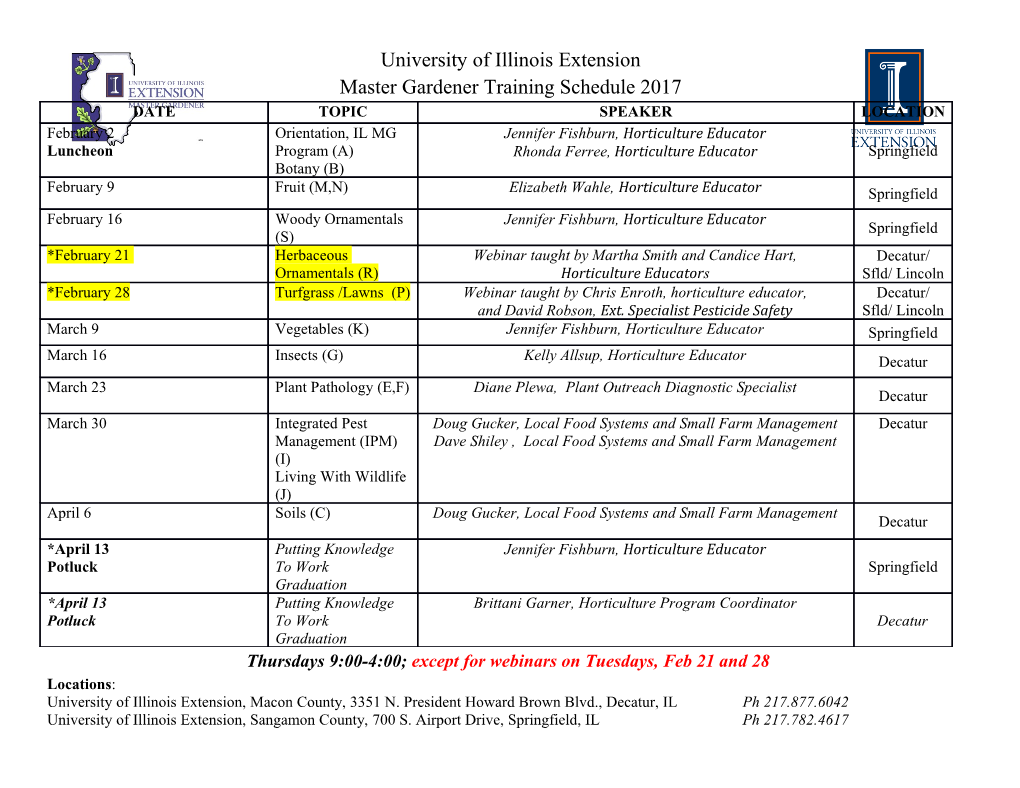
Advances in Computer Science Research (ACSR), volume 76 7th International Conference on Education, Management, Information and Mechanical Engineering (EMIM 2017) Lossless Compression Algorithm for Multi-source Sensor Data Research Changchi Huang1, a and Yuwen Chen1,b* 1Hainan University, Danzhou, Hainan, 571737, China [email protected], [email protected] Keywords: Multi-source sensor; DEFLATE algorithm; Sub-search; Compression algorithm Abstract. In recent years, multi-source sensor system has been widely used in military, agriculture, forestry and other fields, the diversity of information performance, the huge number of information, the complexity of information relations, and require information processing real-time, have been greatly Beyond the general computer integrated processing capacity. In this paper, studies have found that the DEFLATE algorithm is consistent with the "neighbor principle" of sensor data. This is a combination of LZ77 and Huffman algorithms, which in theory should deal with multi-source sensor data more effectively. This paper proposes to increase the "secondary search" function during the DEFLATE algorithm matching process. This improvement effectively increases the possibility of finding a longer matching string, thus further reducing the coding length. Introduction Research Background. With the development of microelectronics technology, integrated circuits and their design technology, computer technology, modern signal processing technology and sensor technology, a variety of multi-sensor systems for complex application background have emerged[1]. Multi-sensor data fusion, it is the 20th century, 80 years to form and develop an automated information processing technology, refers to the data from multiple sensors for multi-level, multi-level, multi-level processing, resulting in new and meaningful information, and this new information is not available to any single sensor. However, the technology in the development process is facing a huge problem such as the problem, which multi-sensor data transmission, save a great impact, so now based on such data compression algorithm demand is increasingly urgent[2]. Study the Significance of Data Compression. Data compression is widely used in various fields. Company data backup needs to compress a lot of data to reduce storage space. In order to take full advantage of the channel, the TV signal, streaming media and other information need to be compressed. Data compression is usually divided into lossless compression and lossy compression[3]. For those who do not pay attention to details of the data, such as images, video, most people use lossy compression technology, such as MPEG, H.263, H.264 and other popular compression technology. And for programs, electronic files and other similar important information, you must use lossless compression technology. So that data recovery will not undermine its integrity. The research on lossless compression technology has been a long time, and the most important lossless compression technology is the LZ series compression algorithm and the minimum redundancy construction algorithm Huffman algorithm. In today's multi-sensor measurement system, in order to accurately measure some of the impact signal, the sensor output signal needs high-speed, high-resolution sampling. This will inevitably produce a large number of data needs to be stored, take up a lot of storage space, but also not easy to real-time transmission. Therefore, in order to ensure the measurement accuracy, in the sampling rate and resolution can not be reduced, the sensor signal compression storage, transmission is very necessary. In this paper, Copyright © 2017, the Authors. Published by Atlantis Press. 1328 This is an open access article under the CC BY-NC license (http://creativecommons.org/licenses/by-nc/4.0/). Advances in Computer Science Research (ACSR), volume 76 LZ77 and Huffman combine a method to deal with multi-source sensor data, and achieved good results. The research of this topic will be beneficial to the further development of multi-source sensor system, effectively improve the efficiency of transmission and reduce the storage space used[4]. Development Status at Home and Abroad. Data compression technology is developed with the rapid development and extensive application of computer, digital communication, signal processing and other information technology, and has gradually developed into an independent discipline in recent years. The early stages of development from the end of the 18th century to the early 1950s were the embryonic period of data compression. Development period is the development of long-term, from the early 1950s to the 1970s, this period is the rapid development of theoretical basis period[5]. The theory of information created by the father of information c. E. Shannon laid the theoretical foundation for all data compression algorithms. The recent development of data compression began to become a theoretical and technically more systematic period of independent discipline, the statistical code has developed to a peak, most people along this idea to try to improve the algorithm, and always reach the ideal Effect. In recent years, more and more data compression software has been developed and used. Since the beginning of the 21st century, the rapid development of science and technology, related disciplines and the emergence of marginal disciplines, broaden the people's research ideas, to the development of data compression technology has injected new vitality. Therefore, compression technology based on multi-source sensor data will be developed rapidly and increasingly popular. Data Compression Theory Foundation Compression Technology. For compression technology, in fact refers to two algorithms[6]. One is the compression algorithm, which takes the input, generates a representation that requires fewer bits, and the other is the reconstruction algorithm, which performs the operation on the compressed representation to produce the reconstructed result. The schematic diagram of these operations is .shown in Fig. 1 This paper will follow the convention, the compression algorithm and reconstruction algorithm together, known as the compression algorithm. According to the requirements of reconstruction, data compression method can be divided into two categories: the first category is lossless compression, reconstruction results with the same; the second category is lossy compression, compression ratio of such compression is usually higher than lossless compression, But the reconstruction results may be different. Figure 2.1. Compression and reconstruction Introduction to Information Theory. Data compression and information theory are inseparable. In general, the establishment of the theoretical basis of information theory began in Shannon 1329 Advances in Computer Science Research (ACSR), volume 76 published in 1948, known as the theory of information for the mountains of "communication theory of mathematics." He used a professional term entropy, which was originally used in thermodynamics to represent the degree of disorder in the physical system. In essence, the purpose of data compression is to eliminate the redundancy in the information, the degree of information redundancy is precisely the information entropy and related theorem with mathematical means to accurately describe. The steps of data compression are generally divided into three parts: modeling expression, quadratic quantization and entropy coding[7]. Firstly, a certain model is established, the original data is transformed into a model reference, and then the model reference is transformed into a quantization symbol by quadratic quantization. Finally, the quadratic quantized symbol is transformed into a compressed stream by entropy coding The The general steps for data compression are shown in Fig. 2. Figure 2. General steps of data compression Let a discrete source be X, and the set A = {x1, x2, ⋯ , xn} represents a set of N symbols. In the source, the probability of occurrence of each symbol is determined, that is, there is a probability N distribution table {p1, p2, ⋯ , pn} and is satisfied ∑k=1 pk = 1. If the appearance of each symbol is irrelevant, or that independent, in the information theory, said the source X is no memory. Set the p(xi) probability that the X will issue the symbol xi at a certain moment, Shannon defines the amount of information for I(xi) as Eqs. (1). I(xi) = −logp(xi) (1) And i = 1,2, ⋯ , n。 The unit I(xi) is determined by the bottom of the logarithm. When 2 is the end, I(xi) = −log2p(xi), the amount of information in units of bits , when the natural number e for the end, the unit for the nat. As can be seen from the above equation, the information function is a decreasing function. Specifically, the less likely the occurrence of a random event, the more information it is, the greater the likelihood of occurrence, the less the amount of information[8]. When X represents all the possible symbol sets of the source output, the information quantity of each symbol xi of X is averaged by probability P(xi), and the average amount of information I(xi) of each symbol of X is Eqs. (2). N N H(X) = ∑i=1 logp(xi)I(xi) = − ∑i=1 p(xi)logp(xi) (2) Because this expression is the same as the entropy in thermodynamics, it is called the entropy of the source X in information theory. The principle of information theory shows that if we know the entropy of a memoryless source X, we can always find a compression method for the information source so
Details
-
File Typepdf
-
Upload Time-
-
Content LanguagesEnglish
-
Upload UserAnonymous/Not logged-in
-
File Pages8 Page
-
File Size-