 23] Energy of a state • Each state (configuration) has an energy level associated with it H( qq,�,t) = � q�iip- L( qqt ,,� ) i Hamiltonian H =+=T VEtot Energy of configuration = Objective function value of design kinetic potential Energy Energy sample problem • Define energy function for “atom” sample problem 1 N 2 2 E = mgy + x - x + y - y 2 i i �(( i j ) ( i j ) ) potential� ji„ energy ������������� kinetic energy N Absolute and relative position of E( R) = � Eri ( i ) i=1 each atom contributes to Energy Compute Energy of Config. A • Energy of initial configuration E1 = 1� 10� 1+ 5 + 18+ 20= 20.95 E2 = 1� 10� 2+ 5 + 5 + 5 = 26.71 E3 = 1� 10� 4+ 18+ 5 + 2 = 47.89 E4 = 1� 10� 3+ 20+ 5 + 2 = 38.12 Total Energy Configuration A: E({rA})= 133.67 Boltzmann Probability P! Number of configurations NR = ( PN- )! P=# of slots=25 N =6,375,600 N=# of atoms =4 R What is the likelihood that a particular configuration will exist in a large ensemble of configurations? Ø-Er({ }) ø Boltzmann probability Pr({ }) = exp Œ œ depends on energy º kTB ß and temperature Boltzmann Collapse at low T T=100 T=10 T=1 T=100 - E =100.1184 T=10 - E =58.9245 avg avg T=1 - E avg =38.1017 14000 14000 12000 12000 12000 10000 10000 10000 8000 8000 8000 6000 Occurences 6000 Occurences 6000 Occurences 4000 4000 4000 2000 2000 2000 0 0 20 40 60 80 100 120 140 160 180 200 0 0 20 40 60 80 100 120 140 160 180 200 0 Energy 0 20 40 60 80 100 120 140 160 180 200 Energy Energy T high T low Boltzmann Distribution collapses to the lowest energy state(s) in the limit of low temperature Basis of search by Simulated Annealing Partition Function Z • Ensemble of configurations can be described statistically • Partition function, Z, generates the ensemble average � -E � NR � -E � Z = Tr exp� i � = � exp � i � Ł kB Tł i=1 Ł kTB ł Initially (at T>>0) equal to the number of possible configurations Free Energy NR Average Energy of all Eav g = E()T= � ET i ( ) Configurations in an i=1 ensemble F( T) =- kB TZln= E ()T- TS NR � ETi ( ) � �exp � - � ETi ( ) i=1 Ł kTB ł -dZln E avg = ET() = = Z d (1 kTB ) Relates average Energy at T with Entropy S Specific Heat and Entropy • Specific Heat NR Ø 1 2 2 ø Œ � Ei () T- ET ( ) œ 2 2 dET() º N R i=1 ß E() T- ET ( ) CT( ) = = 2 = 2 dT kBT kTB • Entropy T 1 CT() dS(T)CT () S(T)= S ()T- dT 1 � = T T dTT Entropy is ~ equal to ln(# of unique configurations) Low Temperature Statistics Specific Heat C and Entropy S 5 10 10 T 8 Entropy S s, N 6 decreases 4 with lower T onfiguration f c 2 # o 0 # configs 10 0 Ht. Capacity 0 2 4 0 2 4 10 10 10 10 10 10 decreases Temperature Temperature 150 150 100 g 100 v a 50 y, E 50 y, F 0 0 Energ -50 -50 Free Energ erage -100 -100 Approaches Approaches Av -150 -150 E* from E* from 0 2 4 0 2 4 10 10 10 10 10 10 below Temperature Temperature above Minimum Energy Configurations • Sample Atom Placement Problem Uniqueness? Atom Configuration - Sample Problem Atom Configuration - Sample Problem 7 7 6 6 5 E*=60 5 E*=14.26 4 4 3 3 2 2 3 2 1 2 1 4 3 1 4 1 0 0 -1 -1 -1 0 1 2 3 4 5 6 7 -1 0 1 2 3 4 5 6 7 Strong gravity g=10 Low gravity g=0.1 Simulated Annealing Dilemma • Cannot compute energy of all configurations ! – Design space often too large – Computation time for a single function evaluation can be large • Use Metropolis Algorithm, at successively lower temperatures to find low energy states – Metropolis: Simulate behavior of a set of atoms in thermal equilibrium (1953) – Probability of a configuration existing at T � Boltzmann Probability P(r,T)=exp(-E(r)/T) The SA Algorithm • Terminology: – X (or R or G) = Design Vector (i.e. Design, Architecture, Configuration) – E = System Energy (i.e. Objective Function Value) – T = System Temperature – D = Difference in System Energy Between Two Design Vectors • The Simulated Annealing Algorithm 1) Choose a random Xi, select the initial system temperature, and specify the cooling (i.e. annealing) schedule 2) Evaluate E(Xi) using a simulation model 3) Perturb Xi to obtain a neighboring Design Vector (Xi+1) 4) Evaluate E(Xi+1) using a simulation model 5) If E(Xi+1)< E(Xi), Xi+1 is the new current solution 6) If E(Xi+1)> E(Xi), then accept Xi+1 as the new current solution with a (- D/T) probability e where D = E(Xi+1) - E(Xi). 7) Reduce the system temperature according to the cooling schedule. 8) Terminate the algorithm. SA BLOCK DIAGRAM Start To End Tmin � � � y Define Initial n T < Tmin ? Configuration Ro � Perturb Configuration Reduce Temperature Evaluate Energy E(Ro) � Ri -> Ri+1 � Tj+1 = Tj - �T �� �� n y � Evaluate Energy E(Ri+1) Reached Equilibrium at Tj ? � Compute Energy Difference Accept R as i+1 Keep Ri as Current �E = E(Ri+1) - E(Ri) � New Configuration Configuration � y y n �E < 0 ? exp -�E > � ? � � Metropolis n Step � � Create Random Number � in [0,1] Matlab Function: SA.m Best Configuration Initial Configuration SA.m xbest Ebest xo Simulated Annealing Option Flags Algorithm History options xhist evaluation perturbation function function file_eval.m file_perturb.m Exponential Cooling k • Typically (T1/To)~0.7-0.9 � T � T = 1 T Simulated Annealing Evolution k +1 � � k 10 C-specific heat Ł To ł 9 S-entropy T/10-temperature 8 7 Can also do linear 6 or step-wise cooling 5 4 3 … 2 But exponential cooling 1 often works best. 0 0 5 10 15 20 25 30 35 40 45 Temperature Step Key Ingredients for SA • A concise description of a configuration (architecture, design, topology) of the system (Design Vector). • A random generator of rearrangements of the elements in a configuration (Neighborhoods).
Details
-
File Typepdf
-
Upload Time-
-
Content LanguagesEnglish
-
Upload UserAnonymous/Not logged-in
-
File Pages44 Page
-
File Size-